On January 24, 2025, a research team led by Professor Bing Xu from Tsinghua University’s Department of Earth System Science published a transformative study (DOI: 10.34133/remotesensing.0379) in Journal of Remote Sensing. Leveraging the power of LiDAR and satellite data fused with machine learning and deep learning technologies, the team tackled persistent challenges in urban forest monitoring. This innovative research opens new avenues for urban ecological studies and resource management, offering practical tools for city planners and environmental stewards.
At the heart of this study lies the Seasonal Tree Height Neural Network (STHNN), a state-of-the-art model that achieved unparalleled accuracy in estimating tree heights (R² = 0.80, MAE = 1.58 meters). The team’s use of SHAP (SHapley Additive exPlanations) for feature optimization streamlined the model by eliminating 23 non-essential variables among 52 variables —enhancing accuracy while reducing computational demands. Impressively, the STHNN model demonstrates robust generalizability, accurately estimating tree heights across seasons and diverse geographic regions. Drawing from a rich dataset spanning 2018 to 2023, the study combined LiDAR and satellite data to analyze spectral, vegetation, texture, polarization, terrain, and seasonal attributes. The researchers explored a variety of machine learning and deep learning models, with STHNN emerging as the top performer, delivering unprecedented precision in tree height predictions.
A critical innovation in the study was the application of SHAP for feature selection. By trimming 23 less impactful features, the model not only sharpened its predictive capabilities but also significantly streamlined computational efficiency—demonstrating how data science can refine complex ecological modeling. The analysis revealed that Shenzhen’s urban tree heights predominantly range between 6 and 14 meters, showing strong spatial autocorrelation. Notably, the study observed that winter canopy heights are consistently lower than summer canopies, reflecting the natural ebb and flow of seasonal growth. This discovery underscores the necessity of factoring in seasonal dynamics for more effective urban forest management. The research offers urban planners and policymakers a detailed, data-driven blueprint for predicting and managing ecosystem changes within rapidly growing cities. To achieve these results, the research team rigorously tested a suite of machine learning and deep learning models, including multiple linear regression, support vector machines, random forests, XGBoost, and artificial neural networks. Using feature selection tools like random forest importance metrics, genetic algorithms, and SHAP, they identified optimal feature sets that maximized predictive accuracy across models.
Experts from the research team emphasized that this study not only advances the precision of tree height assessments but also introduces powerful new tools for tracking the dynamic evolution of urban forests. The STHNN model stands as a cornerstone for future urban greening strategies and ecological protection, with wide-ranging applications for sustainable city development.
Looking ahead, the technology pioneered in this study holds transformative potential for urban ecology and resource management. Its integration into city planning could revolutionize how green spaces are allocated and how tree-planting strategies are devised, fostering more resilient and sustainable urban environments. In the broader realm of ecological conservation, this tool offers a scalable, data-driven solution for monitoring and preserving urban forests, bolstering biodiversity and critical ecosystem services. Extending this technology to cities worldwide could empower global efforts to combat climate change and accelerate sustainable development.
###
References
DOI
Original Source URL
https://spj.science.org/doi/10.34133/remotesensing.0379
Funding information
This study is supported by the National Key Research and Development Program of China (2022YFB3903703).
About Journal of Remote Sensing
The Journal of Remote Sensing, an online-only Open Access journal published in association with AIR-CAS, promotes the theory, science, and technology of remote sensing, as well as interdisciplinary research within earth and information science.
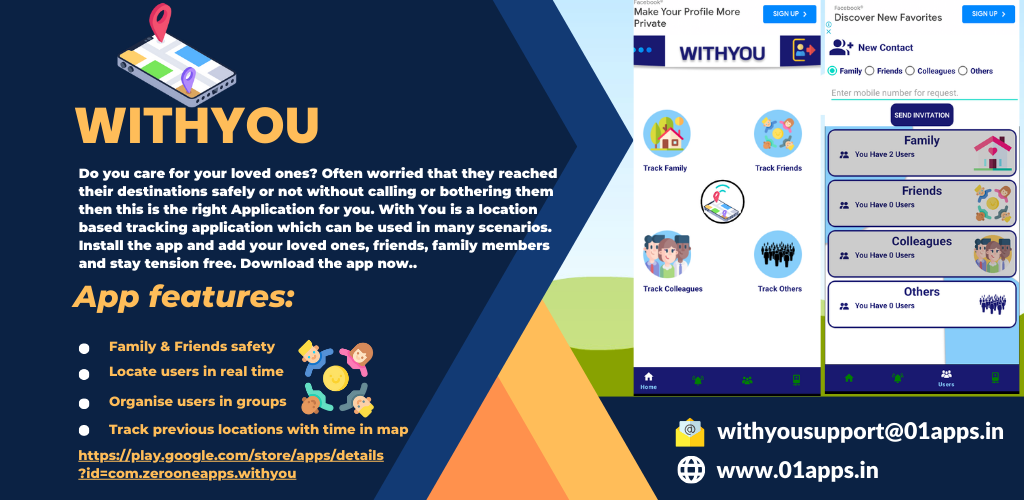