Keith A. Brown
BS Physics, Massachusetts Institue of Technology
PhD Applied Physics, Harvard University
Postdoc in Chemistry, Northwestern University
Keith currently runs the KABlab, a research group at Boston University that studies approaches to accelerate the development of advanced materials and structures with a focus on polymers and smart fluids. The group employs self-driving labs, miniaturization of experiments using scanning probe techniques, and novel platforms for parallel materials development, and machine learning to achieve these goals. Keith has co-authored over 90 peer-reviewed publications, six issued patents, and his work has been recognized through awards including the Frontiers of Materials Award from The Minerals, Metals, & Materials Society (TMS), being recognized as a “Future Star of the AVS,” the Omar Farha Award for Research Leadership from Northwestern University, and the AVS Nanometer-Scale Science and Technology Division Postdoctoral Award. Keith served on the Nano Letters Early Career Advisory Board and currently leads the MRS Artificial Intelligence in Materials Development Staging Task Force.
- Review Article
- Published:
Role of AI in experimental materials science
Abstract
Over the past five years, artificial intelligence (AI) has grown significantly in different aspects of our daily lives, including health, transportation, and the digital world, all by leveraging data. Inspired by these success stories, materials researchers have started to adopt AI in experimental materials science to accelerate materials discovery and development by 10–100× through improving the efficiency of hypothesis generation, testing, and data analysis in a closed-loop fashion. This issue of MRS Bulletin presents a collection of papers discussing the recent advancements of AI in different aspects of experimental materials science and provides a framework for the next generation of autonomous experimentation strategies. In this article, we review the role of AI in experimental materials science and summarize the key aspects and challenges of autonomous experimentation discussed in each contributed article. We pose four questions at the interface of AI and experimental materials science, and present immediate calls for action for researchers working in this emerging field to move beyond optimization toward autonomous discovery. We hope this issue can accelerate convergence as well as flexibility and reconfiguration of hardware and software modules of autonomous robotic experimentation techniques to enable true digitalization of materials synthesis.
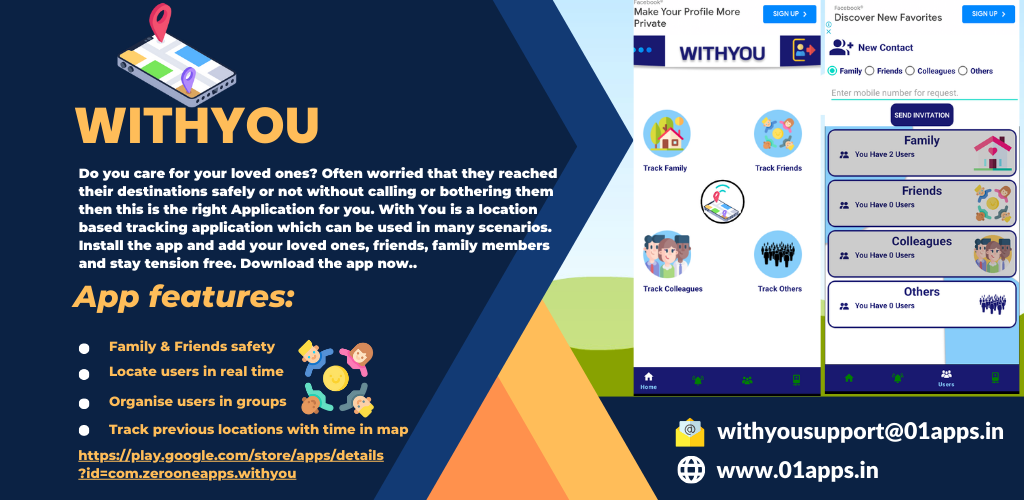