Vanadium flow batteries (VFBs) are promising for stationary large-scale energy storage due to their high safety, long cycle life, and high efficiency.
The cost of a VFB system mainly depends on the VFB stack, electrolyte, and control system. Developing a VFB stack from lab to industrial scale can take years of experiments due to complex factors, from key materials to battery architecture.
Novel methods to accurately predict the performance and cost of a VFB stack and further system are needed in order to accelerate the commercialization of VFBs.
Recently, a research team led by Prof. LI Xianfeng from the Dalian Institute of Chemical Physics (DICP) of the Chinese Academy of Sciences proposed a machine learning-based strategy to predict and optimize the performance and cost of VFBs.
“We use AI technology to improve efficiency, reduce research time, and provide important guidance for the research and development of VFBs” said Prof. LI. “It may accelerate the commercialization of VFBs.”
This work was published in
Energy & Environmental Science
on Sept. 22.
The proposed strategy takes operating current density as the main feature, and the material and structure of the stack as auxiliary features.
This machine learning model can predict the voltage efficiency, energy efficiency, and electrolyte utilization ratio of the VFB stack, as well as the power and energy cost of the VFB system with high accuracy.
In addition, a future R&D direction for the VFB stack was proposed based on model coefficients of machine learning, i.e., developing high-power density VFB stacks under conditions of higher voltage efficiency and higher electrolyte utilization ratio.
This work not only has great significance for the R&D of VFB stacks, but also highlights the prospects for combining machine learning and experiments for optimizing and predicting the dynamic behavior of complex systems.
###
This study was supported by the National Natural Science Foundation of China, the Strategic Priority Research Program of the Chinese Academy of Sciences, the CAS Engineering Laboratory for Electrochemical Energy Storage, and the Liaoning Revitalization Talents Program.
This part of information is sourced from https://www.eurekalert.org/pub_releases/2020-09/caos-atc092820.php
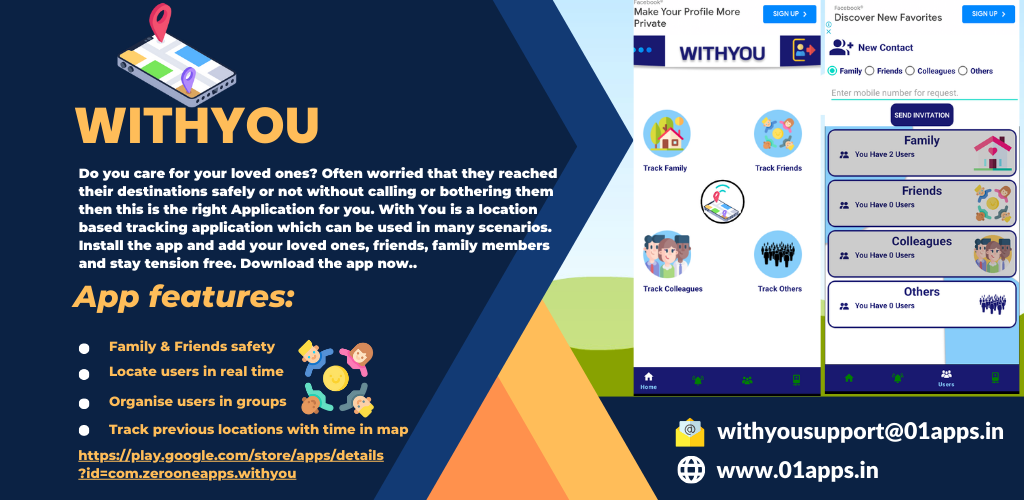