WEST LAFAYETTE, Ind. — To just solve a puzzle or play a game, artificial intelligence can require software running on thousands of computers. That could be the energy that
three nuclear plants
produce in one hour.
A team of engineers has created hardware that can learn skills using a type of AI that currently runs on software platforms. Sharing intelligence features between hardware and software would offset the energy needed for using AI in more advanced applications such as self-driving cars or discovering drugs.
“Software is taking on most of the challenges in AI. If you could incorporate intelligence into the circuit components in addition to what is happening in software, you could do things that simply cannot be done today,” said Shriram Ramanathan, a professor of materials engineering at Purdue University.
AI hardware development is still in early research stages. Researchers have demonstrated AI in pieces of potential hardware, but haven’t yet addressed AI’s large energy demand.
As AI penetrates more of daily life, a heavy reliance on software with massive energy needs is not sustainable, Ramanathan said. If hardware and software could share intelligence features, an area of silicon might be able to achieve more with a given input of energy.
Ramanathan’s team is the first to demonstrate artificial “tree-like” memory in a piece of potential hardware at room temperature. Researchers in the past have only been able to observe this kind of memory in hardware at temperatures that are too low for electronic devices.
The results of this study are published in the journal
Nature Communications
.
The hardware that Ramanathan’s team developed is made of a so-called quantum material. These materials are known for having properties that cannot be explained by classical physics. Ramanathan’s lab has been working to better understand these materials and how they might be used to solve problems in electronics.
Software uses tree-like memory to organize information into various “branches,” making that information easier to retrieve when learning new skills or tasks.
The strategy is inspired by how the human brain categorizes information and makes decisions.
“Humans memorize things in a tree structure of categories. We memorize ‘apple’ under the category of ‘fruit’ and ‘elephant’ under the category of ‘animal,’ for example,” said Hai-Tian Zhang, a Lillian Gilbreth postdoctoral fellow in Purdue’s College of Engineering. “Mimicking these features in hardware is potentially interesting for brain-inspired computing.”
The team introduced a proton to a quantum material called neodymium nickel oxide. They discovered that applying an electric pulse to the material moves around the proton. Each new position of the proton creates a different resistance state, which creates an information storage site called a memory state. Multiple electric pulses create a branch made up of memory states.
“We can build up many thousands of memory states in the material by taking advantage of quantum mechanical effects. The material stays the same. We are simply shuffling around protons,” Ramanathan said.
Through simulations of the properties discovered in this material, the team showed that the material is capable of learning the numbers 0 through 9. The ability to learn numbers is a baseline test of artificial intelligence.
The demonstration of these trees at room temperature in a material is a step toward showing that hardware could offload tasks from software.
“This discovery opens up new frontiers for AI that have been largely ignored because implementing this kind of intelligence into electronic hardware didn’t exist,” Ramanathan said.
The material might also help create a way for humans to more naturally communicate with AI.
“Protons also are natural information transporters in human beings. A device enabled by proton transport may be a key component for eventually achieving direct communication with organisms, such as through a brain implant,” Zhang said.
###
Researchers at the University of California, San Diego, studied the quantum material test strips. The team used synchrotron facilities at the U.S. Department of Energy’s Brookhaven and Argonne National Laboratories to demonstrate that an electric pulse can move protons within neodymium nickel oxide. Other collaborating institutions are the University of Illinois, the University of Louisville and the University of Iowa.
The work was supported by the Lillian Gilbreth Fellowship from Purdue University’s College of Engineering, the Air Force Office of Scientific Research, and the U.S. Department of Energy.
