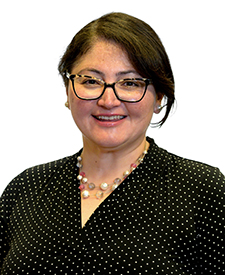
Sarine Markossian, Ph.D., agrees, noting that “an alarmingly high rate of irreproducibility leads to a big gap between preclinical research and the translation of those discoveries to therapies. There are more factors involved in creating this gap, but this is part of the reason,” she says.
Supporting Markossian’s view, a 2016 survey in Nature revealed that more than 70% of researchers have tried and failed to reproduce another scientist’s experiments, and more than half have failed to reproduce their own experiments.
“Most researchers are well-meaning scientists who truly want to make a difference and advance therapeutics for diseases,” continues Markossian, editor-in-chief of the National Institutes of Health’s Assay Guidance Manual (AGM), and lead of the AGM Translational Science Resources Program for NIH’s National Center for Advancing Translational Sciences (NCATS, Bethesda, MD, USA). “It is important for us to provide those scientists the resources and the training to help them understand, first of all, the importance of rigor, and how they can make their assays and experiments more reproducible.”
Robert M. Campbell, Ph.D., editor-in-chief of SLAS Discovery: Advancing the Science of Drug Discovery, comments that reproducibility is rooted in appropriate experimental design, well-described methods, and inclusion of all pertinent details – particularly when scientists enter the publishing process.
“It’s amazing how one small detail can make all the difference between a reproducible experiment and one that is irreproducible,” Campbell says. “Many irreproducible results are unintentional. It’s because the authors did not accurately describe the methods or run appropriate controls, resulting in biased and unbalanced studies,” he says. “As our reviewers read manuscripts, they watch for these issues and another aspect that’s come to the forefront – the potential contamination of cell lines.”
Campbell describes his experiences in big pharma where they sequenced all cell lines and routinely tested for mycoplasma contamination. “In the first analysis of our cell bank, it was quite surprising to see how many cell lines were not what we thought they were, contaminated with other cell lines, or infected with mycoplasma. It’s particularly important to identify the source of the cell lines when publishing. This is an aspect of reproducibility that is fundamental.
For Paige Vinson, Ph.D., director, high-throughput screening (HTS) for Southern Research (Birmingham, AL, USA), and co-chair of the SLAS Screen Design and Assay Technology Topical Interest Group (TIG), “The lack of reproducibility many times stems from the lack of proper protocol and documentation capture,” she says. “Ensuring authentication of experimental reagents, having more than one person in a lab perform experiments to ensure they can be replicated, inclusion of proper controls, non-biased review of data, and publication of detailed protocols is the best path toward resolving the situation.”
Vinson’s co-chair for the SLAS TIG, Kenda LJ Evans, Ph.D., comments that sample preparation is another contributing factor to replication issues. “If multiple people are doing manual research as opposed to using automation, you can typically see much larger variability. Each individual has a different pipetting technique. Once people move to automated sample prep, the reproducibility is almost always improved,” says Evans, an automation workflow specialist for Agilent Technologies (Santa Clara, CA, USA).
Solving the Challenges Around Reproducibility
Markossian states that providing tools for better assessing assay reproducibility, including tools to help research teams analyze the data they produce, is important.
“The AGM program creates best-practice guidelines and shares them with the community to raise awareness,” she notes. “NCATS is also part of a high-throughput screening (HTS) ring testing initiative, where multiple institutions from around the world run the same HTS assay and use the same guidelines,” she continues. NCATS analyzes the data, examines the differences in discovered hits – with particular attention to whether the controls have been run properly – and compares the data across the participating institutions. “The test gives us ideas about where reproducibility problems can come from – such as an instrument that is not calibrated properly,” says Markossian.
