BONUS CONTENT
TRANSCRIPT
[EX MACHINA] NATHAN BATEMAN: One day the AIs are going to look back on us the same way we look at fossil skeletons on the plains of Africa. An upright ape living in dust with crude language and tools. All set for extinction.
ROBERTSON: Are you worried about the robot takeover?
ODEGAARD: I mean, I don’t know. Should I be?
ROBERTSON: Well, many people do have fears about robotics and artificial intelligence. The promise and peril of robotics and AI was the topic of a workshop that was held here at Oregon State. It was really well attended which tells me that it’s a topic that a lot of people are interested in. So, I thought it would be a good idea to focus a whole season on it.
ODEGAARD: That sounds like a pretty good idea. I mean we all see the potential of robotics and AI with world-changing advances in medical treatment, more efficient manufacturing processes, heck maybe even truly autonomous vehicles to whisk us around. But the downsides, or the potential downsides, like manipulatable algorithms influencing world politics, or robots replacing human workers, or AI systems that we humans view as having empathy are certainly there too.
ROBERTSON: But, we probably don’t have to worry about the Terminator. One of my favorite quotes from the promise and peril workshop was “it’s not some magical autonomous being, we’re not creating a new race …
DIETTERICH: it’s not some magical autonomous being, we’re not creating a new race. It is not the new electricity. It is none of those hyped up things. What it is, is we’re building systems that can do mappings from, from our sensors to our actions. And it may make mistakes when they do that. And we have to think carefully about how we apply this technology so that those mistakes don’t lead to catastrophe.
ODEGAARD: Lead to a catastrophe … are you sure we don’t have to worry about the Terminator?
ROBERTSON: Haha, well that’s what we’re about to find out.
ODEGAARD: So, we sat down with two of the leading experts in the field of robotics and AI, who just happen to be here at Oregon State.
DIETTERICH: My name is Tom Dietterich. I’m a, uh, a distinguished professor emeritus in electrical engineering and computer science.
TUMER: I’m Kagan Tumer, I’m a professor in robotics and also the director of the Collaborative Robotics and Intelligence Systems Institute,
DIETTERICH: Also known as CoRIS. Because
TUMER: CoRIS. Yes.
DIETTERICH: Because we make beautiful music as a team.
ODEGAARD: With 27 core faculty, 18 affiliated faculty, and numerous graduate and undergraduate student researchers, CoRIS, that’s C-O-R-I-S is creating a symphony of science, policy, research, and development in the interconnected world of robotics and AI.
ROBERTSON: They’re really focused on that interconnectedness. They’re looking at the policy implications and the ethics of their systems with an emphasis on evaluating what impacts the ongoing robotics and AI revolution will have on individuals, society, and culture.
ODEGAARD: Ya, one of the coolest parts about speaking with Kagan and Tom was getting a glimpse into their brilliant minds. They’re thinking about this stuff on a level that’s pretty mind-blowing, yet at the same time they can explain it in such a crystal clear way.
ROBERTSON: I know, right?! Not only top in their specific area, but big thinkers, and fun guys too. But maybe we should let the people hear for themselves.
ODEGAARD: Yep but first we should introduce ourselves.
ROBERTSON: Good idea. I’m Rachel Robertson.
ODEGAARD: I’m Jens Odegaard, and we’re your hosts.
[MUSIC: “The Ether Bunny,” by Eyes Closed Audio, used with permission of a Creative Commons Attribution License .]
JON THE ROBOT: From the College of Engineering at Oregon State University, this is “Engineering Out Loud.”
ODEGAARD: That narrator was Jon, a joke-telling robot at Oregon State. You’ll probably hear more from Jon throughout the season. But, for now, let’s jump right into the question of why we humans are so fascinated with creating these robotic and AI systems in the first place.
[MUSIC: “Go to Sleep” by Podington Bear, used with permission of a Creative Commons Universal License.]
