The Cornell-led project’s paper, “Correlator Convolutional Neural Networks as an Interpretable Architecture for Image-like Quantum Matter Data,” published June 23 in Nature Communications. The lead author is doctoral student Cole Miles.
The Cornell team was led by Eun-Ah Kim, professor of physics in the College of Arts and Sciences, who partnered with Kilian Weinberger, associate professor of computing and information science in the Cornell Ann S. Bowers College of Computing and Information Science and director of the TRIPODS Center for Data Science for Improved Decision Making.
The collaboration with the Harvard team, led by physics professor Markus Greiner, is part of the National Science Foundation’s 10 Big Ideas initiative, “Harnessing the Data Revolution.” Their project, “Collaborative Research: Understanding Subatomic-Scale Quantum Matter Data Using Machine Learning Tools,” seeks to address fundamental questions at the frontiers of science and engineering by pairing data scientists with researchers who specialize in traditional areas of physics, chemistry and engineering.
The project’s central aim is to find ways to extract new information about quantum systems from snapshots of image-like data. To that end, they are developing machine learning tools that can identify relationships among microscopic properties in the data that otherwise would be impossible to determine at that scale.
Convolutional neural networks, a kind of machine learning often used to analyze visual imagery, scan an image with a filter to find characteristic features in the data irrespective of where they occur – a step called “convolution.” The convolution is then sent through nonlinear functions that make the convolutional neural networks learn all sorts of correlations among the features.
Now, the Cornell group has improved upon that approach by creating an “interpretable architecture,” called Correlation Convolutional Neural Networks (CCNN), that allows the researchers to track which particular correlations matter the most.
“Convolutional neural networks are versatile,” Kim said. “However, the versatility that comes from the nonlinearity makes it difficult to figure out how the neural network used a particular filter to make its decision, because nonlinear functions are hard to track. That’s why weather prediction is difficult. It’s a very nonlinear system.”
To test CCNN, the Harvard team employed quantum gas microscopy to simulate a fermionic Hubbard model – often used to demonstrate how quantum particles interact in a lattice, and also the many unresolved questions that are raised as a result.
“Quantum mechanics is probabilistic, but you cannot learn probability from one measurement, you have to repeat many measurements,” Kim said. “From the Schrödinger’s cat perspective, we have a whole collection of atoms, a collection of live or dead cats. And each time we make a projective measurement, we have some dead cats and some live cats. And from that we’re trying to understand what state the system is in, and the system is trying to simulate fundamental models that hold keys to understanding mysterious phenomena, such as high-temperature superconductivity.”
The Harvard team generated synthetic data for two states that are difficult to tell apart: geometric string theory and pi-flux theory. In geometric string theory, the system verges on an antiferromagnetic order, in which the electron spins form a kind of anti-alignment – i.e., up, down, up, down, up, down – that is disrupted when an electron hole starts to move at a different timescale. In pi-flux theory, the spins form pairs, called singlets, that begin to flip and flop around when a hole is introduced, resulting in a scrambled state.
CCNN was able to distinguish between the two simulations by identifying correlations in the data to the fourth order.
By repeating this exercise, the CCNN essentially learns what occurrences in the image were essential for neural networks to make a decision – a process that Kim compares to the choices made by people boarding a lifeboat.
“You know when a big ship is about to sink, and people are told, OK, you can only bring one personal item,” Kim said. “That will show what’s in their hearts. It could be a wedding ring, it could be a trash can. You never know. We’re forcing the neural network to choose one or two features that help it the most in coming up with the right assessment. And by doing so we can figure out what are the critical aspects, the core essence, of what defines a state or phase.”
The approach can be applied to other scanning probe microscopies that generate image-type data on quantum materials, as well as programmable quantum simulators. The next step, according to Kim, is to incorporate a form of unsupervised machine learning that can offer a more objective perspective, one that is less influenced by the decisions of researchers handpicking which samples to compare.
Kim sees researchers like her student and lead author Cole Miles as representing the next generation that will meld these cutting-edge and traditional approaches even further to drive new scientific discovery.
“More conservative people are skeptical of new and shiny things,” Kim said. “But I think that balance and synergy between classic and the new and shiny can lead to nontrivial and exciting progress. And I think of our paper as an example of that.”
Co-authors include doctoral student Ruihan Wu and researchers from Harvard.
The research was supported by the U.S. Department of Energy, the National Science Foundation and Germany’s Excellence Strategy.
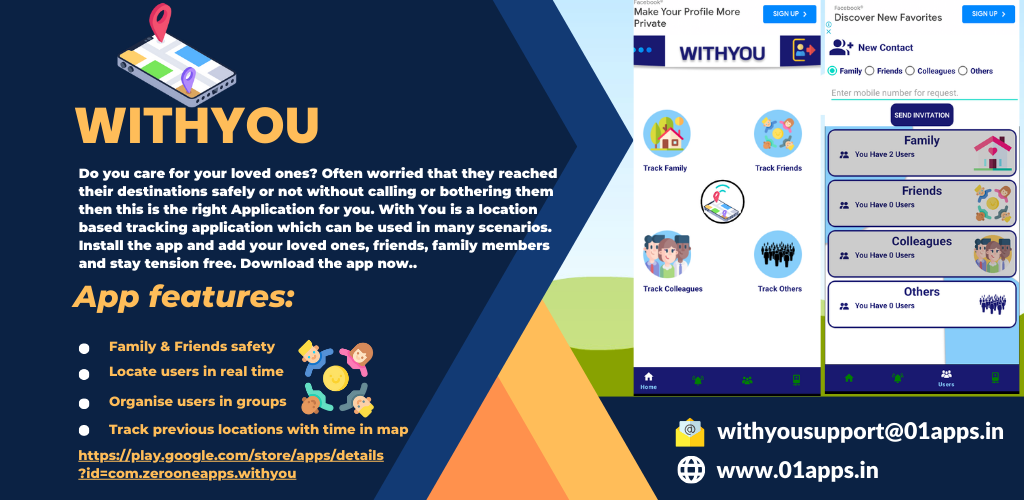