A field experiment investigating how the natural language model GPT-3, the predecessor to the most recently released model, might be used to generate constituent email messages showed that legislators were only slightly less likely to respond to AI-generated messages (15.4%) than human-generated (17.3%).
The 2% difference, gleaned from more than 32,000 messages sent to about 7,000 state legislators in the U.S., was statistically significant but substantially small, the researchers said.
The study, “The Potential Impact of Emerging Technologies on Democratic Representation: Evidence from a Field Experiment,” co-authored by Sarah Kreps and Douglas Kriner, professors of government, published in the journal New Media and Society.
In recent years, new communication technologies have interfered with democratic process multiple times, Kreps said. In the 2016 U.S. presidential election, Russian agents used micro-targeted social media advertisements to manipulate American voters and influence the outcome. And in 2017, the Federal Communications Commission public comment lines were flooded with millions of messages generated by natural language models in response to a proposed rollback of regulations.
With these in mind, Kreps – who was an early academic collaborator of OpenAI – wondered what malicious actors could do with more powerful language models now widely available.
“Could they generate misinformation or politically motivated, targeted content at scale?” she asked. “Could they effectively distort the democratic process? Or might they be able to generate large volumes of emails that seem like they’re coming from constituents and thereby shift the legislative agenda toward the interests of a foreign government?”
In their experiment, conducted throughout 2020, Kreps and Kriner chose six current issues: reproductive rights, policing, tax levels, gun control, health policy and education policy.
Messages on gun control and health policy received virtually identical response rates, and on education policy, the response rate was higher for AI-generated messages, suggesting that “on these issues GPT-3 succeeded in producing content that was almost indistinguishable in the eyes of state legislative offices from human content,” they wrote.
In feedback after the experiment, state legislators shared how they pick out fake emails, such as lack of geographical markers. Some said they represent districts so small they can spot fakes simply by looking at a name.
However, such local clues to authenticity would be more difficult for national-level senators and representatives to spot, the researchers said.
Technological tools employing the same type of neural networks can help differentiate real messages from fake, “but so can a discerning eye and digital literacy,” Kreps said. “Legislators need to be trained to know what to look for.”
The research was funded in part by a A&S New Frontier Grant.
For additional information, see this Cornell Chronicle story.
Cornell University has dedicated television and audio studios available for media interviews.
-30-
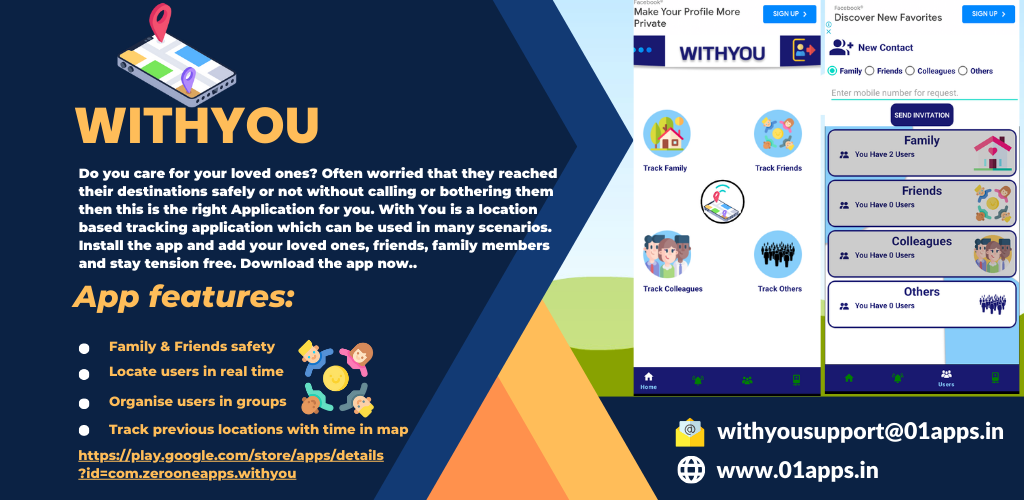