Researchers have found that out of the more than 300 COVID-19 machine learning models described in scientific papers in 2020, none of them is suitable for detecting or diagnosing COVID-19 from standard medical imaging, due to biases, methodological flaws, lack of reproducibility, and ‘Frankenstein datasets.’
The team of researchers, led by the University of Cambridge, carried out a systematic review of scientific manuscripts – published between 1 January and 3 October 2020 – describing machine learning models that claimed to be able to diagnose or prognosticate for COVID-19 from chest radiographs (CXR) and computed tomography (CT) images. Some of these papers had undergone the process of peer-review, while the majority had not.
Their search identified 2,212 studies, of which 415 were included after initial screening and, after quality screening, 62 studies were included in the systematic review. None of the 62 models was of potential clinical use, which is a major weakness, given the urgency with which validated COVID-19 models are needed. The results are reported in the journal
Nature Machine Intelligence.
Machine learning is a promising and potentially powerful technique for detection and prognosis of disease. Machine learning methods, including where imaging and other data streams are combined with large electronic health databases, could enable a personalised approach to medicine through improved diagnosis and prediction of individual responses to therapies.
“However, any machine learning algorithm is only as good as the data it’s trained on,” said first author Dr Michael Roberts from Cambridge’s Department of Applied Mathematics and Theoretical Physics. “Especially for a brand-new disease like COVID-19, it’s vital that the training data is as diverse as possible because, as we’ve seen throughout this pandemic, there are many different factors that affect what the disease looks like and how it behaves.”
“The international machine learning community went to enormous efforts to tackle the COVID-19 pandemic using machine learning,” said joint senior author Dr James Rudd, from Cambridge’s Department of Medicine. “These early studies show promise, but they suffer from a high prevalence of deficiencies in methodology and reporting, with none of the literature we reviewed reaching the threshold of robustness and reproducibility essential to support use in clinical practice.”
Many of the studies were hampered by issues with poor quality data, poor application of machine learning methodology, poor reproducibility, and biases in study design. For example, several training datasets used images from children for their ‘non-COVID-19’ data and images from adults for their COVID-19 data. “However, since children are far less likely to get COVID-19 than adults, all the machine learning model could usefully do was to tell the difference between children and adults, since including images from children made the model highly biased,” said Roberts.
Many of the machine learning models were trained on sample datasets that were too small to be effective. “In the early days of the pandemic, there was such a hunger for information, and some publications were no doubt rushed,” said Rudd. “But if you’re basing your model on data from a single hospital, it might not work on data from a hospital in the next town over: the data needs to be diverse and ideally international, or else you’re setting your machine learning model up to fail when it’s tested more widely.”
In many cases, the studies did not specify where their data had come from, or the models were trained and tested on the same data, or they were based on publicly available ‘Frankenstein datasets’ that had evolved and merged over time, making it impossible to reproduce the initial results.
Another widespread flaw in many of the studies was a lack of involvement from radiologists and clinicians. “Whether you’re using machine learning to predict the weather or how a disease might progress, it’s so important to make sure that different specialists are working together and speaking the same language, so the right problems can be focused on,” said Roberts.
Despite the flaws they found in the COVID-19 models, the researchers say that with some key modifications, machine learning can be a powerful tool in combatting the pandemic. For example, they caution against naive use of public datasets, which can lead to significant risks of bias. In addition, datasets should be diverse and of appropriate size to make the model useful for different demographic group and independent external datasets should be curated.
In addition to higher quality datasets, manuscripts with sufficient documentation to be reproducible and external validation are required to increase the likelihood of models being taken forward and integrated into future clinical trials to establish independent technical and clinical validation as well as cost-effectiveness.
###
This part of information is sourced from https://www.eurekalert.org/pub_releases/2021-03/uoc-mlm031221.php
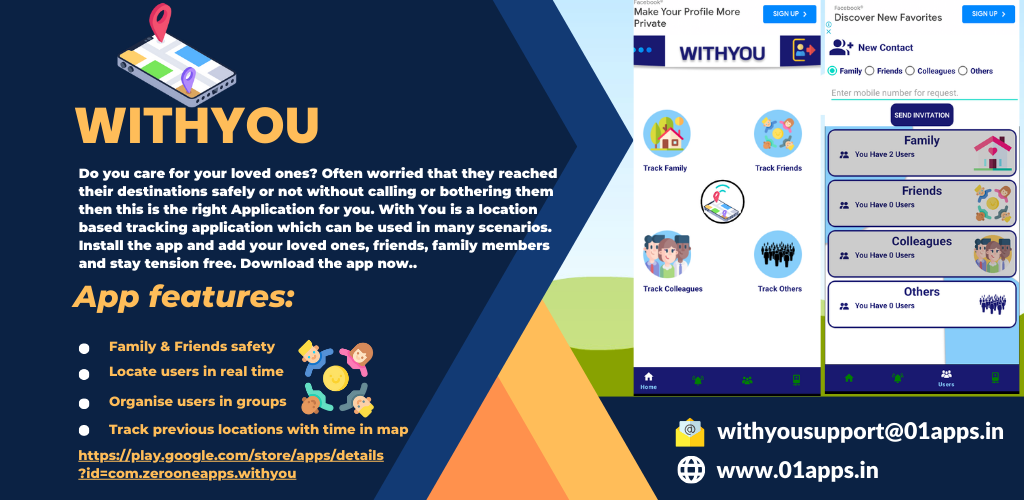