As the 2024 election cycle approaches, concerns about cybersecurity threats to election integrity are mounting. Professor Justin DelVecchio, PhD, a cybersecurity expert from Canisius University, is available to provide insights on emerging threats that could potentially jeopardize the upcoming elections. …
Tag: AI algorithm
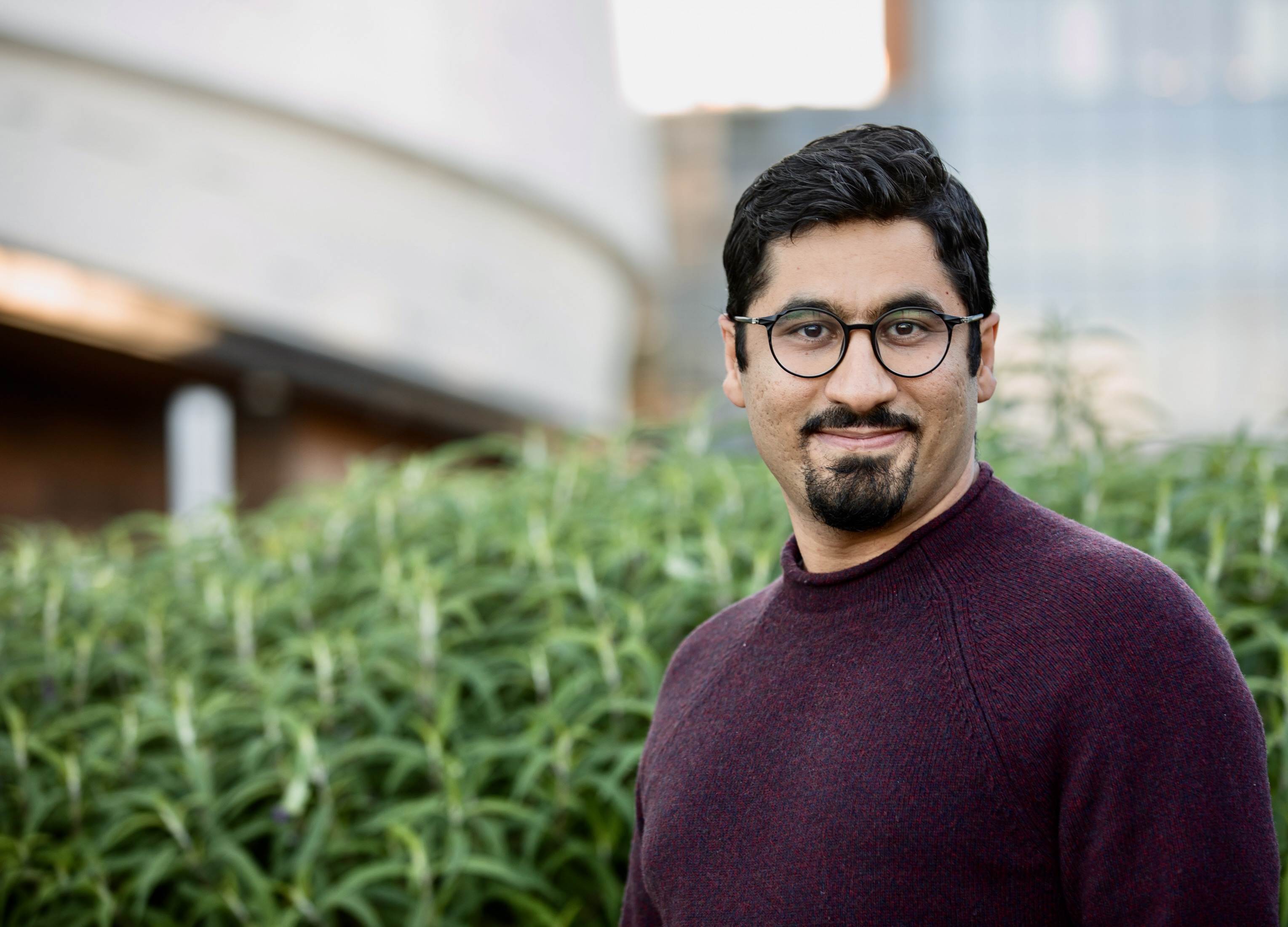
Enhancing MRI with AI to Improve Diagnosis of Brain Disorders
Researchers from UC San Francisco have developed a machine learning algorithm to enhance 3T MRIs by synthesizing 7T-like images that approximate real 7T MRIs. Their model enhanced pathological tissue with more fidelity for clinical insights and represents a new step toward evaluating clinical applications of synthetic 7T MRI models.
New AI Tool Finds Rare Variants Linked to Heart Disease in 17 Genes
Using an advanced artificial intelligence tool, researchers at the Icahn School of Medicine at Mount Sinai have identified rare coding variants in 17 genes that shed light on the molecular basis of coronary artery disease (CAD), the leading cause of morbidity and mortality worldwide. The discoveries, detailed in the June 11 online issue of Nature Genetics, reveal genetic factors impacting heart disease that open new avenues for targeted treatments and personalized approaches to cardiovascular care. The investigators used an in silico, or computer-derived, score for coronary artery disease (ISCAD) that holistically represents CAD, as described in a previous paper by the team in The Lancet. The ISCAD score incorporates hundreds of different clinical features from the electronic health record, including vital signs, laboratory test results, medications, symptoms, and diagnoses. To build the score, they trained machine learning models on the electronic health records of 604,914 individuals
Researchers Develop AI Model to Better Predict Which Drugs May Cause Birth Defects
Data scientists at the Icahn School of Medicine at Mount Sinai in New York and colleagues have created an artificial intelligence model that may more accurately predict which existing medicines, not currently classified as harmful, may in fact lead to congenital disabilities. The model, or “knowledge graph,” described in the July 17 issue of the Nature journal Communications Medicine, also has the potential to predict the involvement of pre-clinical compounds that may harm the developing fetus. The study is the first known of its kind to use knowledge graphs to integrate various data types to investigate the causes of congenital disabilities.
Automated epilepsy lesion detection on MRI: The MELD Project
In this episode of Sharp Waves, the ILAE podcast, Dr. Maryam Nabavi Nouri talks with Dr. Konrad Wagstyl about the MELD Project, an open-science consortium using deep learning principles to develop automated lesion detection of clinical MRI data.
AI-guided screening uses ECG data to detect a hidden risk factor for stroke
An AI-guided targeted screening strategy is effective in detecting new cases of atrial fibrillation that would not have come to attention in routine clinical care.
This strategy could reduce the number of undiagnosed cases of atrial fibrillation, and prevent stroke and death in millions of patients across the globe.

Study confirms the sensitivity of Techcyte’s AI solution for intestinal protozoa detection
A study authored by researchers at Quest Diagnostics and presented at ASM Microbe on June 9-12th 2022 in Washington, D.C. provides evidence that the Techcyte technology aids in the detection of intestinal protozoa.