Changes to water masses which are stored on the continents can be detected with the help of satellites. The data sets on the Earth’s gravitational field which are required for this, stem from the GRACE and GRACE-FO satellite missions. As these data sets only include the typical large-scale mass anomalies, no conclusions about small scale structures, such as the actual distribution of water masses in rivers and river branches, are possible. Using the South American continent as an example, the Earth system modellers at the German Research Centre for Geosciences GFZ, have developed a new Deep-Learning-Method, which quantifies small as well as large-scale changes to the water storage with the help of satellite data. This new method cleverly combines Deep-Learning, hydrological models and Earth observations from gravimetry and altimetry.
So far it is not precisely known, how much water a continent really stores. The continental water masses are also constantly changing, thus affecting the Earth’s rotation and acting as a link in the water cycle between atmosphere and ocean. Amazon tributaries in Peru, for example, carry huge amounts of water in some years, but only a fraction of it in others. In addition to the water masses of rivers and other bodies of fresh water, considerable amounts of water are also found in soil, snow and underground reservoirs, which are difficult to quantify directly.
Now the research team around primary author Christopher Irrgang developed a new method in order to draw conclusions on the stored water quantities of the South American continent from the coarsely-resolved satellite data. “For the so called down-scaling, we are using a convolutional neural network, in short CNN, in connection with a newly developed training method”, Irrgang says. “CNNs are particularly well suited for processing spatial Earth observations, because they can reliably extract recurrent patterns such as lines, edges or more complex shapes and characteristics.”
In order to learn the connection between continental water storage and the respective satellite observations, the CNN was trained with simulation data of a numerical hydrological model over the period from 2003 until 2018. Additionally, data from the satellite altimetry in the Amazon region was used for validation. What is extraordinary, is that this CNN continuously self-corrects and self-validates in order to make the most accurate statements possible about the distribution of the water storage. “This CNN therefore combines the advantages of numerical modelling with high-precision Earth observation” according to Irrgang.
The researchers’ study shows that the new Deep-Learning-Method is particularly reliable for the tropical regions north of the -20° latitude on the South American continent, where rain forests, vast surface waters and also large groundwater basins are located. Same as for the groundwater-rich, western part of South America’s southern tip. The down-scaling works less well in dry and desert regions. This can be explained by the comparably low variability of the already low water storage there, which therefore only have a marginal effect on the training of the neural network. However, for the Amazon region, the researchers were able to show that the forecast of the validated CNN was more accurate than the numerical model used.
In future, large-scale as well as regional analysis and forecasts of the global continental water storage will be urgently needed. Further development of numerical models and the combination with innovative Deep-Learning-Methods will take up a more important role in this, in order to gain comprehensive insight into continental hydrology. Aside from purely geophysical investigations, there are many other possible applications, such as studying the impact of climate change on continental hydrology, the identification of stress factors for ecosystems such as droughts or floods, and the development of water management strategies for agricultural and urban regions.
###
Funding:
This study was funded by the Helmholtz-Association as well as The Initiative and Networking Fund of the Helmholtz-Association through the Advanced Earth Modelling Capacity project (ESM).
Original study:
Irrgang, C., Saynisch-Wagner, J., Dill, R., Boergens, E., & Thomas, M. (2020). Self-validating deep learning for recovering terrestrial water storage from gravity and altimetry measurements.
Geophysical Research Letters
, 47, e2020GL089258.
https:/
/
doi.
org/
10.
1029/
2020GL089258
Scientific contact:
Dr. Christopher Irrgang
Scientist in GFZ-section Earth System Modelling
Helmholtz Centre Potsdam
GFZ German Research Centre for Geosciences
Telegrafenberg, 14473 Potsdam
Phone: +49-331-288-2847
e-mail:
[email protected]
Media contact:
Josef Zens
Head of Public and Media Relations
Helmholtz Centre Potsdam
GFZ German Research Centre for Geosciences
Telegrafenberg
14473 Potsdam
Phone: +49 331 288-1040
e-mail:
[email protected]
This part of information is sourced from https://www.eurekalert.org/pub_releases/2020-08/ggph-ail082720.php
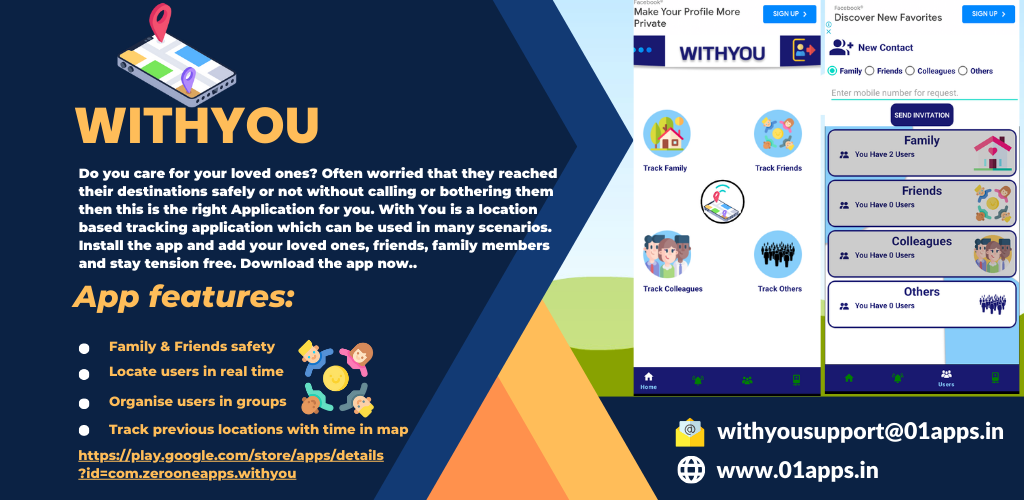