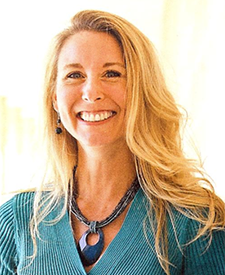
In her work, Evans notices that some research teams, before employing automation such as liquid handlers, accept a larger variability within their samples because it is consistent. “They have reproducibility, it just comes with a larger error amount – a consistent human error,” she comments. “Once these teams use liquid handlers and automation – and commit to conducting sample prep in a more controlled manner – they see more in their results without the noise of variability.”
While automation gets researchers closer to reproducibility, Evans says, some teams opt to not use it in the lab. “If automation is not an option, teams need to ensure that they have excellent quality samples from the start – and that’s always difficult to do. But it’s also just looking at techniques and the assays that they use,” she says. “Some assays are going to give you different kinds of sensitivity and some are more forgiving when things are not quite as controlled as they particularly could be.”
Evans comments that the use of AI and data analysis is more prevalent and positive for reproducibility. “It helps us streamline what worked, what didn’t work and what needs to be changed,” she comments. “In the future, in the best possible way, researchers will use AI to guide what they do next and how to do it better. We are going to become smarter about how we’re designing experiments from the get-go as well as adapting them for improvement.”
As a computational scientist and statistician, Stekhoven observes how biology, life sciences and medicine have become progressively more technical. “Bioinformatics is a direct consequence of this and the increasing amount of data generated, because we suddenly couldn’t handle all the data using dropdown menus and laptop computers anymore,” he comments. “Eventually we needed high-performance computing and version-controlled code.”
He describes how workflow management systems, such as NextFlow, Snakemake and others, “allow users to cast their data-processing pipelines, which may or may not be comprised of different tools that do different steps of the analysis and come from different programming languages, into a contiguous setup. Thus, ensuring that data is always processed in the same way, analyses are traceable, and the results are in theory reproducible.”
For analysis, Stekhoven points to tools like Jupyter or R Markdown notebooks, “which allow your analytic journey to be literate, i.e., the code does not only contain programmer’s comments but also prose on what the intentions of the analyst were to do a certain part of the analysis. The next person who looks at this documentation can understand what your thought process was, and thus making it also in practice reproducible.”
Stekhoven adds that while it does require a certain amount of energy to establish new practices, processes, workflow managers and coding rules, it is well worth it. “By putting additional effort into learning how to code or script, or by introducing certain tools into your daily work and directly from the start of a project, you will get a return on your investment – your research will be more efficient, agile and quicker,” he says. “And above all, you and your peers will be immediately able to build on that research work instead of ‘making it reproducible’ at the end – something that rarely works.”
Campbell comments that the publishing side of the equation is prepared to support researchers employing analytic tools. “Labs generate large data sets rapidly, both by screening and sequencing, and there’s plenty of room for error, because there are many steps involved,” he remarks.
“The SLAS Journals stand ready with qualified reviewers who will examine complex data sets and take the time to understand the appropriateness of the analysis utilized. SLAS Discovery currently has statisticians on the editorial board and as reviewers to help provide critical oversight. We’d certainly like to have more who are strong in informatics, cheminformatics and bioinformatics to make certain research is represented accurately,” Campbell continues. “Case in point; the data could be valid – it just could be interpreted improperly.”
Reproducibility in Life Sciences Journals
Markossian mentions that the rush to publish is another aspect of the reproducibility problem. “Life sciences journals actively recruit novel and interesting results, and researchers are trying to publish their most exciting novel data. While novelty and innovation are important – we all agree on that – I think the value of reproducing your work, focusing on technical details, should also be stated. Repeating the work multiple times is not deemed as interesting or as impactful at the moment,” says Markossian, who also serves as an SLAS Discovery editorial board member.
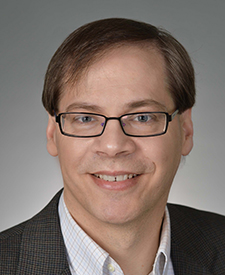
Campbell agrees. “Papers that use some of the data from a previously published article to bridge to new information are extremely helpful. The problem I have with some journals is they will not allow a second paper on the subject, even if only a portion of the manuscript,” he comments.
