Modern electronics face critical challenges, including high energy consumption and increasing design complexity. In this context, magnonics — the use of magnons, or quantized spin waves in magnetic materials — offers a promising alternative. Magnons enable efficient data transport and processing with minimal energy loss. With the growing demand for innovative computing solutions, ranging from 5G and upcoming 6G networks to neuromorphic computing (mimicking functions of the brain), magnonics represents a paradigm shift that redefines how devices are designed and operated. Developing an innovative magnonic processor that enables highly adaptive and energy-efficient computing was a challenge that Andrii Chumak of the University of Vienna’s Nanomagnetism and Magnonics Group and his collaborators successfully met.
Success Through Trial and Error
Noura Zenbaa, first author of the study, together with her colleagues around Dieter Süss, Physics of Functional Materials at the University of Vienna, built a unique experimental setup using 49 individually controlled current loops on an yttrium-iron-garnet (YIG) film. These loops created tunable magnetic fields to control and manipulate magnons. Using an “inverse-design” approach, the team allowed algorithms to determine the optimal configurations to achieve desired device functionalities, significantly streamlining the design process. After more than two years of development and testing, the team overcame many challenges. “It was a tough journey but seeing it all come together with our first successful measurement was incredibly rewarding”, said Noura Zenbaa.
Creating Greener Technologies
The team’s prototype demonstrated two key functions: acting as a notch filter (a component that blocks specific frequencies) and as a demultiplexer (a device that routes signals to different outputs). These capabilities are crucial for next-generation wireless communications like 5G and 6G. Unlike traditional systems, which require custom components, this versatile hardware can be adapted for various applications, reducing complexity, costs, and energy consumption. Ongoing research shows that the device can also perform all logical operations on binary data, and when scaled, it could rival traditional computers. The team plans to integrate this technology into neuromorphic computing and other advanced systems. While the current prototype is large and energy-intensive, shrinking it to under 100 nanometers could unlock exceptional efficiency, paving the way for low-energy, universal data processing and creating solutions for greener computational technologies. “This project was a bold venture with many unknowns”, reflects Andrii Chumak, senior author of the study. “Yet, our initial measurements confirmed its feasibility – this concept works. Our results highlight how artificial intelligence is transforming the field of physics, much like ChatGPT is reshaping text writing and education.”
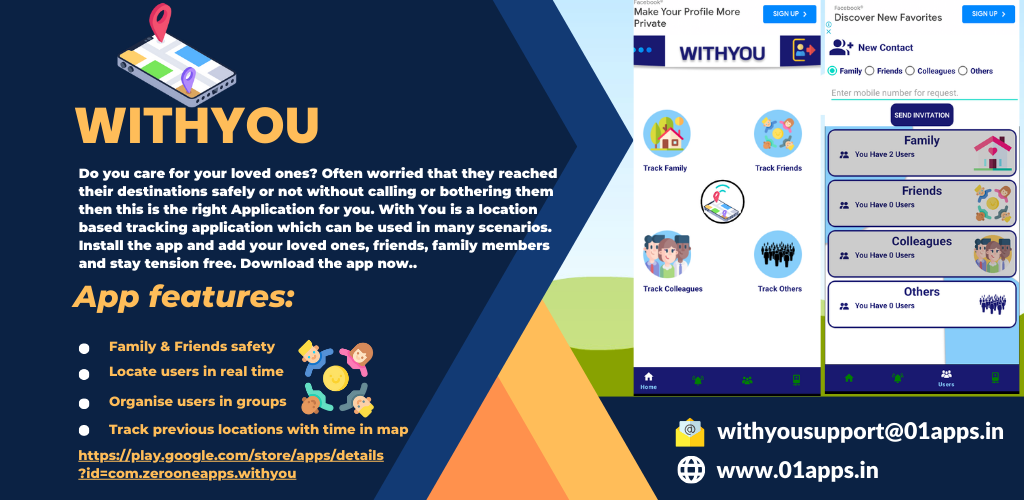