“Just think about what a fly can do,” says Professor Pavan Ramdya, whose lab at EPFL’s Brain Mind Institute, with the lab of Professor Pascal Fua in EPFL’s Institute for Computer Science, led the study. “A fly can climb across terrain that a wheeled robot would not be able to.”
Flies aren’t exactly endearing to humans. We rightly associate them with less-than-appetizing experiences in our daily lives. But there is an unexpected path to redemption: Robots. It turns out that flies have some features and abilities that can inform a new design for robotic systems.
“Unlike most vertebrates, flies can climb nearly any terrain,” says Ramdya. “They can stick to walls and ceilings because they have adhesive pads and claws on the tips of their legs. This allows them to basically go anywhere. That’s interesting also because if you can rest on any surface, you can manage your energy expenditure by waiting for the right moment to act.”
It was this vision of extracting the principles that govern fly behavior to inform the design of robots that drove the development of DeepFly3D, a motion-capture system for the fly Drosophila melanogaster, a model organism that is nearly ubiquitously used across biology.
In Ramdya’s experimental setup, a fly walks on top of a tiny floating ball – like a miniature treadmill – while seven cameras record its every movement. The fly’s top side is glued onto an unmovable stage so that it always stays in place while walking on the ball. Nevertheless, the fly “believes” that it is moving freely.
The collected camera images are then processed by DeepFly3D, a deep-learning software developed by Semih Günel, a PhD student working with both Ramdya’s and Fua’s labs. “This is a fine example of where an interdisciplinary collaboration was necessary and transformative,” says Ramdya. “By leveraging computer science and neuroscience, we’ve tackled a long-standing challenge.”
What’s special about DeepFly3D is that is can infer the 3D pose of the fly – or even other animals – meaning that it can automatically predict and make behavioral measurements at unprecedented resolution for a variety of biological applications. The software doesn’t need to be calibrated manually and it uses camera images to automatically detect and correct any errors it makes in its calculations of the fly’s pose. Finally, it also uses active learning to improve its own performance.
DeepFly3D opens up a way to efficiently and accurately model the movements, poses, and joint angles of a fruit fly in three dimensions. This may inspire a standard way to automatically model 3D pose in other organisms as well.
“The fly, as a model organism, balances tractability and complexity very well,” says Ramdya. “If we learn how it does what it does, we can have important impact on robotics and medicine and, perhaps most importantly, we can gain these insights in a relatively short period of time.”
###
Funding
Microsoft (JRC project)
Swiss National Science Foundation (Eccellenza grant)
EPFL (iPhD grant)
Swiss Government Excellence Postdoctoral Scholarship
Reference
Semih Günel, Helge Rhodin, Daniel Morales, João H. Campagnolo, Pavan Ramdya, Pascal Fua. DeepFly3D, a deep learning-based approach for 3D limb and appendage tracking in tethered, adult Drosophila.
eLife
04 October 2019;8:e48571. DOI: 10.7554/eLife.48571
This part of information is sourced from https://www.eurekalert.org/pub_releases/2019-10/epfd-dtd100919.php
Nik Papageorgiou
41-216-932-105
[email protected]
http://www.epfl.ch/index.en.html
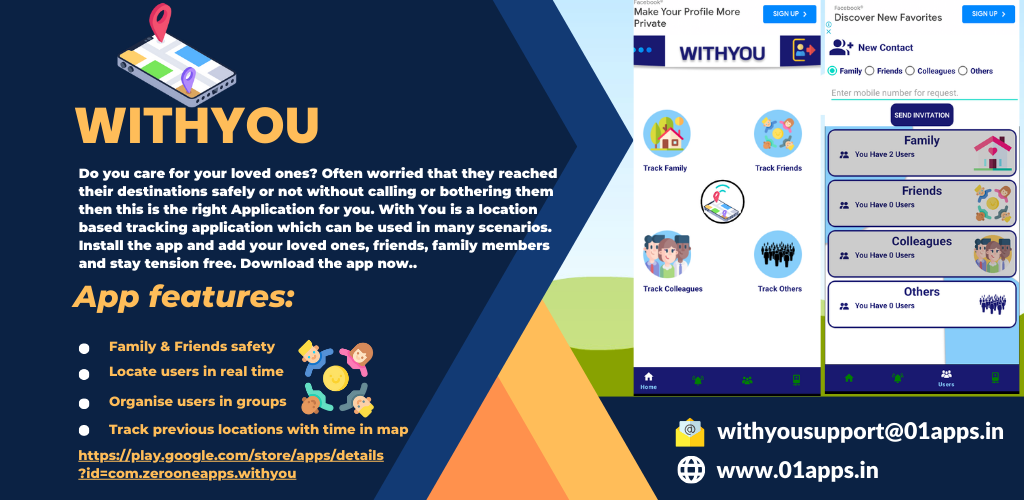