Methods: Two reviewers conducted a systematic search of numerous databases, including PubMed, EMBASE, Cochrane Library, and CINAHL, and then extracted the relevant information from the eligible studies. For this study, we conducted a meta-analysis using a random-effects model. Pooled estimates were calculated for any combination of outcomes and population when at least two studies had relevant data. Study heterogeneity was assessed using I² statistics. The area under the curve (AUC) of the best model and 95% confidence intervals were set as effect measures. Additionally, funnel plots and results from meta-regression analysis were reported. Meta-analysis was performed with R software ver. 4.3.1, and quality evaluation of evidence was conducted according to the GRADE standard.Results:A total of seven studies with 535,418 participants were eligible. A random-effects model showed that models that could predict the risk of developing BM among lung cancer cases had a pooled AUC of 81% (95% CI: 74% to 87%). However, the studies had significantly higher heterogeneity, with an I2 = 99% (p<0.01). Results from meta-regression analysis, accounting for the year of publication (?=-0.013), sample size (?< 0.00), number of variables (?=-0.015), and latitude (?=0.0024) did not show any statistically significant associations with a p>0.05. Egger’s regression test for publication bias did not indicate small study effects (Bias estimate: 4.91, SE = 6.01, p< 0.44).
Conclusions: Our meta-analysis underscores the utility of existing models in predicting the incidence of BM in LC patients, with a pooled AUC of 81%. Further research aimed at refining predictive models and addressing sources of heterogeneity is imperative to enhance clinical decision-making and improve patient outcomes in this population.
Study | AUC [95% CI] | %W |
---|---|---|
Zhao et al. (2023) | 0.7190 [0.5950; 0.9880] | 6.9 |
Li et al. (2022) | 0.7670 [0.7120; 0.8230] | 15.3 |
Zuo et al. [a] (2020) | 0.7310 [0.7260; 0.7360] | 17.1 |
Zuo et al. [b] (2020) | 0.8800 [0.8700; 0.8900] | 17.1 |
Fu et al. (2020) | 0.7240 [0.6800; 0.7500] | 16.4 |
Chong et al. (2021) | 0.9210 [0.8940; 0.9480] | 16.7 |
Cai et al. (2020) | 0.8630 [0.7340; 0.9920] | 10.5 |
Random effects model | 0.8630 [0.7388; 0.8722] | z = 23.67; P< 0.0001; I2= 99.3% |
This material on this page is ©2024 American Society of Clinical Oncology, all rights reserved. Licensing available upon request. For more information, please contact [email protected]
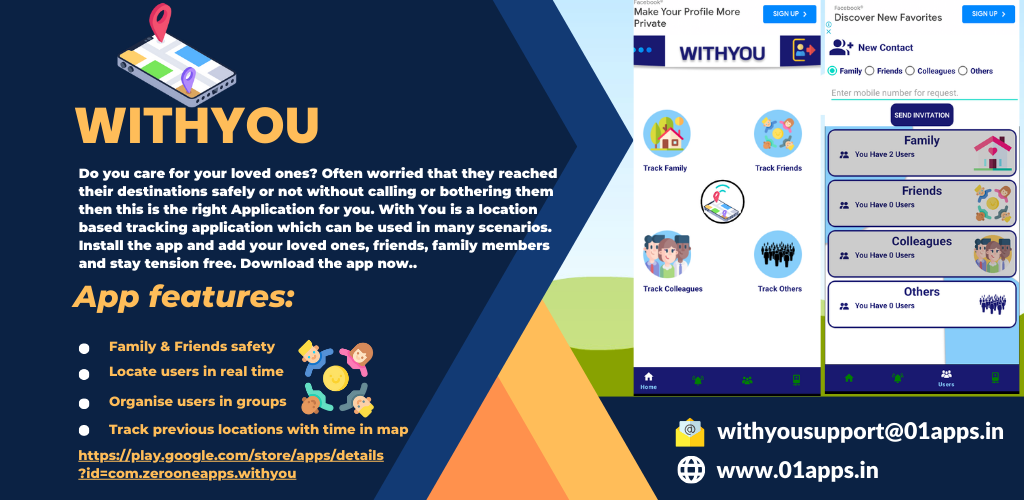