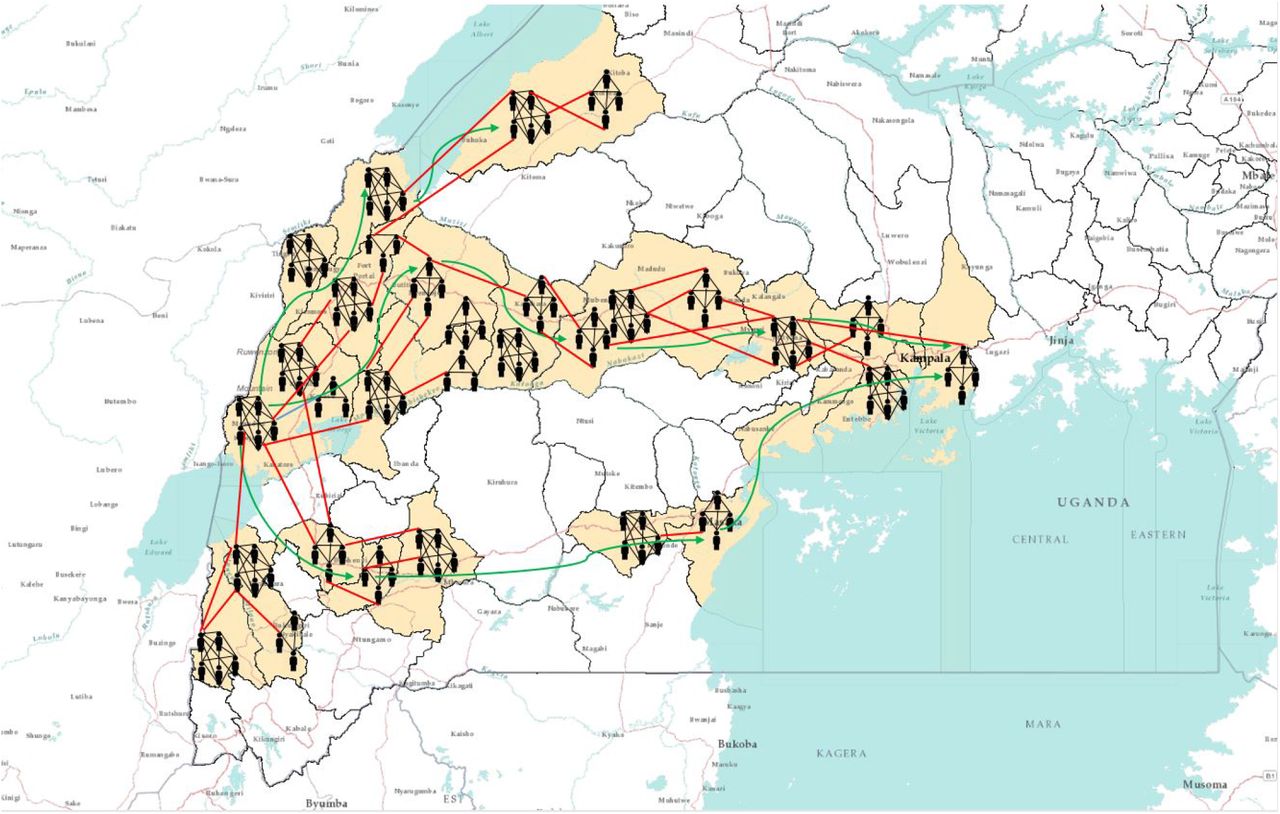
MANHATTAN, KANSAS — A new risk assessment model for the transmission of Ebola accurately predicted its spread into the Republic of Uganda, according to the Kansas State University researchers who developed it.
Caterina Scoglio, professor,
and Mahbubul Riad, doctoral student, both in the Mike Wiegers Department
of Electrical and Computer Engineering in the Carl R. Ice College of
Engineering at Kansas State University; Musa Sekamatte and Issa Makumbi
at Uganda Ministry of Health; and Felix Ocom with the World Health
Organization in Uganda, published “Risk assessment of Ebola virus disease spreading in Uganda using a multilayer temporal network” in bioRxiv on May 23.
The paper describes a new model to better predict how diseases like
Ebola spread. The model combines data of people’s constant contacts —
such as family members and co-workers — with their temporary contacts —
such as people in a market or encountered during travel. According to
Scoglio, the model should be used as a risk assessment tool to prepare
and distribute resources, but it also has been accurate thus far
regarding the movement of Ebola from the Democratic Republic of Congo
into Uganda.