DIETTERICH: Well, I guess I’ve always been interested in the, almost the philosophical question of how is it that we learn about the world. We were born with some knowledge, but obviously our children learn incredibly quickly about all kinds of things from social norms to just what things feel like and how to pick them up. And so physical interaction but also social interaction. And the question is, how does that happen and can we replicate that in, in software or hardware or synthetic biology or whatever. And so it’s the fundamental question of how we learn about the world. Philosophers had been wondering about this for a long time, uh, and they don’t have the answer and I suppose neither do we, but we, we’re making progress on it.
ODEGAARD: This philosophical question of can we replicate our own capabilities and capacity for learning and knowledge is balanced by the question of whether we can create the robots and AI systems to make a future science fiction fantasy a present-day reality.
TUMER: I’m almost not sure which came first. My interest in this science fiction type AI and robots or the scientific side because I always remember thinking it would be so cool if we had robots and AI who could do things that now have to send a person to do: talk about exploring a planet, talk about, uh, looking at different places on earth, but now also more mundane things like a, whether it’s driving or helping you around the house. It’s not so much nicer, but the fact that we could now be thinking about other things.
Maybe go back to the philosophical questions because you have the time and energy to do that now rather than do the more day to day tasks. And I’ve always been fascinated with pushing that envelope of what’s possible and what’s really out there. And I think part of it is also that if you look back 20 years, what we thought was out there, a lot of those things exist today.
ODEGAARD: Twenty years ago I was 13 years old. Our desktop computer was running Windows 98, the dial up internet kind of worked, and Google was just coming on the scene.
Today my kids are using Google Assistant to voice search cute puppy videos. And most nights at bedtime we watch a “How It’s Made” clip on YouTube. Though some things are still made by hand, most of the episodes feature robots building things.
ROBERTSON: When I was 13, which was 40 years ago.
ODEGAARD: You do the math!
ROBERTSON: Rosie the Robot was doing all kinds of useful things for the Jetsons. And it’s so strange how the future we imagined when we were kids is now becoming a reality. But even though we’re used to a level of AI and robotics in our everyday lives, I still feel like sometimes we find ourselves wondering what exactly robots and AI are. When I started really digging into the recordings of the promise and peril workshop and thinking more deeply about it, I realized I had some basic questions.
ROBERTSON: …and one of the things that I realized is that we have these three different things that are, there’s actually a lot of crossover. So we’ve got robotics, we’ve got AI, we’ve got autonomous vehicles, and I didn’t really know how to define those things separately or if they even should be defined separately.
DIETTERICH: Well, I think in the presentation we talked about artificial intelligence as smart software. So if we think about the self-driving vehicle, this mythological creature, it would have hardware, and sensors and control technology in it, that would be the, the mechanical engineering and robotics foundation. And then it would have software which would be doing things like the computer vision, recognizing, oh, that’s a pedestrian, that’s a bicyclist. This is my lane that I’m supposed to stay in.
But it is very tricky. Because one of the research areas that we’re exploring here at Oregon State is the simultaneous design of the hardware and the software. The tradition has been for computer scientists and roboticists to be kind of separate and you know, in computer science there’s this idea that dates back to the ‘60s that you build a general purpose computer and then programmers can make it do anything we want. And there’s been a similar kind of abstraction, especially on the computer science side of robotics, which is we’ll make a general purpose robot and then give it to the programmers and they’ll make things happen. That doesn’t actually work all that well.
TUMER: Right
DIETTERICH: And so usually you get something that’s very clumsy. It takes a huge amount of energy and is also just really hard to program.
ODEGAARD: At CoRIS, researches including Jonathan Hurst, an associate professor of robotics, are working on ways to overcome this clumsiness and inefficiency by integrating software and hardware together from the start. Jonathan is doing this in robots with legs and arms called Cassie and Digit. And you’ll actually hear more from Jonathan later in the season, but for now back to Tom.
DIETTERICH: So one of the most exciting things I think about Jonathan Hurst’s work is that the walking and energy storage and all of this is done in the hardware.So the software can work at a much higher level and say, well, where are we going? How are we trying to get there? And not, where do I put my foot in the next millisecond? And so that turns out to be a much better way of doing things. And that is clearly the future direction in robotics and artificial intelligence is that the line will become harder and harder to draw because they’re designed together.
