“Within the field of health economics and outcomes research, generative AI has the potential to transform HTA evidence generation methods,” said author Jagpreet Chhatwal, PhD, Director of the Institute for Technology Assessment at Massachusetts General Hospital, Boston, United States. “Ensuring human oversight, transparency, and adherence to ethical standards is critical as we navigate this transformative era,” noted Chhatwal, who is also an Associate Professor at Harvard Medical School and core faculty member of the Center for Health Decision Science at Harvard University.
The report identifies 3 key areas where generative AI, including large language models (LLMs), could significantly impact HTA processes:
- Systematic Literature Reviews: Generative AI has the potential to assist in automating aspects of systematic literature reviews by proposing search terms, screening abstracts, extracting data, and generating code for meta analyses.
- Real World Evidence: Generative AI demonstrates capability in analyzing large collections of real-world data, including unstructured clinical notes and imaging, making it easier to extract valuable insights from complex healthcare data.
- Health Economic Modeling: AI tools can assist in various stages of health economic model development, from conceptualization to validation, potentially increasing efficiency in this critical aspect of HTA.
Despite their potential, current generative AI applications are in their early stages and present limitations, including issues of scientific validity and reliability, risk of bias and impact on equity, and regulatory and ethical considerations. These will continue to require careful evaluation and human oversight in their application to HTA for the foreseeable future.
The authors provide several important suggestions for HTA agencies on responsibly integrating generative AI into their workflows. They recommend establishing clear guidance on the appropriate use of LLMs. It will be important to standardize and harmonize processes across agencies, ensuring transparent reporting. A focus on health equity will enhance the quality and efficiency of submissions and ensure that appropriate populations are represented in HTA analyses. Additionally, investing in training for the HTA workforce will be essential for the responsible and inclusive use of generative AI technologies.
“The field of generative AI provides potentially transformative tools to augment and support the efficient generation of evidence to support HTA under human supervision,” noted Chhatwal. “Because of the nature of these models, there is added complexity in using them and evaluating the validity and reproducibility of their results. It is expected, as with all new technologies, that both user expertise with foundation models, and the actual performance of foundation models themselves, will improve rapidly in the near future.”
Additional coauthors include Rachael L. Fleurence, PhD, Jiang Bian, PhD, Xiaoyan Wang, PhD, Hua Xu, PhD, Dalia Dawoud, PhD, and Mitch Higashi, PhD. The authors are currently developing a framework for health economics and outcomes research (HEOR) professionals to evaluate the quality, transparency, and rigor of LLM-assisted research. For more information on ISPOR’s AI in HEOR Strategic Initiative, please visit here.
Suggested reading:
- Consolidated Health Economic Evaluation Reporting Standards for Interventions That Use Artificial Intelligence (CHEERS-AI)
- Machine Learning Methods in Health Economics and Outcomes Research—The PALISADE Checklist
- Do People Favor Artificial Intelligence Over Physicians? A Survey Among the General Population and Their View on Artificial Intelligence in Medicine
- Systematic Review of Health Economic Evaluations Focused on Artificial Intelligence in Healthcare: The Tortoise and the Cheetah
- Assessing the Economic Value of Clinical Artificial Intelligence: Challenges and Opportunities
- Fulfilling the Promise of Artificial Intelligence in the Health Sector: Let’s Get Real
###
ABOUT ISPOR
ISPOR—The Professional Society for Health Economics and Outcomes Research (HEOR), is an international, multistakeholder, nonprofit dedicated to advancing HEOR excellence to improve decision making for health globally. The Society is the leading source for scientific conferences, peer-reviewed and MEDLINE®-indexed publications, good practices guidance, education, collaboration, and tools/resources in the field.
Website | LinkedIn | Twitter (@ispororg) | YouTube | Facebook | Instagram
ABOUT VALUE IN HEALTH
Value in Health (ISSN 1098-3015) is an international, indexed journal that publishes original research and health policy articles that advance the field of health economics and outcomes research to help healthcare leaders make evidence-based decisions. The journal’s current impact factor score is 4.9 and its 5-year impact factor score is 5.6. Value in Health is ranked 5th of 118 journals in Health Policy and Services, 15th of 174 journals in Health Care Sciences and Services, and 56th of 597 journals in Economics. Value in Health is a monthly publication that circulates to more than 55,000 readers around the world.
Website | Twitter (@isporjournals)
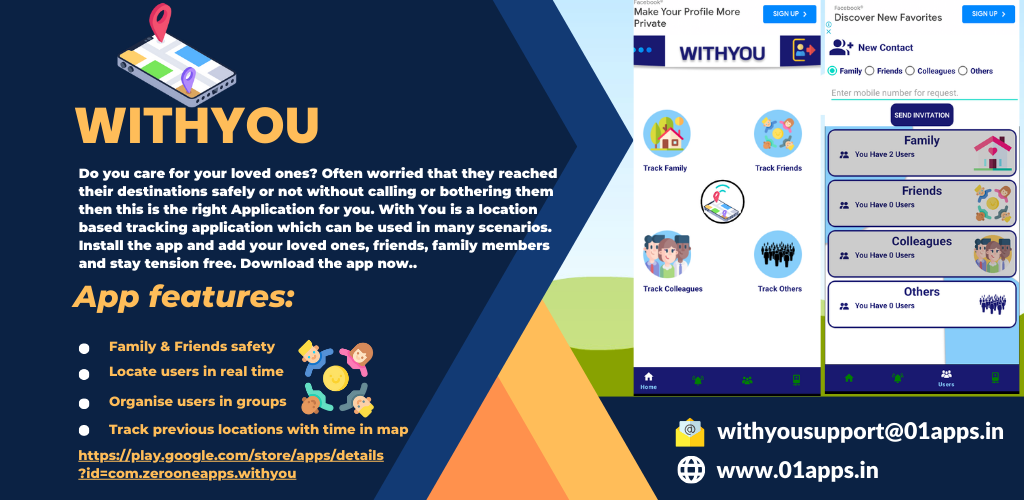