The Maria Goeppert Mayer Fellowship is an international award given to outstanding doctoral scientists and engineers to help them develop their careers in Argonne’s high-impact research environment. The fellowship honors Maria Goeppert Mayer, a theoretical physicist who earned the Nobel Prize in Physics in 1963 for her work at Argonne proposing a mathematical model for the structure of nuclear shells in the atomic nucleus. The fellowship provides early-career scientists the opportunity to pursue their own research directions, with the support of a sponsor and up to three years of funding. Here, Andrejevic discusses her interdisciplinary research interests and how the fellowship has advanced her career.
“What really stood out to me about this program was the unique opportunity to develop and carry out an independent research project and potentially see the impact firsthand, since Argonne is home to high performance computing systems in addition to state-of-the-art experimental facilities.” — Nina Andrejevic, Argonne scholar
Q: What is your research focus at Argonne?
A: Scientific advances in characterizing materials are enabling us to collect bigger and more detailed datasets. The issue we’re now facing is that the traditional methods used to interpret and analyze these experimental measurements can’t keep pace with the amount of data we’re generating. My research is centered on developing physics-aware machine learning models to accelerate the analysis of materials characterization data, including the data we obtain at DOE Office of Science user facilities like Argonne’s Advanced Photon Source (APS) and Center for Nanoscale Materials (CNM).
Q: What do you mean by physics aware?
A: Most of the well-known machine learning models, like ChatGPT, are trained on massive and highly diverse datasets. By contrast, scientific data represent a comparatively small subset of data that respect certain physical laws, suggesting that we need more specialized machine learning models. Physics aware means that we have incorporated scientific knowledge in the form of meaningful constraints on the model’s architecture in order to satisfy necessary physical laws. These constraints are important because, as scientists, we care about being able to interpret the models we develop and explain the predictions that we make.
Q: How do you develop machine learning models?
A: My research sits at the intersection of materials science, X-ray characterization and machine learning. While I primarily write and test code, I also draw upon knowledge from X-ray scattering theory and materials modeling to simulate training data, process experimental data and develop machine learning model architectures.
Q: What are some broader impacts of your work?
A: Materials characterization plays a huge role in investigating new materials for microelectronics, clean energy and quantum computing. The ability to analyze and make sense of data quickly and accurately is really important to accelerate discoveries about candidate materials and push forward next-generation technologies.
Q: What inspired you to apply for the Maria Goeppert Mayer Fellowship?
A: During my Ph.D. program at MIT, I came to Argonne several times as a user to perform experiments at the APS. However, I didn’t know about the fellowship or seriously consider working at Argonne until I contacted Dr. Maria Chan, who is my current co-supervisor alongside Dr. Mathew Cherukara. I emailed Dr. Chan out of the blue to learn about her research group and what it might be like to be a postdoc at Argonne. She invited me to collaborate on a side project while I completed my Ph.D., and I realized I would really enjoy continuing my research at Argonne. When Dr. Chan brought the fellowship to my attention, it sounded like the perfect opportunity.
Q: How has the fellowship contributed to your career development?
A: What really stood out to me about this program was the unique opportunity to develop and carry out an independent research project and potentially see the impact firsthand, since Argonne is home to high performance computing systems in addition to state-of-the-art experimental facilities. I hope that the computational tools I’m developing can be directly integrated into the experimental pipeline here.
Applying for the fellowship also helped me articulate what my own research direction would be beyond my Ph.D. I’m now gaining experience managing different aspects of a research project from the proposal phase to the process of carrying out, and hopefully deploying my research.
Q: What do you like about working at Argonne?
A: I really appreciate how collaborative the research environment is. I’ve been able to work with a very diverse group of scientists across the APS and CNM, which has helped me creatively bring together knowledge from different disciplines and learn to converse in multiple scientific languages. Apart from my Fellowship project, I’ve also been able to help develop proposals and get involved in other collaborative projects, which has connected me to the broader scope of research pursued at Argonne.
Q: What do you enjoy doing outside of work?
A: I really enjoy biking and dancing. The Postdoctoral Society of Argonne organizes a weekly social dance series, so I’ve been able to learn some new dance techniques and teach a few styles that I’ve learned in the past.
Q: What advice do you have for other scientists interested in your career path?
A: Don’t be afraid of entering a new field after your Ph.D. Actively seek out collaborators across disciplines, because the perspective and expertise you have in one area could be very valuable to another project or application.
Q: What did I miss that’s important to you?
A: A big part of my educational journey has been my twin sister. We’re very close and have followed similar academic and career tracks. When I was at MIT, she did her Ph.D. at Harvard, and we even had the opportunity to co-author a paper together. We’re both passionate about scientific data visualization and generative art, which has brought us together on several creative side projects as well.
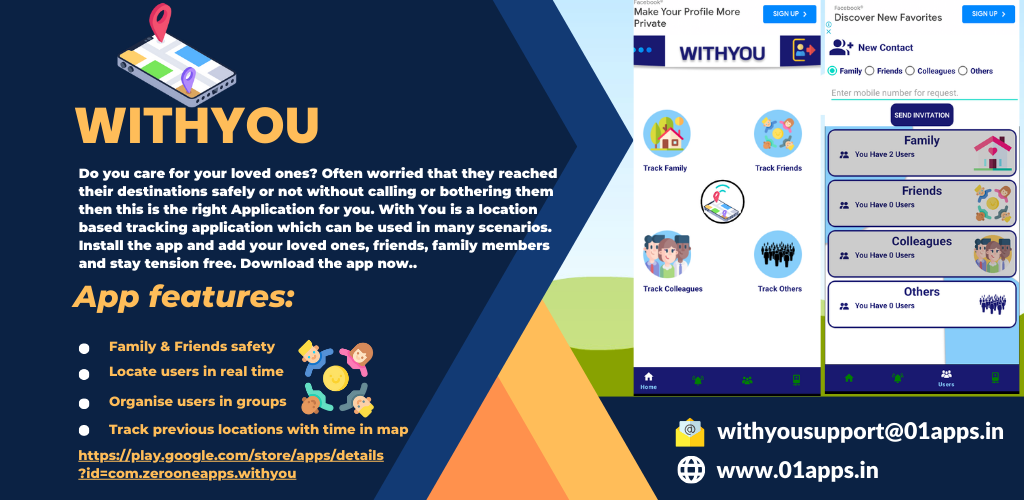