ODEGAARD: To further help explain this blurring of the lines between robotics and AI, Kagan shifts gears from autonomous cars to the granddaddy of strategic board games.
[MUSIC: “Sunset Stroll into the Wood” by Podington Bear, used with permission of a Creative Commons Universal License.]
TUMER: I’ll give you an example of chess. If you think of chess as a board, a grid on your computer screen, that is an entirely AI solution to that to make it play chess. So when you talk about chess players, that are software, that’s a purely AI system because it’s simply making decisions based on reading what’s going on at that point. Take the complete extreme example and say that you have this arm that has three fingers on top and you mechanically tweak that to move your own piece. So two humans are playing, but one of them is using this weird robotic arm to move it. That’s the pure robotics, no software, extreme. Both of those are extremes when you start to operate in the real world. What if you had a like a Cassie or Digit. What if that happened to be playing chess? While you would have that software that needs to learn how to make the moves, but you also have to have some kind of vision to look at the board if you want to move the piece, right? So to me that the AI and robotics blur in that part where all of that interacts with the real world.
ODEGAARD: To fully bring the point home, Kagan then brings it all the way back around to autonomous vehicles. He builds off the example that Tom gave earlier, of pedestrians interacting with these cars.
TUMER: But now you’re not just moving chess pieces, right? You see a bicycle, you see another human, you see another car, you have to detect those. You have to understand what they are, whether they’re moving. So get, build, an internal map of what’s going on and then make a decision and then act upon that. So that is AI, and robotics, and autonomous vehicles, all of that together.
ROBERTSON: That explanation pretty much set up our whole season on robotics and AI.
ODEGAARD: Almost. As he alluded to, once you start developing these systems, you have to figure out how to roll them out into the world. It’s no longer just a simple chess algorithm, it’s real people and real machines trying to work together in real situations.
ROBERTSON: This is where things get exciting, and difficult, and ambiguous.
DIETTERICH: Full autonomy is a long way off. So that means there is a driver in the car who is maybe interacting with some sort of interface to communicate with the car to make some of those decisions to step in when there are problems. And so that brings us to the human and robot interaction which is probably the most difficult and most fascinating part of it. Even the car interacting with a pedestrian in a crosswalk, how does the car signal to the pedestrian I see you? I’m not going to hit you. And the pedestrian might say, oh, go ahead, wave their arm. How does the car know it’s okay for it to go instead? I mean, it’s very challenging.
TUMER: I think one of the things that we really are setting up as our next challenges is, is how do you take these single task AI or robots into the real world? Even chess example that we started with, there’s a very clear, you played a game, there’s a win loss, so you can actually start to learn and plan around that. When you operate in the real world, there is no real obvious end of the game or win loss.
Even a fully autonomous car is not fully autonomous because it didn’t wake up that morning and decide I want to drive, right. That that car is programmed to do things. So it’s doing what it’s meant to be doing.
I don’t know where that line of autonomy is. Maybe we should say fully competent in doing whatever its task was. We’re still a ways from that certainly for driving. But I think we should be thinking more in terms of it’s not a single task or a game, but it’s a longer term operation and things change. And how do you design that? That’s where I see a key challenge in the near future.
ROBERTSON: Working toward meeting this challenge is done by building smart software, which is done one of three ways. I’ll paraphrase Tom here. The first way is rule-based programming where software makes decisions based on a strict set of rules. Think tax preparation software. You input the answer and the software makes a decision based on the rules of the tax code that are programmed in.
ODEGAARD: The second way to program is by building in lots of training examples from which the computer can learn to predict what will happen next and then make a decision from that prediction. Imagine a software system that takes a bunch of video from a four-way stop sign, and based on what happens in the video, the computer starts to predict who should go next based on the behavior it observed.
ROBERTSON: The third way is to build software that doesn’t just predict or imitate human behavior. Instead this software is built with a goal, rather than a process in mind, and it learns as it goes along.
