Imagine if you could predict your future movements by attaching a few electromyography (EMG) sensors to your legs. This method of prediction relies on muscle states and is an alternative to the mainstream method that uses multi-view cameras to construct time-series posture. However, there is still a gap between muscle states and predicting future movements.
When muscles move, they push against the ground and create a force called ground reaction force. This force, along with muscle states, produces body movements. To predict future movements based on muscle states, it’s important to estimate ground reaction force, but this is a challenging task due to the complex and time-dependent mapping. To tackle this challenge, a research team from Tohoku University used a long short-term memory network. Their research was published in the journal Cyborg and Bionic Systems on Feb. 21.
Motion prediction plays a crucial role in the animal kingdom, where it is necessary for both hunters and prey to survive. In our daily lives, motion prediction is also important, especially in sports. For instance, when playing basketball, a defender needs to predict whether the offender will try to break through their left or right side, so they can react accordingly and be prepared in advance.
According to Mitsuhiro Hayashibe, the corresponding author of the study and a researcher at the Neuro-Robotics Lab at Tohoku University, motions are generated by forces, which can make it difficult to predict force-driven motion. For example, predicting the initial movement from a static pose without measuring the forces involved is challenging. To build better motion prediction systems, it’s essential to incorporate both kinematics (the study of motion without considering forces) and dynamics (the study of motion and forces) information, especially for predicting actions from static poses.
According to Mitsuhiro Hayashibe, some human motions are not related to previous movements, but only come from muscle contractions. In this case, only using the current and past positions and poses is not enough to predict future motion. For example, when waiting at a traffic light, standing still cannot predict a sudden forward walk. The dynamics of our muscles, especially in the lower limbs, are important to consider in order to make better motion predictions.
In their research, the team conducted two experiments to show that predicting ground reaction force and moment through EMG sensing is possible. In the first experiment, participants repeated a motion that involved swaying their bodies back and forth. The team found that previous time-series postures were important in predicting future movement in this case. In the second experiment, participants were asked to perform a stepping movement, which involved standing and then suddenly taking a step forward. In this case, previous kinematics did not provide much information about future movement.
The authors of the study explained that they used a special type of neural network called a long short-term memory network to predict the future movement of participants in both experiments. They found that this approach was successful in predicting movement in both experiments. However, when compared to a traditional algorithm that only used kinematics information, the new approach performed better in the second experiment where past kinematics provided little information on future movement states.
The authors believe that their method can also be useful for other biomechanical research where the force plate cannot be used to estimate the ground reaction force. They plan to extend their proposed method to integrate with motion performance, motion discrimination information, and time-scale considered motion synergy information for rehabilitation purposes in the future.
The authors of the paper include Sei-Ichi Sakamoto, Yonatan Hutabarat, Dai Owaki, and Mitsuhiro Hayashibe.
The research is funded by the Japan Society for the Promotion of Science Grant-in-Aid for Scientific Research and for Fostering Joint International Research.
The paper, “Ground Reaction Force and Moment Estimation through EMG Sensing Using Long Short-Term Memory Network during Posture Coordination,” was published in the journal Cyborg and Bionic Systems on Feb. 21, 2023, at DOI: https://doi.org/10.34133/cbsystems.0016
Reference
Authors: Sei-ichi Sakamoto1, Yonatan Hutabarat1, Dai Owaki2, and Mitsuhiro Hayashibe1,2
Title of original paper: Ground Reaction Force and Moment Estimation through EMG Sensing Using Long Short-Term Memory Network during Posture Coordination
Journal: Cyborg and Bionic Systems
DOI: 10.34133/cbsystems.0016
Affiliations:
1Neuro-Robotics Lab, Graduate School of Biomedical Engineering, Tohoku University, Sendai, Japan.
2Department of Robotics, Graduate School of Engineering, Tohoku University, Sendai, Japan.
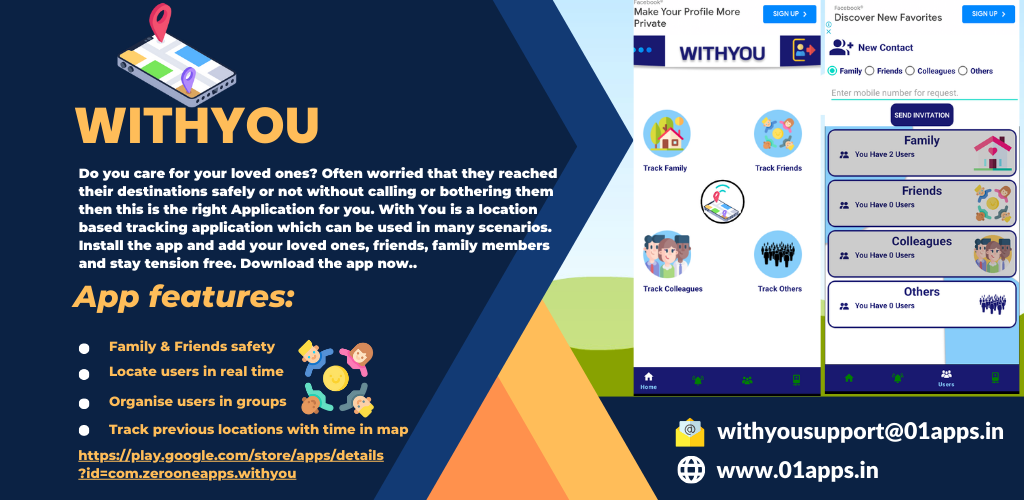