The Science
Linear accelerator operators use computer algorithms to automate some parts of the machine tuning process. These algorithms make fast decisions, but they have not yet incorporated fundamental physics or learned from past mistakes. Scientists created a machine learning algorithm that learns both from experience and from physics simulations. This new method reduced the time for a part of the machine tuning process by 65 percent. This improvement is critical for optimizing user facility operations.
The Impact
The method reduces tuning time for the Linac Coherent Light Source (LCLS), the Department of Energy’s free-electron laser user facility. The method frees operators to focus on other critical tasks and potentially saves hundreds of hours of experiment preparation time per year. Researchers can use this time for actual LCLS experiments. They can also use this method to tune other parts of the accelerator or for individual experiments that use the X-rays produced by LCLS. Other accelerator laboratories around the United States are adopting this method.
Summary
Accelerator operators increasingly rely on computer optimizers to automate some tuning tasks. These optimizations generally lack their human counterparts’ physics knowledge and experience. Scientists used a machine learning tuning technique that combines the speed of autonomous control with the physics and institutional knowledge of human operators to reduce the time needed for a frequently used part of linac tuning by two thirds. The method uses Bayesian optimization, a strategy that can find optimal settings of accelerators and X-ray experiments. Bayesian optimization searches for optimal conditions by building a probabilistic model of the experiment, built on prior experience and new real-time data, to select the most promising point to sample next. The method was applied to maximizing the X-ray pulse energy produced by electrons traversing undulator magnets in the LCLS free-electron laser. During operation of the LCLS, operators “tune” dozens of focusing quadrupole magnets to optimize the lasing by controlling the transverse size of the electron beam in the undulators several times per day. The new computer model learns from the data based on hundreds of scans over months of running. Incorporating a physics model of the electron beam further improves the model by adding relationships between magnet pairs not resolved by prior runs. This approach further speeds up the search, and the benefit grows as the number of control parameters increases.
Funding
Research reported in this publication was supported by funding from the Department of Energy, Laboratory Directed Research and Development program at the SLAC National Accelerator Laboratory in collaboration with research funded by the DOE Office of Science, Basic Energy Sciences’ Accelerator and Detector Research Program. LCLS is a DOE Office of Science user facility.
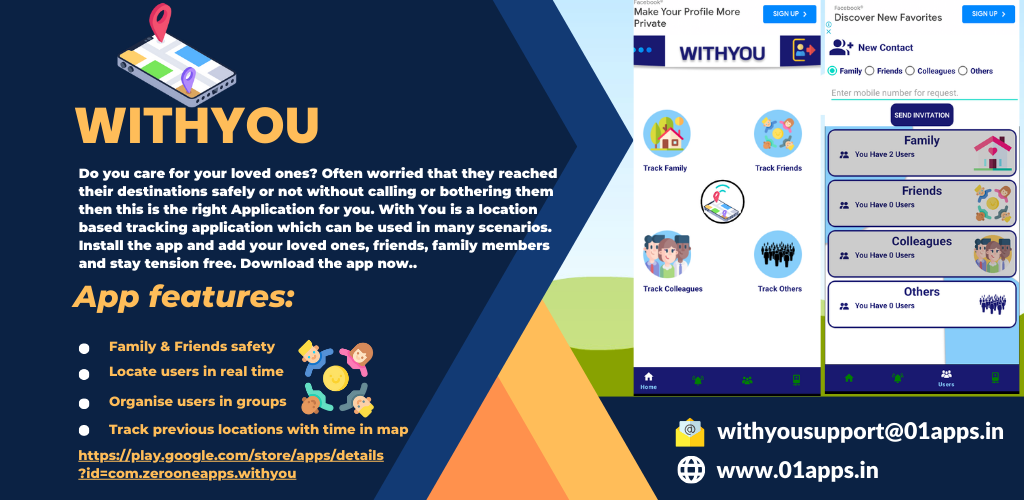