EMBARGOED UNTIL 2 A.M. PACIFIC JUNE 20, 2024
How Your Sleep Patterns Change Can Tell You About Your Health
Researchers identified five sleep types; the way people move between these types yields insights about both chronic and acute conditions
This is one of the findings of a study that analyzed data from 5 million nights of sleep across roughly 33,000 people. Based on the new analyses, the researchers identified five main types of sleep, which they called sleep phenotypes, and which can be further divided into 13 subtypes.
The researchers also found that how and how often a person switches between sleep phenotypes could offer two to ten times more information relevant to detecting health conditions compared with just relying on a person’s average sleep phenotype alone.
The study appears in the journal npj Digital Medicine on June 20, 2024.
Using data collected from Oura Ring–a smart ring that tracks sleep, skin temperature and other information–the researchers looked at individual people over a series of months, noting whether they had chronic health conditions such as diabetes and sleep apnea, or illnesses such as COVID-19 and the flu.
The research team found that people would often move between sleep phenotypes over time, reflecting a change in an individual’s health conditions, and creating what resembles a person’s travel log through the data-driven sleep landscape the researchers created.
“We found that little changes in sleep quality helped us identify health risks. Those little changes wouldn’t show up on an average night, or on a questionnaire, so it really shows how wearables help us detect risks that would otherwise be missed,” said Benjamin Smarr, one of the study’s senior authors and a faculty member in the Jacobs School of Engineering and Halicioglu Data Science Institute at the University of California San Diego.
In addition, the researchers highlighted that tracking changes in sleep over the long term at the population scale could unlock new insights that are relevant for public health, such as whether some changes in patterns through these sleep landscapes can provide early warning for chronic illness or vulnerability to infection.
The research team’s work is based on new analyses from the TemPredict dataset from University of California, San Francisco, which was created using data collected from people wearing the commercially available Oura Ring during the 2020 COVID-19 pandemic.
The analyses were led by Smarr, who is also faculty in the University of California San Diego Shu Chien – Gene Lay Department of Bioengineering, and Professor Edward Wang in the University of California San Diego Department of Electrical and Computer Engineering, collaborating with the study lead at University of California, San Francisco, Professor Ashley E. Mason, a practicing sleep clinician. The lead author was Varun Viswanath, a graduate student in the Department of Electrical and Computer Engineering at the University of California San Diego Jacobs School of Engineering.
The five sleep types
These are the five sleep phenotypes researchers singled out based on data from 5 million nights of sleep across roughly 33,000 people. While many factors went into the study, the researchers also identified some trends that help to intuitively separate the 5 sleep phenotypes.
- Phenotype 1: What we think of as “normal” sleep. In this phenotype, people get about eight hours of uninterrupted sleep for at least six days in a row. This is the type of sleep recommended by the National Institutes of Health and was the most common sleep type researchers found.
- Phenotype 2: People sleep continuously about half the nights, but they only sleep for short periods of time in bouts of less than three hours the other half.
- Phenotype 3: People sleep mostly continuously, but they experience interrupted sleep around one night each week. The interrupted night is characterized by one period of relatively long sleep of about five hours, and one period of short sleep of less than three hours.
- Phenotype 4: People again sleep mostly continuously. But they experience rare nights, in which long bouts of sleep are separated by a mid-sleep waking.
- Phenotype 5: People only sleep for very short periods of time every night. This phenotype was the rarest the researchers found, and represents extremely disrupted sleep.
Tracking changes in sleep type
To measure how sleep phenotypes changed over time, Viswanath constructed a spatial model of all 5 million nights, in which the phenotypes were represented as different islands, composed of mostly similar weeks of sleep. Different patterns emerged over time that allowed the researchers to model each individual’s routes between islands.
From there, what helped to distinguish people with chronic conditions, such as diabetes and sleep apnea, was not their average phenotype. Instead, it was how frequently they switched between islands in this sleep landscape. In this way, even if someone switched phenotypes only rarely, the fact that they did switch could still provide useful information about their health.
The data showed that it is rare for most people to go multiple months without a few nights of disrupted sleep. “We found that the little differences in how sleep disruptions occur can tell us a lot. Even if these instances are rare, their frequency is also telling. So it’s not just whether you sleep well or not – it’s the patterns of sleep over time where the key info hides,” said Wang, a coauthor and electrical and computer engineering faculty member at UC San Diego.
Conversely, people did not tend to remain in patterns defined by broken up sleep. But how often they visited specific disrupted-sleep patterns says a lot about how well they’re doing.
“If you imagine there’s a landscape of sleep types, then it’s less about where you tend to live on that landscape, and more about how often you leave that area,” said Viswanath, the paper’s corresponding author.