Campbell finds the quantity of irreproducible research found in high-impact-factor journals frustrating. While he believes that there are excellent papers in life sciences publishing, “I think there remains a significant amount of data published that’s not reproducible,” he comments.
“We need to be able to challenge some of the profession’s prestigious authors, and I’m happy to see people such as Derek Lowe, Ph.D., and organizations such as Bayer HealthCare, trying to reproduce results and publishing the fact that they’re having issues,” Campbell continues. Lowe will be the opening keynote speaker at the SLAS 2024 Data Sciences and AI Symposium to be held November 12-13, 2024, in Cambridge, MA, USA.
Markossian encourages both sides of the publishing equation to pursue long-term over immediate impact. “If you publish something that’s not reproducible, it’s negatively impacting drug discovery. It’s not going to create therapies for any patient,” she says. “Scientists of all experience levels should understand that replicating their work and making sure that the data is reproducible is important because it will make a bigger, long-term impact.”
On a positive note, she comments that many life sciences journals and grant-awarding institutions push for greater transparency on the raw data that is the basis of published manuscripts. “Science journals now are more willing to publish negative as well as positive data,” Markossian continues.
Vinson, who is also an editorial board member for SLAS Discovery, supports journals becoming more rigorous with methods reporting and demonstration of reagent authentication. “This is a move in the right direction,” she says. “I believe it will take significant effort for improvement to occur. Diving into the details of the generated data, and how it is analyzed and interpreted, can often shed light on the differences between two sets of studies.”
Forging a Path Forward
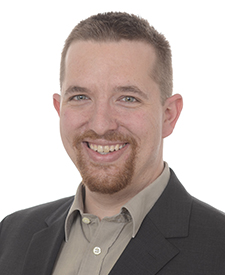
Stekhoven says many reproducibility issues have roots in the attitude and culture of research. “I’m a strong believer that it is somewhat ‘the old ways of doing science’ that hampers progress. Some researchers hold onto the idea that their data is the most important data in the world and cannot under any circumstances be shared,” he comments. “This is a huge problem, because I believe that publicly funded research that generates data should be FAIR – findable, accessible, interoperable, reusable – and ready for ancillary research.”
He is encouraged by early career researchers – as well as undergraduate and graduate students – who are increasingly more computational, and open to collaboration, data sharing and adopting FAIR principles.
“The younger scientists are digital natives,” Stekhoven comments. “They see less of a problem learning how to code or to use version control. They do not shy away when it comes to scripting their science. They’re not afraid to use a cloud tool to support their research. They’re accustomed to touching screens and not touching paper.”
Stekhoven stresses the urgent need to reform science education to further implement reproducibility practices. “Similar to enforcing statistical training for a lot of undergrad or graduate courses, I believe that we should also teach students FAIR data principles and reproducibility, either in a separate mandatory class, or declare mandatory components in courses across all studies,” he says. “I’m speaking not only about the life sciences, but also arts and social sciences. I can’t think of a study direction where it wouldn’t benefit from the inclusion of these elements as these academic paths equally require reproducibility in their research.”
Funders might also play a role in improving rigorous, transparent and reproducible science. Stekhoven points to positive examples of data sharing across country borders and institutions. “I once discussed a project with a colleague in geology whose institute took a research ship to Antarctica to drill ice cores – all at a huge expense,” says Stekhoven. “The moment they had the data, took the pictures, and completed the mass spec analysis on the cores – they immediately published the data. If you don’t do that, you will be banned from further funding. So, not sharing your data has consequences!”
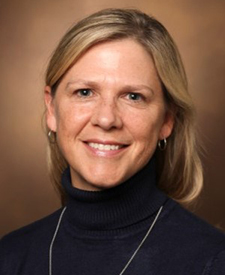
Collaboration is another key component in advancing reproducibility. Vinson comments that although her work with in vitro experiments is more difficult as experimental models become more complex, “I have been a part of collaborative efforts where protocols are ‘harmonized’ between groups and a representative set of experimental results compared across groups,” she explains, adding that this ensures redundancy in experimental capabilities so that projects continue if there is an issue at one site, and safeguards confidence in the validity of results from both sites.
