Scientists built a machine learning model that can rapidly predict how atoms absorb x-rays for materials science research.
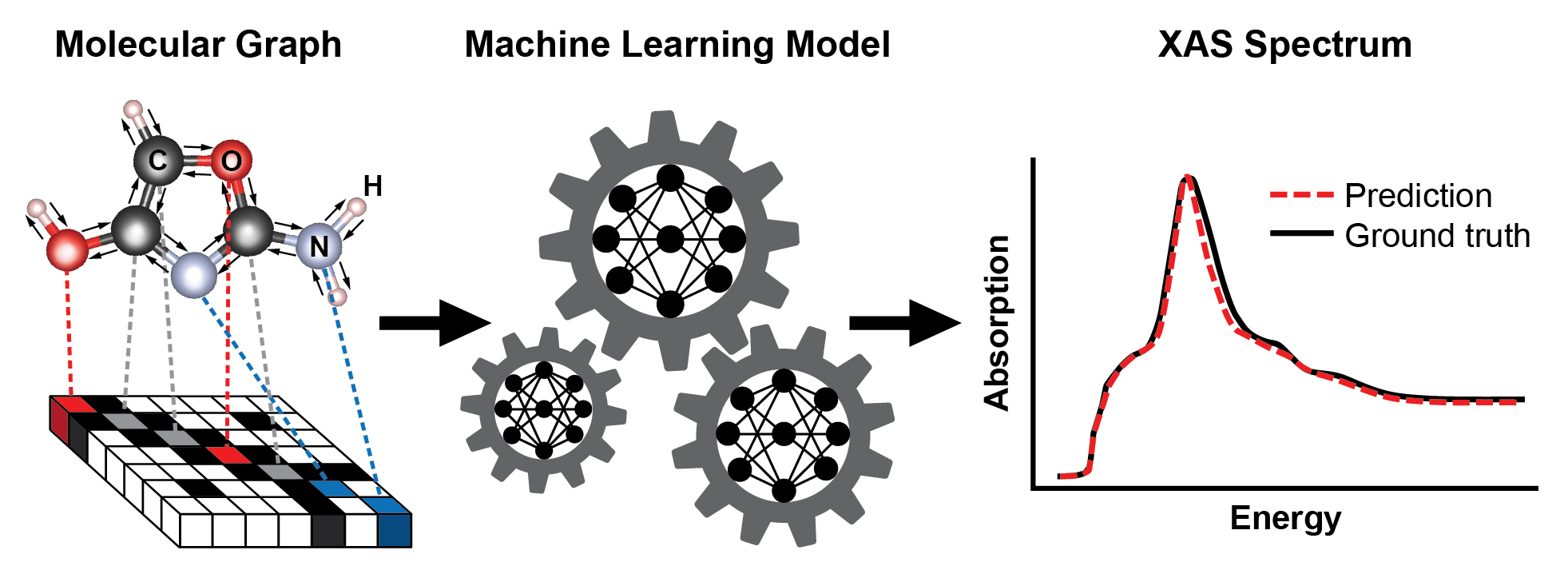
news, journals and articles from all over the world.
Scientists built a machine learning model that can rapidly predict how atoms absorb x-rays for materials science research.
Researchers at the University of New Hampshire used SDSC’s Comet supercomputer to validate a model using a machine learning technique called Dynamic Time Lag Regression (DTLR) to help predict the solar wind arrival near the Earth’s orbit from physical parameters of the Sun.
Research from the McKelvey School of Engineering at Washington University in St. Louis has shown that understanding brain activity as a network instead of readings from an EEG allow for more accurate and efficient detection of seizures in real-time.
Researchers at Binghamton University, State University of New York are using machine learning to track the coronavirus and predict where it might surge next.
NIBIB-funded researchers at Stanford University have created an artificial neural network that analyzes lung CT scans to provide information about lung cancer severity that can guide treatment options.
Argonne scientists Michael Bishof, Maria Chan, Marco Govini, Alessandro Lovato, Bogdan Nicolae and Stefan Wild have received funding for their research as part of DOE’s Early Career Research Program.
Potential biases that limit the effectiveness of machine learning process technologies and the scope for human capital to be complementary in reducing such biases is explored by Rajshree Agarwal and Evan Starr at the University of Maryland’s Robert H. Smith…
At a glance:
• Scientists develop AI-based tool to predict adverse drug events
• Such events are responsible for some 2 million U.S. hospitalizations per year
• The free, open-source system could enable safer drug design, optimize drug safety
Understanding the source and network of signals as the brain functions is a central goal of brain research. Now, Carnegie Mellon engineers have created a system for high-density EEG imaging of the origin and path of normal and abnormal brain signals.