ABSTRACT
Perovskite Neural Trees
Hai-Tian Zhang1,2, Tae Joon Park1, Ivan A. Zaluzhnyy3, Qi Wang1, Shakti Nagnath Wadekar4, Sukriti Manna5,6, Robert Andrawis4, Peter O. Sprau3, Yifei Sun1, Zhen Zhang1, Chengzi Huang1, Hua Zhou7, Zhan Zhang7, Badri Narayanan8, Gopalakrishnan Srinivasan4, Nelson Hua3, Evgeny Nazaretski9, Xiaojing Huang9, Hanfei Yan9, Mingyuan Ge9, Yong S. Chu9, Mathew J. Cherukara5, Martin V. Holt5, Muthu Krishnamurthy10, Oleg Shpyrko3, Subramanian K.R.S. Sankaranarayanan5,6, Alex Frano3, Kaushik Roy4, and Shriram Ramanathan1,
1School of Materials Engineering, Purdue University, West Lafayette, IN 47907, USA
2Lillian Gilbreth Fellowship Program, College of Engineering, Purdue University, West Lafayette, IN 47907, USA
3Department of Physics, University of California, San Diego, La Jolla, CA, 92093, USA
4School of Electrical and Computer Engineering, Purdue University, West Lafayette, IN 47907, USA
5Center for nanoscale materials, Argonne National Laboratory, Argonne, IL 60439, USA
6Department of Mechanical and Industrial Engineering, University of Illinois, Chicago, IL 60607, USA
7X-ray Science Division, Advanced Photon Source, Argonne National Laboratory, Lemont, IL 60439, USA
8Department of Mechanical Engineering, University of Louisville, Louisville, KY 40292, USA
9National Synchrotron Light Source II, Brookhaven National Laboratory, Upton, NY 11973, USA
10Department of Mathematics, University of Iowa, Iowa City, IA 52242, USA
DOI: 10.1038/s41467-020-16105-y
Trees are used by animals, humans and machines to classify information and make decisions. Natural tree structures displayed by synapses of the brain involves potentiation and depression capable of branching and is essential for survival and learning. Demonstration of such features in synthetic matter is challenging due to the need to host a complex energy landscape capable of learning, memory and electrical interrogation. We report experimental realization of tree-like conductance states at room temperature in strongly correlated perovskite nickelates by modulating proton distribution under high speed electric pulses. This demonstration represents physical realization of ultrametric trees, a concept from number theory applied to the study of spin glasses in physics that inspired early neural network theory dating almost forty years ago. We apply the tree-like memory features in spiking neural networks to demonstrate high fidelity object recognition, and in future can open new directions for neuromorphic computing and artificial intelligence.
This part of information is sourced from https://www.eurekalert.org/pub_releases/2020-05/pu-aii050520.php
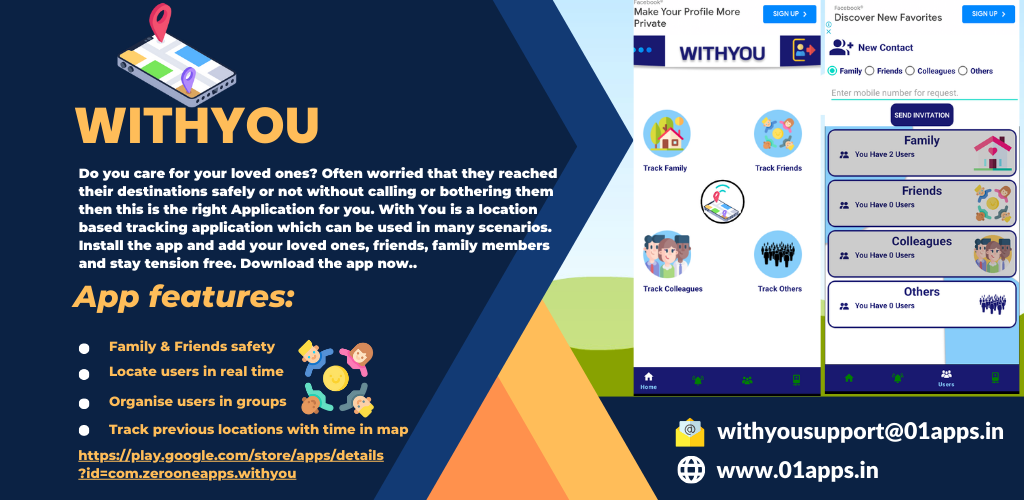