“We all must be open to having methods and results questioned,” Vinson continues. “An open dialog of reasons why a key experiment or research concept cannot be reproduced should be had with a positive intent from all involved in that conversation.”
“The challenges of reproducibility are not going to go away,” Evans comments. “We need it when we’re making go/no-go decisions. If you don’t have good reproducibility, it’s hard to know what’s a true result and what’s a false-positive. I think that talking about reproducibility and finding better ways of achieving it in our assays is crucial to drug discovery and research.”
Markossian believes life sciences is ready for change. She points to the increase in access to the AGM as proof that the community is aware and moving to make improvements. “The monthly access rate of the manual in 2023 varied between 43,000 to 56,000 times per month. That jumped to about 58,000 to 64,000 accessions per month in the first quarter of 2024. My hope is that the AGM program at NCATS will be part of this global change to improve reproducibility that we would like to achieve in the future.”
Campbell, along with the SLAS Journals’ reviewers and editorial boards, feels the weight of ensuring reproducibility standards. The teams – which includes those of SLAS Technology: Translating Life Sciences Innovation – work tirelessly on analyzing submitted manuscripts.
To ensure reproducibility, the SLAS Journals’ author instructions request additional detail for specific statistics on the reproducibility of data they include in their articles, and “we’ve also given them guidance by citing the AGM,” says Campbell, who had a part in creating the early document at Eli Lilly and Co. that eventually developed into the AGM. He notes that the SLAS Journals added a new Protocols article format that allows investigators to go into exquisite detail about the methods.
“The SLAS Journals do their utmost to help the authors improve the quality of their manuscript, such as going through subsequent revisions, and sometimes requesting that the authors conduct additional experiments to make certain that the data is reproducible and with appropriate controls and/or benchmarks, even though it takes more effort and a longer time to complete the review process,” he says.
Campbell would like to see a time when most, if not all, of the published data is reproducible. “It’s sad because in drug discovery we’re trying to help patients who are often left waiting for a new treatment or having to pay more for a drug because of the time and money wasted trying to reproduce other’s results and failing. We can do better,” he comments.
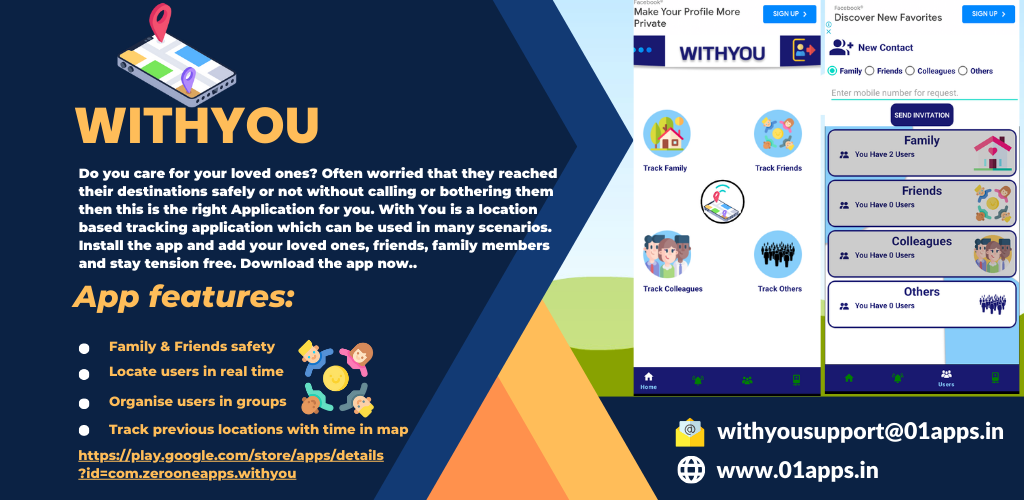