This new agreement will dramatically improve and reduce the computational expense of fluid dynamics models. Both partners aim to improve the design and durability of engine components.
ISPOR—The Professional Society for Health Economics and Outcomes Research (HEOR) announced that its HEOR Short Course Program is now being offered virtually with 9 upcoming short courses in June and July.
In order to identify materials that can improve storage technologies for fuel cells and batteries, you need to be able to visualize the actual three-dimensional structure of a particular material up close and in context. Researchers from the University of Delaware’s Catalysis Center for Energy Innovation (CCEI) have done just that, developing new techniques for characterizing complex materials.
Using advanced machine learning, drones could be used to detect dangerous “butterfly” landmines in remote regions of post-conflict countries, according to research from Binghamton University, State University at New York.
One of the many unanswered scientific questions about COVID-19 is whether it is seasonal like the flu – waning in warm summer months then resurging in the fall and winter. Now scientists at Lawrence Berkeley National Laboratory (Berkeley Lab) are launching a project to apply machine-learning methods to a plethora of health and environmental datasets, combined with high-resolution climate models and seasonal forecasts, to tease out the answer.
ISPOR held its second Virtual ISPOR 2020 plenary session this afternoon, “Health Economics and Outcomes Research and Clinical Decision Making—Advancing Meaningful Progress.”
Roboticists at the University of California San Diego have developed an affordable, easy to use system to track the location of flexible surgical robots inside the human body. The system performs as well as current state of the art methods, but is much less expensive.
Johns Hopkins researchers recently received a $195,000 Rapid Response Research grant from the National Science Foundation to, using machine learning, identify which COVID-19 patients are at risk of adverse cardiac events such as heart failure, sustained abnormal heartbeats, heart attacks, cardiogenic shock and death.
Researchers at Columbia Engineering and the University of South Carolina have developed a method that combines big data and machine learning to selectively design gas-filtering polymer membranes to reduce greenhouse gas emissions. Their study, published today in Science Advances, is the first to apply an experimentally validated machine learning method to rapidly design and develop advanced gas separation membranes.
Berkeley Lab’s Kristin Persson shares her thoughts on what inspired her to launch the Materials Project online database, the future of materials research and machine learning, and how she found her own way into a STEM career.
New machine learning methods bring insights into how lithium ion batteries degrade, and show it’s more complicated than many thought.
A group of Harrisburg University of Science and Technology students and professors have developed an automated computer classifier capable of predicting with 80% accuracy and no racial bias whether someone is likely to be a criminal based solely on a picture of their face.
Jonathan W. Korn, a PhD student in Harrisburg University of Science and Technology’s Data Science program and a NYPD veteran; Prof. Nathaniel J.S. Ashby, and Prof. Roozbeh Sadeghian’s research titled “A Deep Neural Network Model to Predict Criminality Using Image Processing” will appear in the forthcoming Springer Nature – Research Book Series: Transactions on Computational Science & Computational Intelligence
Identifying sources of light plays an important role in the development of many photonic technologies, such as lidar, remote sensing, and microscopy. Traditionally, identifying light sources as diverse as sunlight, laser radiation, or molecule fluorescence has required millions of measurements, particularly in low-light environments, which limits the realistic implementation of quantum photonic technologies. In Applied Physics Reviews, researchers demonstrated a smart quantum technology that enables a dramatic reduction in the number of measurements required to identify light sources
University of Alabama in Huntsville (UAH) professor of biological science Dr. Jerome Baudry is collaborating with Hewlett Packard Enterprise (HPE) to use HPE’s Cray Sentinel supercomputer to search for natural products that are effective against the COVID-19 virus.
Lawrence Livermore National Laboratory researchers have identified an initial set of therapeutic antibody sequences, designed in a few weeks using machine learning and supercomputing, aimed at binding and neutralizing SARS-CoV-2, the virus that causes COVID-19. The research team is performing experimental testing on the chosen antibody designs.
Irvine, Calif., April 29, 2020 — Researchers at the University of California, Irvine have developed a new mathematical machine-intelligence-based technique that spatially delineates highly complicated cell-to-cell and gene-gene interactions. The powerful method could help with the diagnosis and treatment of diseases ranging from cancer to COVID-19 through quantifing crosstalks between “good” cells and “bad” cells.
SLAC researchers have developed a new tool, using machine learning, that may make part of the accelerator tuning process five times faster compared to previous methods.
A team of materials scientists at Lawrence Berkeley National Laboratory – scientists who normally spend their time researching things like high-performance materials for thermoelectrics or battery cathodes – have built a text-mining tool in record time to help the global scientific community synthesize the mountain of scientific literature on COVID-19 being generated every day.
