The two-step method, described in the paper, “Fairmandering: A Column Generation Heuristic for Fairness Optimized Political Districting,” first creates billions of potential electoral maps for each state, and then algorithmically identifies a range of possibilities meeting the desired criteria for fairness.
“Fairmandering” won the INFORMS Undergraduate Operations Research Prize at the Nov. 8-11 INFORMS Annual Meeting, the leading meeting of operations research and analytics professionals. First author is Wes Gurnee, now a software engineer at Google.
The American congressional district system empowers politicians to manipulate district boundaries in order to influence election results. Districts may be drawn by the party in power to include large numbers of people in their party, a process known as gerrymandering, swaying the outcome of elections and determining political control at the local and national level.
It’s an urgent issue – especially as states prepare for the decennial redistricting next year, based on the results of the 2020 census.
“Advances in data science have helped the parties get better and better at designing districts to keep political control,” said co-author David Shmoys, professor in the school of operations research and information engineering. “We wanted to offer a completely different perspective that goes to the core of what it means to do a fair districting, and to put algorithmic tools in policymakers’ hands that allow them to do the right thing.”
In the research, the largest-ever study of legal congressional district maps, Gurnee and Shmoys sought to create election maps with fair outcomes – those that accurately reflect a state’s political leanings, create enough competitive races to ensure accountability and treat each party symmetrically.
Once they’ve generated the maps, the researchers used the tools of integer programming – a mathematical modeling framework for which recent advances allowed them to solve a very large-scale problem – to evaluate the maps for fairness.
Though the researchers chose a balanced representation of political affiliation as their definition of fairness in the study, other demographic factors could be considered. The model could also apply to state and local representative maps, in addition to congressional districts.
“It’s not the geographic shape of the district that’s important – it’s really thinking about more holistic principles of what it means to do a fair districting,” Shmoys said. “We’re hoping this will really impact the conversation that’s going to be taking place state by state over the next year and a half, both at the congressional level and the state legislative level.”
For additional information, please see the Cornell Chronicle story.
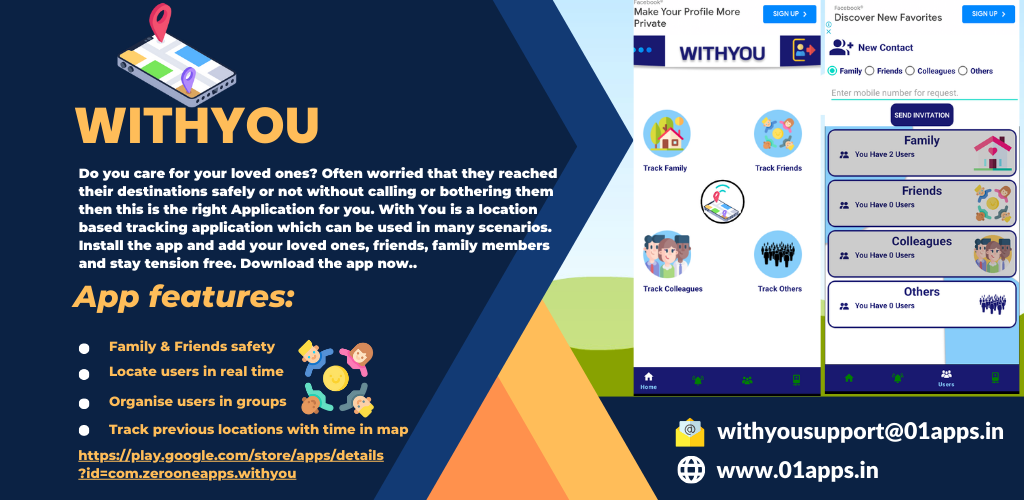