In response to these challenges, a research team from Beijing University of Technology and The University of Tokyo has developed EasyDAM_V4, a pioneering AI-driven approach, published (DOI: 10.1093/hr/uhae007) in Horticulture Research on January 10, 2024. Leveraging a Guided-GAN model, this method enables cross-species fruit image translation, significantly improving labeling accuracy even for fruits with large phenotypic variations. The study’s results highlight substantial improvements in automated labeling, offering a powerful new tool for agricultural image processing.
At the core of EasyDAM_V4 lies a multi-dimensional phenotypic feature extraction technique, which integrates deep learning and latent space modeling to enhance fruit image translation. The method employs a pre-trained VGG16 network to extract shape and texture features from fruit images, which are then fused with original RGB images to enhance the input for the GAN model. A novel multi-dimensional loss function is introduced, with separate loss components for shape, texture, and color features, dynamically weighted using an entropy-based adjustment strategy. This sophisticated approach enables precise control over the generation of fruit features, overcoming previous limitations in handling large shape and texture variations across fruit species.
The effectiveness of EasyDAM_V4 was demonstrated using pear images as the source domain and pitaya, eggplant, and cucumber images as target domains. The model achieved impressive labeling accuracies of 87.8% for pitaya, 87.0% for eggplant, and 80.7% for cucumber, significantly outperforming existing methods. The Guided-GAN model’s ability to generate realistic fruit images with large phenotypic differences represents a major leap forward in automated dataset generation for agricultural AI.
Dr. Wenli Zhang from Beijing University of Technology, one of the lead researchers behind the study, emphasized the impact of this breakthrough:”EasyDAM_V4 represents a significant step toward full automation in fruit detection. By effectively addressing the challenges of shape variance and domain adaptation, this technology not only enhances labeling accuracy but also lays the groundwork for the next generation of agricultural AI and smart orchards.”
The potential applications of EasyDAM_V4 extend far beyond fruit labeling. By streamlining dataset preparation, this innovation could accelerate the development of smart agriculture, enabling more accurate yield predictions, efficient robotic harvesting, and advanced phenotypic studies. Additionally, high-quality labeled datasets will support plant phenomics and breeding strategies, ultimately contributing to more sustainable and resilient agricultural systems.
As AI continues to reshape modern farming, EasyDAM_V4 stands at the forefront, revolutionizing how fruit detection models are built, trained, and deployed—one pixel at a time.
###
References
DOI
Original Source URL
https://doi.org/10.1093/hr/uhae007
Funding information
This study was partially supported by the National Natural Science Foundation of China (NSFC) Program 62276009 and the Japan Science and Technology Agency (JST) AIP Acceleration Research JPMJCR21U3.
About Horticulture Research
Horticulture Research is an open access journal of Nanjing Agricultural University and ranked number one in the Horticulture category of the Journal Citation Reports ™ from Clarivate, 2022. The journal is committed to publishing original research articles, reviews, perspectives, comments, correspondence articles and letters to the editor related to all major horticultural plants and disciplines, including biotechnology, breeding, cellular and molecular biology, evolution, genetics, inter-species interactions, physiology, and the origination and domestication of crops.
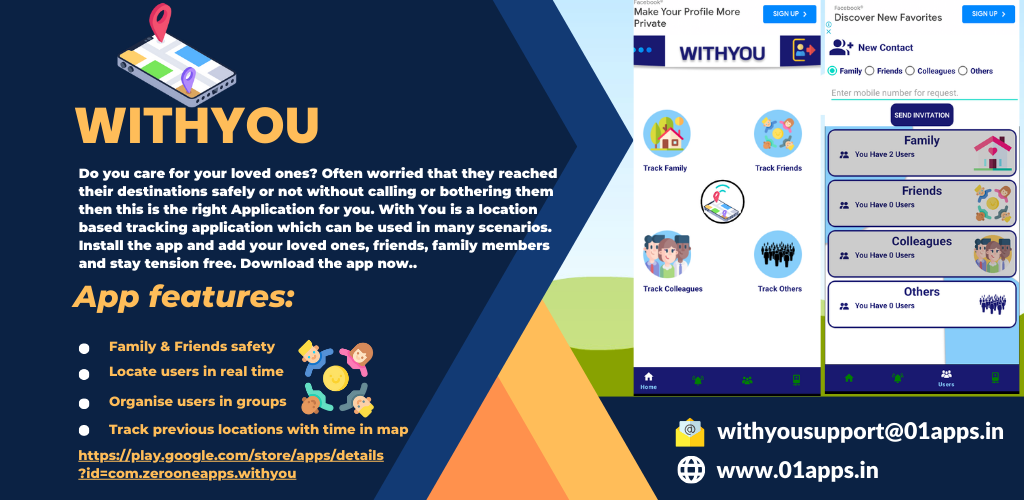