What if, like a murmuration of starlings, our cars and trucks moved cooperatively on the road in response to each vehicle’s environmental sensors, reacting as a group to lessen traffic jams and protect the humans inside?
This question forms the basis of Kuilin Zhang’s National Science Foundation CAREER Award research. Zhang, an associate professor of civil and environmental engineering at Michigan Technological University, has published “A distributionally robust stochastic optimization-based model predictive control with distributionally robust chance constraints for cooperative adaptive cruise control under uncertain traffic conditions” in the journal Transportation Research Part B: Methodological.
The paper is coauthored with Shuaidong Zhao, now a senior quantitative analyst at National Grid, where he continues to conduct research on the interdependency between smart grid and electric vehicle transportation systems.
Creating vehicle systems adept at avoiding traffic accidents is an exercise in proving Newton’s First Law: An object in motion remains so unless acted on by an external force. Without much warning of what’s ahead, car accidents are more likely because drivers don’t have enough time to react. So what stops the car? A collision with another car or obstacle — causing injuries, damage and in the worst case, fatalities.
But cars communicating vehicle-to-vehicle can calculate possible obstacles in the road at increasing distances — and their synchronous reactions can prevent traffic jams and car accidents.
“On the freeway, one bad decision propagates other bad decisions,” Zhang said. “If we can consider what’s happening 300 meters in front of us, it can really improve road safety. It reduces congestion and accidents.”
Zhang’s research asks how vehicles connect to other vehicles, how those vehicles make decisions together based on data from the driving environment and how to integrate disparate observations into a network.
Zhang and Zhao created a data-driven, optimization-based control model for a “platoon” of automated vehicles driving cooperatively under uncertain traffic conditions. Their model, based on the concept of forecasting the forecasts of others, uses streaming data from the modeled vehicles to predict the driving states (accelerating, decelerating or stopped) of preceding platoon vehicles. The predictions are integrated into real-time, machine-learning controllers that provide onboard sensed data. For these automated vehicles, data from controllers across the platoon become resources for cooperative decision-making.
The model indicates controllers could help vehicles maintain constant time gaps between themselves to reduce congestion and traffic accidents and could also conserve energy by reducing the need to accelerate and decelerate.
The next phase of Zhang’s CAREER Award-supported research is to test the model’s simulations using actual connected, autonomous vehicles. Among the locations well-suited to this kind of testing is Michigan Tech’s Keweenaw Research Center, a proving ground for autonomous vehicles, with expertise in unpredictable environments.
Ground truthing the model will enable data-driven, predictive controllers to consider all kinds of hazards vehicles might encounter while driving and create a safer, more certain future for everyone sharing the road.
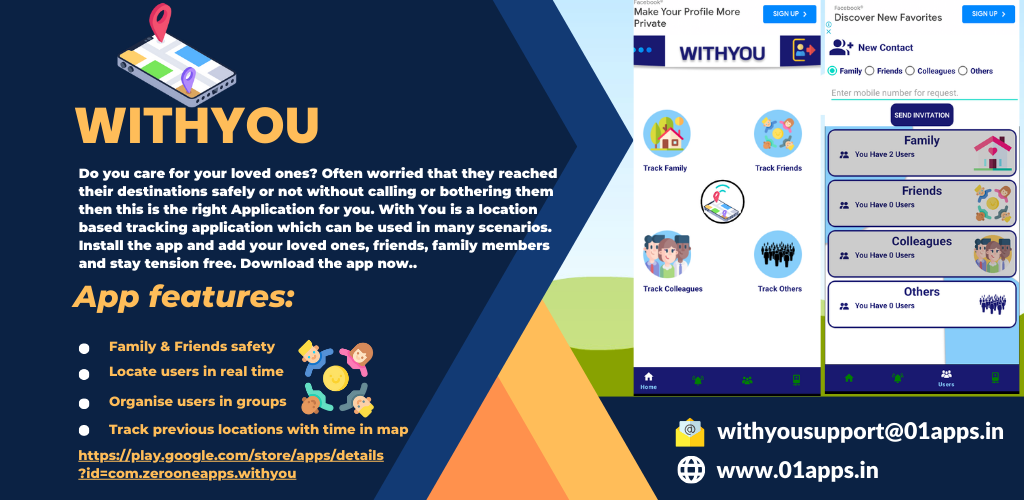