To advance the use of trustworthy and responsible AI for science, the Argonne Leadership Computing Facility (ALCF) is providing researchers with access to a growing collection of cutting-edge AI machines, dubbed the ALCF AI Testbed. The ALCF is a DOE Office of Science user facility at Argonne.
“I was drawn to the series because it is unique in that it offered a broad overview of the AI landscape, but also invaluable practical experience in training and using AI models.” — John Wu, Blinn College
“With powerful capabilities for AI workloads, our AI Testbed systems are opening doors to new and innovative research campaigns at the intersection of AI and science,” said Michael Papka, director of the ALCF. “As the volume of data produced by simulations, telescopes, light sources and other research facilities continues to skyrocket, it’s imperative that we explore how emerging AI technologies can support and accelerate data-intensive science.”
Argonne has partnered with multiple AI start-up companies to acquire and deploy a diverse set of AI systems, known as AI accelerators, to assemble the testbed. It is currently comprised of accelerators from Cerebras, Graphcore, Groq, Intel Habana and SambaNova.
“These AI accelerators were largely designed for enterprise workloads, such as e-commerce, but our goal is to understand how these novel systems can enhance scientific research,” said Venkat Vishwanath, AI and machine learning team lead at the ALCF. “Researchers using the testbed systems are achieving promising results across a wide range of research areas, including drug discovery, experimental data analysis and battery simulations. The accelerators are also supporting efforts to build safe and trustworthy AI capabilities for the nation.”
Researchers can request access to the systems by submitting a project proposal to the ALCF. The testbed systems are also available through the National Artificial Intelligence Research Resource (NAIRR) Pilot.
The testbed’s AI accelerators are equipped with unique hardware and software features to efficiently handle a variety of AI tasks, including:
- AI Model Training: Using large datasets to “teach” an AI model to detect patterns and make accurate, trustworthy predictions.
- Inference: Employing a trained AI model to make predictions on new data.
- Large Language Models (LLMs): AI models that are trained on large amounts of text data to understand, generate and predict text-based content.
- Computer Vision Models: AI models that are trained to understand and analyze visual data for tasks such as image classification and object recognition.
- Foundation Models: Similar to LLMs, these AI models are trained on diverse datasets to perform a broad set of processing tasks. Foundation models, however, can serve as a starting point for developing more specialized AI models for specific domains or applications.
Many of these AI techniques are now commonplace in everyday life. For example, computer vision models power the facial recognition software on our smartphones, while LLMs enable ChatGPT and other chatbots to generate human-like text responses.
These methods have also emerged as powerful tools for speeding up scientific progress. Computer vision models can help scientists automate the analysis of images generated by microscopes, X-ray light sources and other imaging techniques. LLMs, on the other hand, are helping researchers to sift through massive amounts of published scientific data quickly to identify promising materials for medicines, solar cells and other uses.
As part of a study that leveraged the ALCF AI Testbed, an Argonne-led team trained LLMs with genomic data to create the first genome-scale language models. Known as GenSLMs, the team’s models were built to study the evolution of SARS-CoV-2 (the virus that causes COVID-19). Their research demonstrated how LLMs can help scientists identify and classify new variants of SARS-CoV-2 and other viruses. The team’s work was recognized with the prestigious Gordon Bell Special Prize for High Performance Computing-Based COVID-19 Research in 2022
Experimental data analysis is another area getting a boost from the lab’s AI Testbed. Researchers from the Advanced Photon Source (APS), a DOE Office of Science user facility at Argonne, are exploring how different accelerators can enable fast, scalable AI model training and inference to accelerate the analysis of X-ray imaging data. Rapid data analysis methods are becoming increasingly important for the APS and other experimental facilities as data generation rates continue to grow.
“AI is not just a helpful tool for us; it is expected to become a necessity for fully exploiting the experimental capabilities of the upgraded APS,” said Mathew Cherukara, computational scientist and group leader at the APS. “The ALCF AI Testbed is giving us some new avenues to test and develop methods for processing and analyzing the large and varied data streams generated by our user community.”
To help researchers get started with the testbed, the ALCF team is partnering with the AI companies to host training events focused on their respective systems. This year, the ALCF is hosting a series of two-day workshops to introduce system hardware and software, cover best practices and provide hands-on guidance using the machines. The ALCF team has also organized tutorials on programming the AI accelerators for science at the annual Supercomputing conference for the past two years.
Accelerating discoveries and advancing the use of trustworthy AI for scientific research are not the only goals of the ALCF AI Testbed. Argonne is also using the testbed to explore how AI systems can play a role in boosting the capabilities and efficiency of next-generation computing facilities.
“Another goal of the AI Testbed is to determine out how AI accelerators could be integrated with next-generation supercomputers,” Vishwanath said. “Beyond speeding up time to solution, accelerators offer advantages in energy efficiency, which is an important factor as we architect larger and larger systems in the future.”
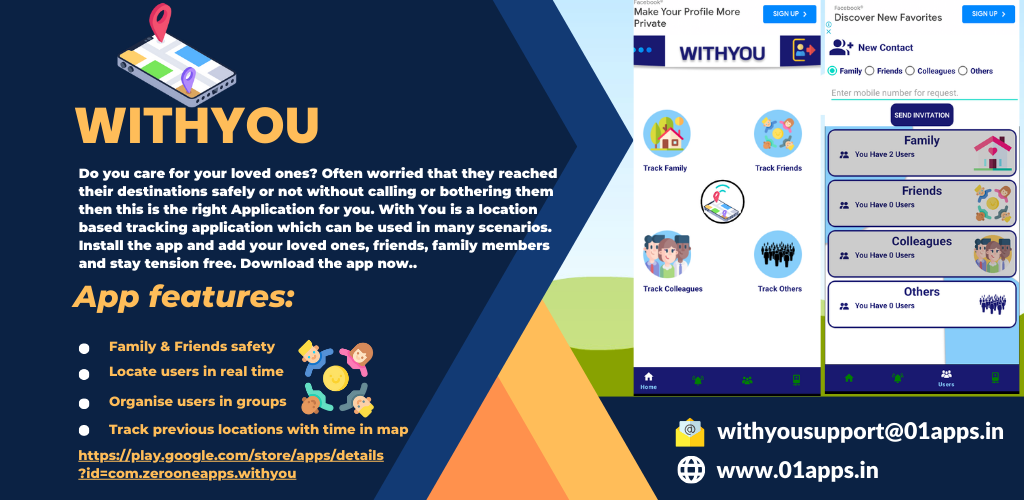