A research team created seven learning models using Support Vector Machine (SVM) algorithms to discern flowering-time-associated genes (FTAGs) from non-FTAGs, with the SVM-Kmer-PC-PseAAC model performing the best (F1 score = 0.934, accuracy = 0.939, and receiver operating characterstic = 0.943).
Tag: machine learning models
‘Molecular Compass’ points way to Reduction of Animal Testing
In recent years, machine learning models have become increasingly popular for risk assessment of chemical compounds. However, they are often considered ‘black boxes’ due to their lack of transparency, leading to scepticism among toxicologists and regulatory authorities. To increase confidence in these models, researchers at the University of Vienna proposed to carefully identify the areas of chemical space where these models are weak. They developed an innovative software tool (‘MolCompass’) for this purpose and the results of this research approach have just been published in the prestigious Journal of Cheminformatics.
Researchers Design Multiclass Cancer Diagnostic Tool Using AI, MicroRNA
MicroRNAs, or miRNAs, regulate genes and biological processes in the human body, including cancer formation and development. To explore the feasibility of miRNAs as cancer biomarkers, researchers created a multiclass cancer diagnostic model using miRNA expression profiles. The study examined the relationship between the composition of miRNAs and various types of cancers. Findings suggest that miRNAs may be highly unique to specific cancerous tissues and can be strong biomarkers for detection and classification in both research and the clinical field
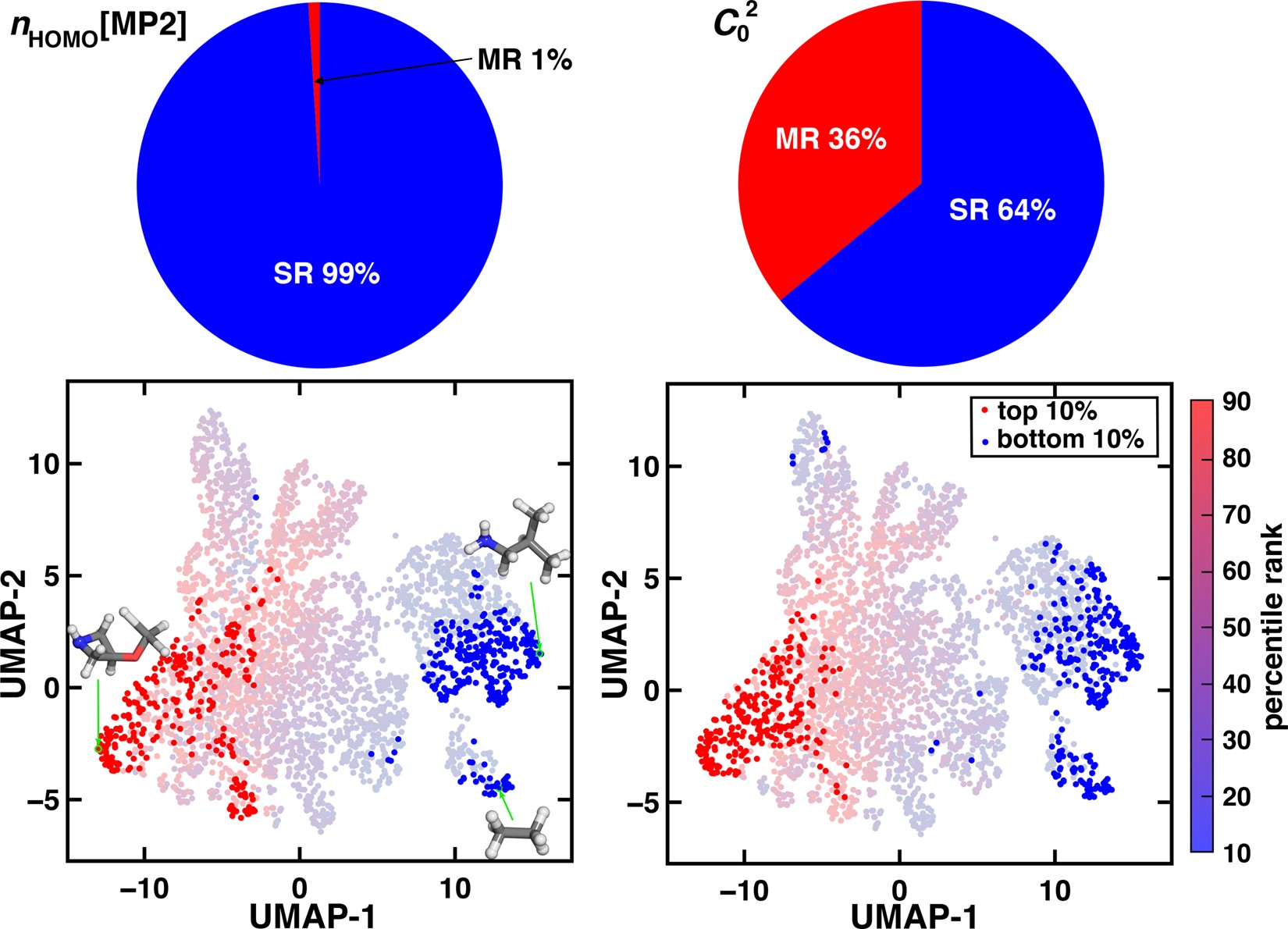
Supercomputers Help Advance Computational Chemistry
Researchers at the Massachusetts Institute of Technology (MIT) have succeeded in developing an artificial intelligence (AI) approach to detect electron correlation – the interaction between a system’s electrons – which is vital but expensive to calculate in quantum chemistry.
Mount Sinai Researchers Build Models Using Machine Learning Technique to Enhance Predictions of COVID-19 Outcomes
Mount Sinai researchers have published one of the first studies using federated learning to examine electronic health records to better predict how COVID-19 patients will progress.