The study introduces a unique approach that combines machine learning algorithms, such as XGBoost and LightGBM, with ICESat-2 satellite data and multispectral imagery from Landsat-8 and Sentinel-2. The core innovation lies in the application of these advanced algorithms, which significantly improve depth estimation accuracy. For example, XGBoost achieved a root mean square error (RMSE) of just 0.54 meters when applied to Sentinel-2 L1C imagery, marking a substantial improvement over traditional methods like the radiative transfer equation (RTE) and spectral band ratio (SBR), which often struggle with accuracy, especially in deeper lakes.
The research team developed an enhanced Automated Lake Depth (ALD) algorithm to extract reliable lake depth sample points from ICESat-2 ATL03 data. These points were then paired with multispectral imagery to generate training data for machine learning models. Testing the method on seven supraglacial lakes in Greenland, the results showed that the machine learning algorithms, particularly when using Sentinel-2 L1C imagery, offered the most accurate depth estimates. The study also explored the impact of atmospheric corrections on depth retrieval, finding that top-of-atmosphere reflectance data performed better than atmospherically corrected data for mapping lake bathymetry. This suggests that current atmospheric correction methods could introduce errors, particularly over water and snow/ice surfaces.
Dr. Qi Liang, the lead researcher, highlighted the significance of the study: “Our machine learning-based approach not only enhances the accuracy of glacier lake depth estimation but also provides a scalable solution for large-area monitoring. This innovation offers the potential to advance our understanding of ice sheet dynamics and climate change impacts.”
By employing an improved ALD algorithm to process ICESat-2 ATL03 data and pairing it with multispectral imagery from Landsat-8 and Sentinel-2, the team created a powerful tool for glacier lake monitoring. This methodology was tested against reference depths from the ALD algorithm and validated using ArcticDEM data. The high accuracy and scalability of the machine learning-based approach open up new possibilities for large-scale monitoring in polar regions and other glaciated areas, crucial for assessing climate change impacts. The advancement holds great promise for refining sea-level rise predictions and enhancing global climate models.
###
References
DOI
Original Source URL
https://spj.science.org/doi/10.34133/remotesensing.0416
Funding information
This work was supported by the National Natural Science Foundation of China (grants 41925027 and 42306256), the Innovation Group Project of Southern Marine Science and Engineering Guangdong Laboratory (Zhuhai) (grant 311021008,) and the Guangdong Basic and Applied Basic Research Foundation (grant 2023A1515010925).
About Journal of Remote Sensing
The Journal of Remote Sensing, an online-only Open Access journal published in association with AIR-CAS, promotes the theory, science, and technology of remote sensing, as well as interdisciplinary research within earth and information science.
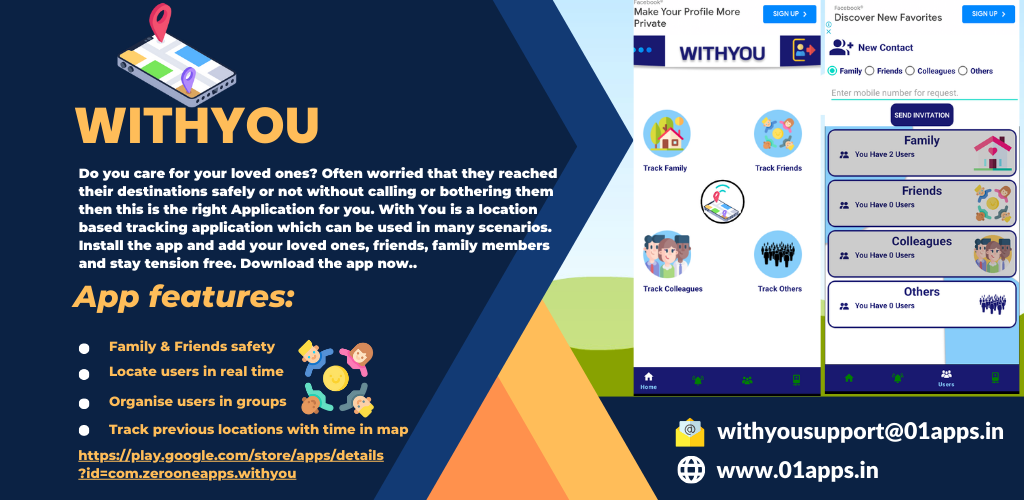