Computers play a significant role in data science and analysis, but despite their speed and accuracy, they are unable to understand nuance and mitigating factors that could make raw data more usable.
Gautam Das, a computer science professor at The University of Texas at Arlington, is leading a team of researchers working to address that shortcoming by increasing the role of humans in the data science pipeline.
Won Hwa Kim, an assistant professor of computer science, is co-principal investigator on the project, which is funded by a $309,583 share of a larger $498,762 grant from the National Science Foundation. UTA alumna Senjuti Basu Roy, a former doctoral student under Das and now an assistant professor of computer science at the New Jersey Institute of Technology, also is collaborating on the project.
The data science pipeline is a sequence of steps by which raw data is collected, cleaned, organized and stored in databases with appropriate features and attributes, then modeled and analyzed for unknown patterns and insights. It can help solve problems in a variety of areas, including the business world and scientific domain.
Humans are involved in the data pipeline at its beginning and end, while automated processes and artificial intelligence algorithms do the majority of the work in between. At the beginning, human contributions are mostly in leveraging a large number of workers for menial tasks, such as labelling photos and noting whether their content is positive, negative or neutral in tone. At the end, a few highly trained data scientists and AI experts create predictive models, deploy them and interpret the outcomes.
The computers’ contributions include organizing and storing data in ways that make it easier to search and find patterns. A significant component of data organization is attribute or feature engineering, which is accomplished through cumbersome automated processes using machine learning or reliant on domain experts that can be slow and expensive.
Das and his team are working to optimize the process by developing a human-in-the-loop framework that makes people a larger part of the attribute engineering segment. Their focus is on medical data and tabular information, where, for instance, a computer can look for information about lengths of hospitalizations but won’t make note of whether and why a patient is readmitted shortly after being released. Such additional information could be used to detect patterns in patient care that otherwise go undetected.
“If a human can look at a dataset and add another column that adds context, it could be very useful in future algorithms,” Das said. “Once the information is labelled, it helps the computer determine what it is, making the process faster and less labor-intensive for the algorithm.”
This new approach to humans-in-the-loop computing is an important step in data analysis, says Hong Jiang, chair of UTA’s Computer Science and Engineering Department.
“Massive amounts of data are available to end users, and the ability to make that data usable and contextual through humans-in-the-loop computing is an important step in making the data pipeline more accessible,” Jiang said. “The work that Dr. Das and his team are doing will make it easier for data scientists to make the best use of the information at their fingertips.”
###
This part of information is sourced from https://www.eurekalert.org/pub_releases/2020-09/uota-mrd092220.php
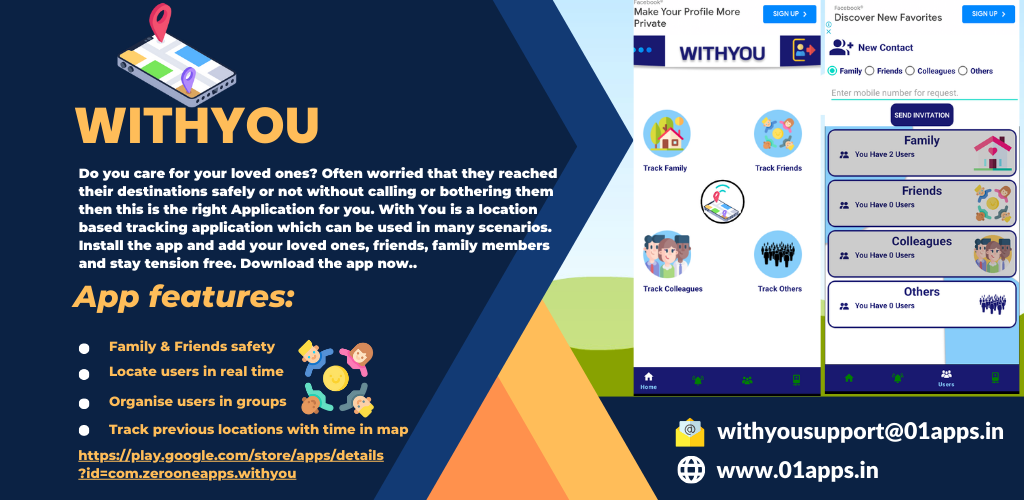