Previous research
In this new paper published June 20, the research team modified the technique used in previous research that had been the largest similar investigation of sleep to date, which had drawn approximately 103,000 nights of data from the UK biobank. That previous study looked at sleep timing and awakenings and many related features, and then constructed a “landscape” of where nights fell in relation to each other. But prior researchers did not do two key things: they could not look across time, as they had only two to three nights per person; and they could not tie the resulting patterns of sleep to health outcomes.
Other large-scale sleep analyses looked at high-level differences in simple sleep characteristics, like the total time people spent asleep.
In contrast, this new work is the first to show that researchers can quantify the changing dynamics of people’s sleep over time and use this quantification to give people better insights into their sleep health. The research also suggests that these changes in sleep may indicate a higher risk for a wide range of conditions.
Paper “Five million nights: temporal dynamics in human sleep phenotypes” in the journal npj Digital Medicine
Paper authors
Varun K. Viswanath, Wendy Hartogenesis, Stephan Dilchert, Leena Pandya, Frederick M. Hecht, Ashley E. Mason, Edward J. Wang, and Benjamin L. Smarr
Author affiliations
Department of Electrical and Computer Engineering, Jacobs School of Engineering, University of California, San Diego; Osher Center for Integrative Health, University of California, San Francisco; Zicklin School of Business, Baruch College, The City University of New York; Shu Chien—Gene Lay Department of Bioengineering, Jacobs School of Engineering, University of California, San Diego; Halıcıoğlu Data Science Institute, University of California, San Diego
Corresponding author: Varun K. Viswanath [email protected]
Funding Acknowledgments
This effort was funded under MTEC solicitation MTEC-20-12-Diagnostics-023 and the USAMRDC under the Department of Defense (#MTEC-20-12-COVID19-D.-023). The #StartSmall foundation (#7029991), and Oura Health Oy (#134650) also provided funding for this work. The views and conclusions contained herein are those of the authors and should not be interpreted as necessarily representing the official policies or endorsements, either expressed or implied, of the U.S. Government.
Conflict of interest statement
A.E.M. has received remuneration for consulting work from Oura Ring Inc. but declares no non-financial competing interests. B.L.S. has received remuneration for consulting work from, and has a financial interest in, Oura Ring Inc. but declares no other non-financial competing interests.
A.E.M., PhD, and B.L.S., PhD, are listed as co-inventors on patent applications as follows: 17/357,922, filed June 24, 2021, entitled “ILLNESS DETECTION BASED ON TEMPERATURE DATA,” status is pending; PCT/US21/39260, filed June 25, 2021, entitled “ILLNESS DETECTION BASED ON TEMPERATURE DATA,” status is expired; and 17/357,930, filed June 24, 2021, entitled “HEALTH MONITORING PLATFORM FOR ILLNESS DETECTION,” status is pending. These were all filed as of July 2021 by Oura Health Oy on behalf of UCSD. All applications cover the use of wearable device data to detect illness onset.
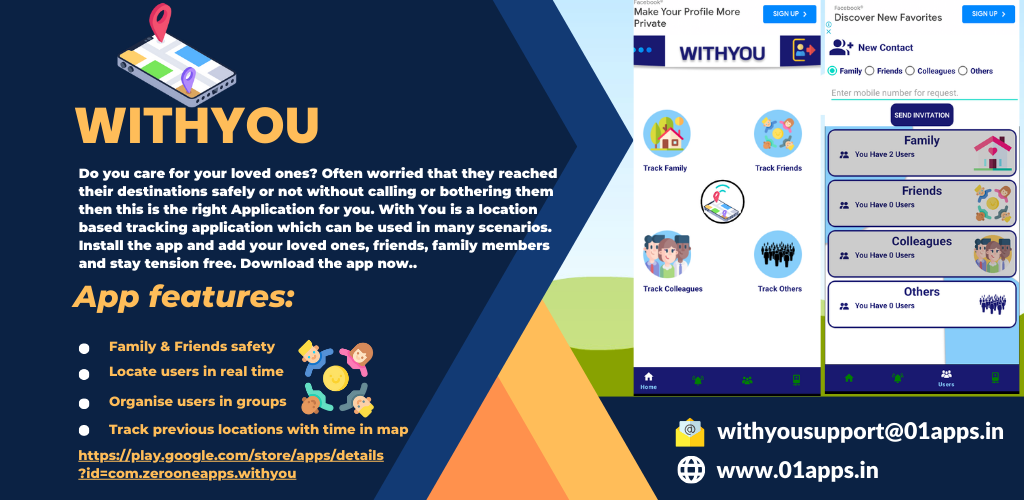