DIETTERICH: So we call this optimization because we don’t teach the computer to imitate what people are doing. We instead say, here is your goal, or we call it sometimes the objective. So in, for a chess playing program, we might say, your goal is to win at the game of chess. And then we just let it play thousands, millions, billions of games in simulation. And we have learning algorithms now just in the last five years that can learn to play at levels that exceed human performance and find novel moves that people have never tried before. So the, the first two don’t lead to very much in the way of surprises. I mean software that we hand code. There are bugs in it and those are bad surprises, and we do have to test them and so on.
And the same with, uh, programming a computer to imitate us. It won’t do that perfectly. It will make mistakes and we will need to debug that. But the place where we get the big surprises is that when we say, here is your goal, and then it goes off and finds a way of achieving that goal that is not really what we wanted. So we didn’t really write the goal down properly. And so then we have to change that and do it again and then change it and do it again. And so it’s quite difficult to know when we have specified the correct behavior, and it gets more and more difficult the more open the world is, the more it involves social and ethical concerns as opposed to just moving pieces around in the chessboard.
ODEGAARD: Kind of the tagline, or the goal, of the robotics program here at Oregon State, is robots in the real world …
How are we, how are we approaching these ethical concerns and incorporating that into the development and deployment of these systems that we’re working on?
TUMER: That’s a great question. In fact, we have different layers where we are doing that. And one of them is that from the robotics program, that’s a tagline and it’s not just about the research. We also are in the business of education. And we are one of the only places I know where we are offering robot ethics courses. It’s not engineering ethics or tech ethics where you learn not to plagiarize and this and that. It’s the ethics of the technology where students read and comment on autonomous weapons or privacy issues. So they, our intent there is that when they graduate, they’ve at least been exposed to the topics. I’m not claiming to teach ethics in a class. You can’t teach ethics in a class. But what you can do is raise awareness of these issues and give students a concept of how would you even discuss this?
ROBERTSON: These discussions revolve around trying to answer questions like …
TUMER: What does privacy mean? Having a smartphone in your house, it’s already a camera and a microphone at all times. Having Alexa is a problem. Now imagine having a robot that not only has all those capabilities but also moves around.
How do you tell that robot, well, this area is off limits. That’s the bathroom. To you and I, that’s obvious. You’re smiling because you’re like, well obviously we didn’t go and take pictures in a bathroom. But you know, that’s not obvious to a device that doesn’t really have those boundaries. So you need to be aware of privacy in a sense that the technology to say this area isn’t for you, has to also be built into that. So it’s not just an ethicist coming and telling us, don’t take bathroom pictures. We know that. But how do you come up with the technology that can enforce that? Those are the kind of topics we’re looking at as well.
ROBERTSON: Here’s a real-world example of new technology and ethical implications. Robot caregivers are beginning to be used to help take care of the sick and elderly. And these robots are designed to look cute and programmed to have so called personality to help patients be more comfortable interacting with them. Sounds good right? Well …
TUMER: If you make that robot cuter and cuddlier and occasionally tell jokes, they’re more likely to follow those directions. So you’re trying to achieve something. But that’s where the ethics come in as well because you are at that point, you are using that robot to manipulate that person. You need to decide and we need to decide as a society whether the ends justify the means. Certainly you might get to a point where they take their medicine more often, but maybe that’s the goal, and maybe that’s okay and maybe it’s not okay to start to form attachment to a, an inanimate object, which is what it is at the end of the day to get those goals. So that research is very interesting because it’s showing you what’s capable. But once again, we need to decide what we are willing to accept as ethical or not as we start to deploy that. Right now it’s still at the research stage, but if you start to say your insurance is going to provide you one of these and now you must follow their instructions, there’s a very interesting choice you’re making there in what is acceptable and what is not.
DIETTERICH: Right. Because we’re replacing what we hope is genuine human empathy of a human caregiver with fake simulated empathy because the robot does not have human experience on which and is not made of biological stuff. It doesn’t know what pain is or what happiness is or any of these things. It can just simulate it. And I find it very troubling that someone might, especially a dementia patient, might develop an emotional attachment to what is essentially a trick, a fake thing.
[MUSIC: “The Speed of Life” by Poddington Bear, used with permission of a Creative Commons Universal License.]
