Unlike in comic books, real species don’t tend to explode with many superpowered mutants. But mutations that lead to new abilities are very real. A team from the University of Michigan used PSC’s NSF-funded Neocortex supercomputer to simulate hypermutators — mutants with an ability to mutate more quickly. Neocortex allowed the scientists to expand their artificial populations from the tens of thousands in earlier simulations to billions, helping to explain the relative lack of hypermutators in real organisms. But they also showed how some conditions can lead to hypermutators dominating a species.
It’s a sci-fi and comic-book staple. Mutations lead to superheroes, supervillains, and (at least in more modern tellings) the inevitable question of where we “normal” people fit in when there are demigods among us.
Of course, that doesn’t happen in real life. But it’s not that crazy to ask why. Why don’t we see a lot more mutations leading to new abilities? Part of the issue is that a new mutation can easily — and is more likely to — lead to a genetic disease or other disadvantage in survival than to an advantage. But mutations that benefit an organism are very real and do arise, for example, making viruses and bacteria more dangerous.
Pioneering simulations of hypermutator evolution conducted by Yevgeniy Raynes of Brown University added a twist. In these sims, every “organism” had a 32-bit number as a “genome,” consisting of 32 zeros or ones. This super-simplified “DNA sequence” determined its fitness and the frequency with which it mutated. This approach allowed scientists to simplify evolution and mutation enough to run on desktop computers.
They found something amazing. In small populations, between 500 and 1,000 individuals, hypermutators tended to die off. But when you make the simulated population larger, the hypermutators take over. This pattern matched well with lab experiments evolving yeast. But key questions remained about why hypermutators are rarely found in nature.
“The question that’s posed by [the earlier] work that I think is really interesting is, we have all kinds of large populations in biology where there aren’t hypermutators that have won out,” said Matthew Moreno, a Schmidt AI in Science Fellow at the University of Michigan. “So what’s the missing piece from the puzzle? … There’s a really interesting role for agent based modeling in a broader understanding of evolution, because everything in evolution is driven by so many causal factors that it’s really challenging to take different parts of theory and synthesize them … models and simulations can help to take all these different parts of theory and put them together in a systematic way.”
Moreno wanted to understand the phenomenon of hypermutation, and why mutants don’t always take over in the real world. His work led him to getting a computing allocation from ACCESS, the NSF’s network of supercomputing resources, in which PSC is a leading member. That allocation gave him time on a unique computer: Neocortex, an experimental AI supercomputer funded by NSF at PSC.
Moreno’s work began with PSC’s flagship Bridges-2 system, which he used to compile the files he’d need to carry out his simulations. To run them, though, he’d need a computer optimized for a vast simulation in which individual processors in the computer stood in for subpopulations of an organism.
Neocortex was perfect for the job. Its shared file system with Bridges-2 made transferring to Neocortex relatively easy. But what was even more important to Moreno was the revolutionary architecture of the wafer-scale engines (WSEs) that lie at its core.
The WSE, invented by Cerebras Systems, takes a completely different approach to its hardware compared with traditional computers. Instead of connecting many small computing chips, the WSE incorporates a massive number of computing cores — 850,000 apiece, in the two WSE2s featured in Neocortex — on a single, dinner-plate-sized wafer with multiple connections between the cores. This design speeds artificial intelligence (AI) learning greatly.
In Moreno’s case, it also allowed him to carry out a simulation in which each subpopulation of an artificial species — consisting of simulated agents standing in for individual organisms — “lives” in one core of the WSE. The size of the WSEs also allowed Moreno to vastly increase the simulated population. While the earlier sims had gone up to only 10,000 agents, the WSEs allowed the Michigan scientists to simulate populations as large as 1.5 billion agents. The WSEs also allowed the computations to speed up — 111,000 times faster than a conventional CPU-based computer, and 290 times faster than a graphics processing unit (GPU) computer.
“For these hypermutator experiments, because that population size is a critical parameter, having that scale on the wafer scale engine allowed us to run experiments up to 1.5 billion agents in a population,” Moreno said. “[Another] thing that has been a really important part of this project has been the help that I’ve been able to get … The ByteBoost workshop [hosted at PSC] was an incredible opportunity; going to [the PSC] webinars was really informative about getting the lay of the land. And then the low barrier to entry for trial hardware access to start building and experimenting was a huge onramp.”
As in the earlier simulations, when populations were in the tens of thousands, hypermutators took over in the Neocortex sims. But when the populations expanded even larger, toward real-world sizes, the normally mutating variants regained their advantage and tended to dominate. That is, under one important condition: limited beneficial mutations were available to be discovered. When the team lifted limits on the number of beneficial mutations, the hypermutators regained their advantage.
The team reported related work on porting agent-based evolution simulations to the Wafer-Scale Engine at the 2024 Conference on Artificial Life and the Genetic and Evolutionary Computation Conference, both in July. Analysis of results from their hypermutator experiments is currently ongoing.
Follow-up sims are allowing the team to go deeper. The details of the initial population, including how hypermutators arise and how many of them there are, affects the results. Making the subpopulations more isolated also favored the normal mutators. That’s likely because the hypermutators spread more slowly, giving the normal mutators more time to generate their own beneficial mutations. Another complexity Moreno and his colleagues plan to add to their simulations is a more complex, real-world-like genome, consisting of far more than 32 bits of information.
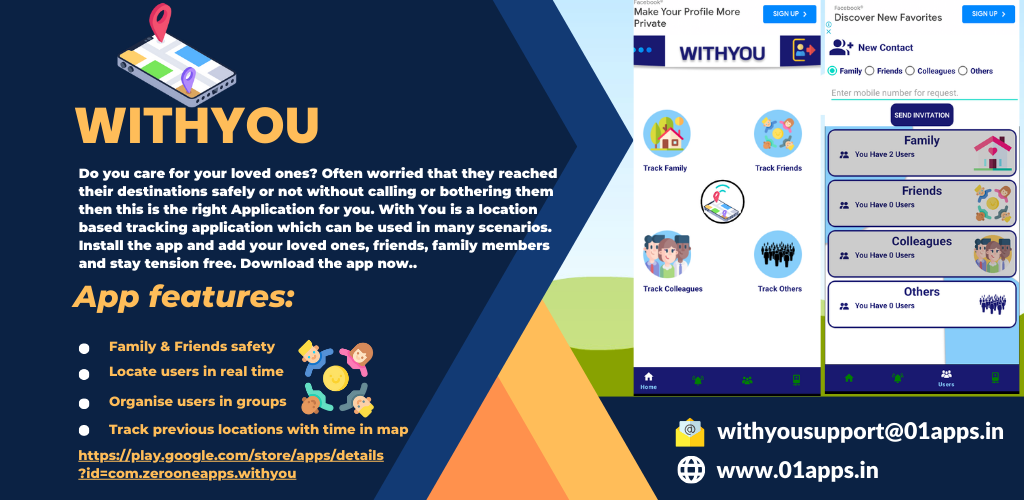