The spread of hate speech via social media could be tackled using the same “quarantine” approach deployed to combat malicious software, according to University of Cambridge researchers.
Definitions of hate speech vary depending on nation, law and platform, and just blocking keywords is ineffectual: graphic descriptions of violence need not contain obvious ethnic slurs to constitute racist death threats, for example.
As such, hate speech is difficult to detect automatically. It has to be reported by those exposed to it, after the intended “psychological harm” is inflicted, with armies of moderators required to judge every case.
This is the new front line of an ancient debate: freedom of speech versus poisonous language.
Now, an engineer and a linguist have published a proposal in the journal
Ethics and Information Technology
that harnesses cyber security techniques to give control to those targeted, without resorting to censorship.
Cambridge language and machine learning experts are using databases of threats and violent insults to build algorithms that can provide a score for the likelihood of an online message containing forms of hate speech.
As these algorithms get refined, potential hate speech could be identified and “quarantined”. Users would receive a warning alert with a “Hate O’Meter” – the hate speech severity score – the sender’s name, and an option to view the content or delete unseen.
This approach is akin to spam and malware filters, and researchers from the ‘Giving Voice to Digital Democracies’ project believe it could dramatically reduce the amount of hate speech people are forced to experience. They are aiming to have a prototype ready in early 2020.
“Hate speech is a form of intentional online harm, like malware, and can therefore be handled by means of quarantining,” said co-author and linguist Dr Stefanie Ullman. “In fact, a lot of hate speech is actually generated by software such as Twitter bots.”
“Companies like Facebook, Twitter and Google generally respond reactively to hate speech,” said co-author and engineer Dr Marcus Tomalin. “This may be okay for those who don’t encounter it often. For others it’s too little, too late.”
“Many women and people from minority groups in the public eye receive anonymous hate speech for daring to have an online presence. We are seeing this deter people from entering or continuing in public life, often those from groups in need of greater representation,” he said.
Former US Secretary of State Hillary Clinton
recently told a UK audience
that hate speech posed a “threat to democracies”, in the wake of many women MPs
citing online abuse
as part of the reason they will no longer stand for election.
While in a
Georgetown University address
, Facebook CEO Mark Zuckerberg spoke of “broad disagreements over what qualifies as hate” and argued: “we should err on the side of greater expression”.
The researchers say their proposal is not a magic bullet, but it does sit between the “extreme libertarian and authoritarian approaches” of either entirely permitting or prohibiting certain language online.
Importantly, the user becomes the arbiter. “Many people don’t like the idea of an unelected corporation or micromanaging government deciding what we can and can’t say to each other,” said Tomalin.
“Our system will flag when you should be careful, but it’s always your call. It doesn’t stop people posting or viewing what they like, but it gives much needed control to those being inundated with hate.”
In the paper, the researchers refer to detection algorithms achieving 60% accuracy – not much better than chance. Tomalin’s machine learning lab has now got this up to 80%, and he anticipates continued improvement of the mathematical modeling.
Meanwhile, Ullman gathers more “training data”: verified hate speech from which the algorithms can learn. This helps refine the “confidence scores” that determine a quarantine and subsequent Hate O’Meter read-out, which could be set like a sensitivity dial depending on user preference.
A basic example might involve a word like ‘bitch’: a misogynistic slur, but also a legitimate term in contexts such as dog breeding. It’s the algorithmic analysis of where such a word sits syntactically – the types of surrounding words and semantic relations between them – that informs the hate speech score.
“Identifying individual keywords isn’t enough, we are looking at entire sentence structures and far beyond. Sociolinguistic information in user profiles and posting histories can all help improve the classification process,” said Ullman.
Added Tomalin: “Through automated quarantines that provide guidance on the strength of hateful content, we can empower those at the receiving end of the hate speech poisoning our online discourses.”
However, the researchers, who work in Cambridge’s Centre for Research into Arts, Humanities and Social Sciences (CRASSH), say that – as with computer viruses – there will always be an arms race between hate speech and systems for limiting it.
The project has also begun to look at “counter-speech”: the ways people respond to hate speech. The researchers intend to feed into debates around how virtual assistants such as ‘Siri’ should respond to threats and intimidation.
###
The work has been funded by the International Foundation for the Humanities and Social Change.
This part of information is sourced from https://www.eurekalert.org/pub_releases/2019-12/uoc-ohs121719.php
Fred Lewsey
44-122-376-5566
[email protected]
http://www.cam.ac.uk
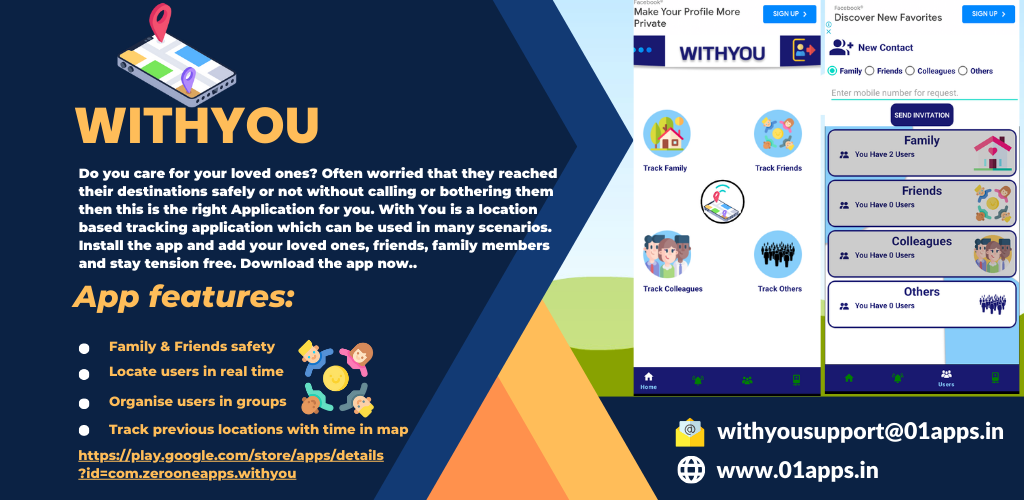