ODEGAARD: Another area of ethical concern is ensuring that human biases and poor behaviors aren’t passed along to the robotic and AI systems through the programming.
DIETTERICH: This becomes a real problem, when we create AI by having it imitate us. There’s quite a lot of evidence that in these things like stop and frisk programs that the police have done, that minorities are stopped and frisked a lot more than majority people. And if you train the computer system to imitate that, you are just automating this bad behavior. So there has now become a huge area of research on what’s called fairness, accountability, and transparency. It goes by the unfortunate name of fat AI, which is trying to look at formalizing some of these aspects. What does it mean to be fair? What does it mean to be transparent? Should people have a right to get an explanation out of these systems about why it made, the decision it did.
Why was I denied a credit card? What can I do about that so that I’m not denied the next time? So you want not only the why but some actionable why, and this is leading to all kinds of exciting and interesting conversations. Technologists tend to want to find a technical solution to these questions. But really it’s a socio-technical problem and we need to understand the broader society, the broader context in which the systems are, are living. So as you can hear, there’s a real theme here nowadays, which I think in the early days of AI, we, we did what you might call context-free research. We would just work on a system that would work on a narrow task all by itself and didn’t have to pay any attention to the surrounding context. But now, if you’ve built a system that can recognize objects, well maybe you say, well, now I want to recognize faces.
Now you have this huge rich context that involves privacy, security, policing, all kinds of things, civil liberties and the definition of what’s correct is no longer something that is up
[MUSIC: “The Speed of Life” by Poddington Bear, used with permission of a Creative Commons Universal License.]
just to the engineer alone. You need lawyers, historians, sociologists, anthropologists, everyone, all of the potential stakeholders need to be at the table and providing their perspectives and guidance.
ODEGAARD: To help facilitate this broader input, Kagan, and especially Tom, are helping lead the ethical policy conversation at a national and international level.
DIETTERICH: The National Science Foundation is funding a 20-year roadmap for the future of AI and robotics. And I was in charge of the machine learning part of that.
DIETTERICH: And so we were trying to imagine why, where will we be over the next 20 years and what are the topics we need to be studying. And we also tried to take a slice through the technology, that it was new, uh, to force people to think in new ways.
TUMER: So we definitely are playing in shaping the policy of not only what research should be done, but how it should be done and, and you know, at the end of the day, it’s our responsibility to make sure that robotics and AI are done in the way that we would want it to be done.
ROBERTSON: This is so important, because we may fear the technology, but the reality is that humans are behind it and responsible for it. It’s on us. We are the intelligence behind the AI.
TUMER: We talk about AI as a thing. You know, AI is a noun. AI does this, AI does that. I mean that already is putting a picture in your mind that there’s this little AI like thing that has all these cognitive features. And if we were to replace the word AI with physics, I mean you don’t say, when somebody gets shot, you don’t say physics killed this person. Even though the expanding air propelled the object where this physics was helpful in designing that, right?
It’s just that, that’s the big umbrella field where you study all these things. And I feel if similarly we were to label these parts of AI with what they are. Like you said: Software does this, inference engine designed by this data does this.
We say AI is biased. Well, no, AI wasn’t biased. What you really mean is that the decision system based on biased data exposes companies hiring practices. Now that’s not quite as exciting to people that say AI is biased. But it’s a much more accurate reflection of what that …
DIETTERICH: Ya, the AI didn’t go rogue.
TUMER: Exactly.
DIETTERICH: But, the programmers made a mistake.
TUMER: They imply AI having this volition and doing things when they use those words. Whereas all it is is either poorly designed software or software designed just fine, but fed poorly curated data. All of those things will be much more apparent if you actually use the word of what it is. Software. Inference engine. Even a robot is a vague term, right? Bipedal delivery mechanism, maybe.
And then you no longer worry about this crazy intelligent AI from your movie perception, which is what, every time we say AI, we are putting that image in people’s minds that there’s some rogue elements, somewhere doing something kind of nefarious behind it, but it’s really a piece of software that you designed and trained with something. That’s all it is. So, pet peeve, we should be calling things what they are and you have AI as a big umbrella term that captures them all, but not put AI in every title of everything that we put out there because they’re very different types of things.