“This is very a new type of model,” Scoglio said.
“Since we consider movement data in addition to constant contacts, we
saw that not only are the districts directly bordering Congo at risk but
that the districts on the path to some important Ugandan destinations
also are at risk.”
In 2018, Scoglio and her collaborators worked
with Ugandan officials to collect movement data to model disease
progression and find areas most at risk. According to the model, the
Kasese district was the highest risk area for an infected person to
enter the country. The researchers used the model to create a 150-day
simulation of possible disease progression in Uganda and produced a map
of 23 Ugandan districts at risk.
The specific scenario used in
the simulation is similar to actual events so far. According to the
Uganda Health Ministry’s June 18 release, there are three confirmed
cases of Ebola in travelers to Uganda — all from one family that entered
the country at the Kasese district border.
“The risk
assessment maps can be used to allocate and distribute limited
resources,” Scoglio said. “Uganda has about 4,000 doses of the new Ebola
vaccine. They are vaccinating health workers, communicating about how
to prevent spreading diseases, and advising people to limit travel in
high-risk areas. We have much respect and admiration for how Uganda has
organized the preparedness and now the response.”
The
researchers used the simulation of Ebola in Uganda to test their model
because there is a lot of traffic coming into the country from the
Democratic Republic of Congo for health care, trading and refuge. Ebola
is highly contagious through physical contact with an infected person
and their bodily fluids.
Scoglio said that even
though the real events in Uganda have aligned with the simulated model,
the scenario should only be used to mitigate the risk.
“One very
important point for the public to understand is the concept of risk and
probability with these maps,” Scoglio said. “It should not be
interpreted that these red regions will be affected because that will
cause panic in the population, but rather these are a guide for
allocation of limited resources in regions that could be potentially
affected if no mitigations are implemented.”
This model may
open a new era in infectious disease management, Scoglio said. She gives
credit to Aram Vajdi, doctoral student in electrical and computer
engineering at Kansas State University, for developing the framework for
the theoretical model based on a multilayer temporal network and the
Gillespie algorithm. Scoglio also praised Riad, who applied the data
collected from Uganda and how Ebola was transmitted to create the risk
assessment.
According to Scoglio, network models used for highly
infectious disease risk assessment must be able to anticipate changes
in human-to-human contacts — unlike many other models, which are based
mainly on constant contacts and constant movement flows. Using these
models can help increase the effectiveness of preventive measures by
targeting the most critical regions and can help decrease the risk of
Ebola and other infectious diseases from spreading.
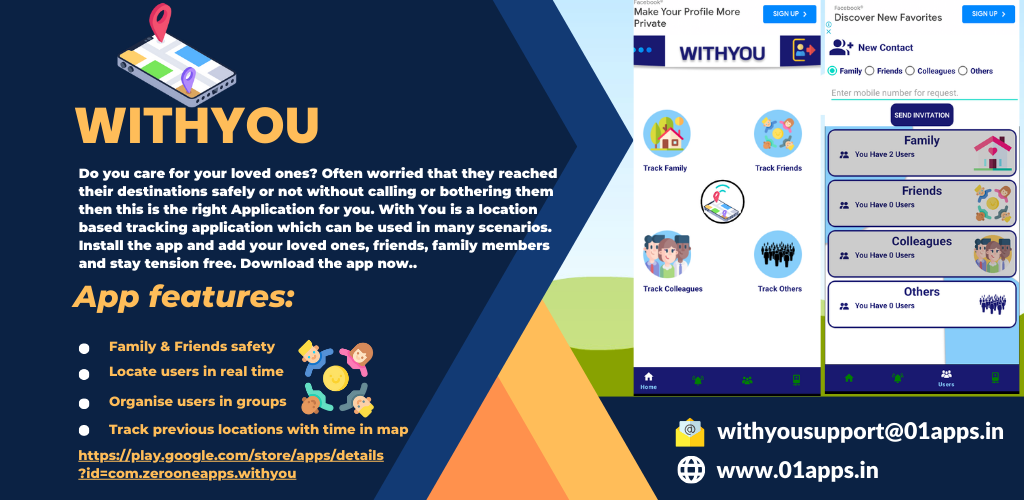