Medical physicists at the Mayo Clinic have just made a unique library of computed tomography (CT) data publicly available so that imaging researchers can study, develop, validate, and optimize algorithms and enhance imaging hardware to produce peak-quality CT images using low radiation doses.
“There are no simple, robust, general tools that, for example, officials in Albany could use to make projections,” said Magdon-Ismail, a professor of computer science, and expert in machine learning, data mining, and pattern recognition. “These models show that the projections vary enormously from one city to another. This knowledge could relieve some of the uncertainty that is around in developing policy.”
The lab is responding to the coronavirus crisis by imaging disease-related biomolecules, developing standards for reliable coronavirus testing and enabling other essential research.
The INCITE program is now seeking proposals for high-impact, computationally intensive research projects that require the power and scale of DOE’s leadership-class supercomputers.
Bioengineers have combined standard microscopy, infrared light, and artificial intelligence to assemble digital biopsies that identify important molecular characteristics of cancer biopsy samples.
Argonne researchers have invented a machine-learning based algorithm for quantitatively characterizing material microstructure in three dimensions and in real time. This algorithm applies to most structural materials of interest to industry.
Cornell researchers have developed an automated system that uses machine learning, data analysis and human feedback to automatically verify statistical claims about the new coronavirus.
Activity from phony Twitter accounts established by the Russian Internet Research Agency between 2015 and 2017 may have contributed to politicizing Americans’ position on the nature and efficacy of vaccines, a health care topic which has not historically fallen along party lines, according to new research published in the American Journal of Public Health.
Argonne scientists are working around the clock to analyze the virus to find new treatments and cures, predict how it will propagate through the population, and make sure that our supply chains remain intact.
Argonne scientists and engineers are looking toward AI — specifically, machine learning — to help us better understand the mechanics that govern nuclear reactors.
Researchers have used artificial intelligence to detect Vietnam War-era bomb craters in Cambodia from satellite images – with the hope that it can help find unexploded bombs.
In a machine learning challenge dubbed the 2020 Large Hadron Collider Olympics, a team of cosmologists from Berkeley Lab developed a code that best identified a mock signal hidden in simulated particle-collision data.
Proteins are the building blocks of life and scientists have long studied how to improve them or design new ones. Traditionally, new proteins are created by mimicking existing proteins or manually editing their amino acids. This process is time-consuming, and it is difficult to predict the impact of changing an amino acid. In APL Bioengineering, researchers explore how to create new proteins by using machine learning to translate protein structures into musical scores, presenting an unusual way to translate physics concepts across domains.
Recent advances in unmanned‐aerial‐vehicle‐ (UAV‐) based remote sensing utilizing lightweight multispectral and thermal infrared sensors allow for rapid wide‐area landmine contamination detection and mapping surveys. We present results of a study focused on developing and testing an automated technique of…
In a study, the researchers used a machine learning algorithm to classify more than 110 types of plastics, including commercial and lab-made varieties, to better understand how they might degrade in the ocean.
Astrophysicists supported by the Department of Energy’s Office of Science are developing these guides in the form of computer models that rely on machine learning to examine the LSST data.
Applying deep learning to seismic data has revealed tremor and slip occur at all times—before and after known large-scale slow-slip earthquakes—rather than intermittently in discrete bursts, as previously believed.
Scientists may be a step closer to solving some of anthropology’s biggest mysteries thanks to a machine learning algorithm that can scour through remote sensing data, such as satellite imagery, looking for signs of human settlements, according to an international team of researchers.
Machine learning is helping Penn Medicine researchers identify the size and shape of brain networks in individual children, which may be useful for understanding psychiatric disorders. In a new study published in Neuron, a multidisciplinary team showed how brain networks unique to each child can predict cognition. The study is the first to show that functional neuroanatomy can vary greatly among kids, and is refined during development.
Argonne scientists are combining one-of-a-kind x-ray experiments with novel computer simulations to help engineers at aerospace and defense companies save time and money.
In the future, robots could take blood samples, benefiting patients and healthcare workers alike. A Rutgers-led team has created a blood-sampling robot that performed as well or better than people, according to the first human clinical trial of an automated blood drawing and testing device.
Bias in artificial intelligence is well established. Researchers are now proposing that developers incorporate the concept of “feminist design thinking” into their process as a way of improving equity – particularly in the development of software used in hiring.
As a staff member in the Theory and Computation Group at Brookhaven Lab’s Center for Functional Nanomaterials, Qu applies various approaches in artificial intelligence to analyze experimental and computational nanoscience data.