ODEGAARD: We’ll need some much longer acronyms.
[ALL LAUGH]
DIETTERICH: Ya, you’ll have to explain what an inference engine is.
TUMER: True, but, just, software would be a good place to start.
ODEGAAARD: Yep.
TUMER: Software does this doesn’t sound so scary. It’s just a piece of software that does that.
DIETTERICH: But then also we know how bad software can be.
TUMER: Right.
ODEGAARD: Right
DIETTERICH: Cause you have to reboot your computer every once in a while. So we, we know that it’s not something you should be trusting with your life.
TUMER: Yeah.
[MUSIC: “Blossoming” by Podington Bear, used with permission of a Creative Commons Universal License.]
ODEGAARD: In looking to the future, Kagan sees Oregon State as being the place where research, ethics, policy, and education all coalesce.
TUMER: Well, I think our role is a pretty, uh, clear the way we’ve had a very strong AI group for the last 20, 30 years now. Uh, robotics started about 10 years ago and now we have a very strong presence in robotics. And with the institute what our role is to both educate and drive the research and drive the policies. We want to be the place that has the technology and the research that is cutting edge. And we do. We also want to be the to be talking about all of these things we talked about in terms of for the ethics and the deployment and also the education part.
So I view our role as a leader in education policy and research in all these areas.
DIETTERICH: Ditto.
[ALL LAUGH]
DIETTERICH: That was perfect.
ODEGAARD: Stay tuned for the coming episodes as we meet the researchers and robots putting this into practice.
This episode was produced and hosted by me, Jens Odegaard.
ROBERTSON: And me, Rachel Robertson. Special thanks today to Naomi Fitter who provided us with the audio of Jon the Robot. Naomi is an assistant professor of robotics here and you can expect to hear more from her later in the season. Recording assistance and audio magic was performed by Molly Aton.
ODEGAARD: Our intro music, as always, is “The Ether Bunny” by Eyes Closed Audio. You can find them on SoundCloud and we used their song with permission of a Creative Commons attribution license. Other music and sound effects in this episode were also used with the appropriate license.
ROBERTSON: For more episodes, bonus content, and links to the licenses, visit engineeringoutloud.oregonstate.edu.
ODEGAARD: Also, please subscribe by searching “Engineering Out Loud” on Spotify or your favorite podcast app. See ya on the flipside.
Original post https://alertarticles.info
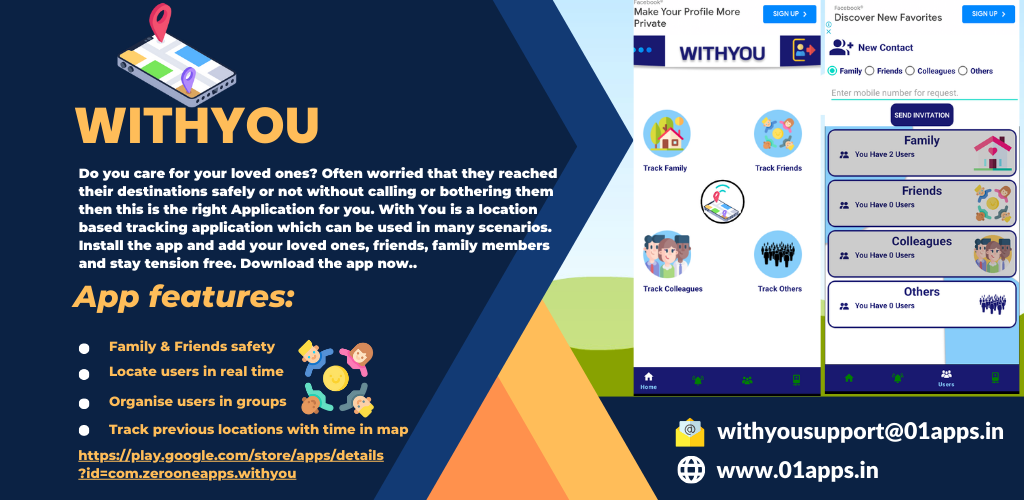