FOR IMMEDIATE RELEASE
Media Contact: Monica McDonald
Office – (404) 633-3777 ext. 332
Axial spondyloarthritis (AxSpA) is a type of spondyloarthritis. This inflammatory form of arthritis usually attacks the spine and pelvis. The main symptom in most patients is low back pain. Many people with axial spondyloarthritis progress to having some degree of spinal fusion, known as ankylosing spondylitis. AxSpA affects both males and females, usually developing before age 30.
If doctors suspect AxSpA, they typically recommend conventional radiography, or X-rays, of the sacroiliac joints. Magnetic resonance imaging (MRI) is also used to assess patients with suspected AxSpA. Researchers select patients for enrollment in clinical trials using classification criteria for their disease. Patients are classified as having either radiographic or non-radiographic AxSpA based on definite signs of the disease on their imaging seen by readers. However, the reliability of radiographic sacroiliitis assessment is known to be poor. Expert readers – as opposed to evaluation by local rheumatologists or radiologists – usually produce more reliable results, but they are not available in many locations.
One proposed solution to this problem is determining if an artificial intelligence (AI)based model can be used to analyze radiographs for improved accuracy of these readings making them comparable to the top experts. This study set out to develop and validate an artificial neural network model that uses AI technology to detect definite radiographic sacroiliitis as a manifestation of AxSpA.
“Conventional X-ray of sacroiliac joints is still the first and sometimes the only available imaging procedure to diagnose AxSpA in many parts of the world,” says study co-author Professor Denis Poddubnyy, Head of the Division of Rheumatology at Charité Universitätsmedizin Berlin in Germany.
“Accurate assessment of radiographic sacroiliitis is relevant for making the correct diagnosis. In the presence of definite radiographic sacroiliitis in a patient with symptoms suggestive of spondyloarthritis, no other imaging procedures are normally needed, and treatment can be started right away. The presence or absence of radiographic sacroiliitis is also relevant for the inclusion in many clinical trials in spondyloarthritis. We see a big discrepancy between the local and central assessment of sacroiliitis reaching sometimes half of the cases.”
For the study, the researchers used conventional radiographs of sacroiliac joints from two independent cohorts of patients with AxSpA, including 1,669 radiographs used to train and validate the neural network, and 100 radiographs used as a test dataset. All the radiographs went through reading by both humans and the artificial neural network. Readers used the modified New York criteria to determine either the presence or absence of definite radiographic sacroiliitis. The researchers then analyzed whether the human readers or artificial neural network agreed.
The artificial neural network achieved excellent performance in accurately recognizing definite radiographic sacroiliitis in these patients, with high ratings of sensitivity and specificity, the study’s findings showed. This artificial intelligence-driven model could enable accurate detection of sacroiliitis, making it relevant for both diagnosis of patients in the clinic and classification of AxSpA when selecting patients for clinical trials.
“I do think that the developed might be helpful in both clinical practice (as a decision-supportive tool) and research (classification of the included patients),” says Dr. Poddubnyy. “However, this is only the first step. The next big challenge is to develop an artificial intelligence-based tool for the assessment of MRI of sacroiliac joints. This would be especially relevant for the diagnosis and differential diagnosis of AxSpA at the early stage.”
###
About ACR Convergence
ACR Convergence, the ACR’s annual meeting, is where rheumatology meets to collaborate, celebrate, congregate, and learn. Join ACR for an all-encompassing experience designed for the entire rheumatology community. ACR Convergence is not just another meeting – it’s where inspiration and opportunity unite to create an unmatched educational experience. For more information about the meeting, visit https://www.rheumatology.org/Annual-Meeting, or join the conversation on Twitter by following the official hashtag (#ACR20).
About the American College of Rheumatology
The American College of Rheumatology (ACR) is an international medical society representing over 7,700 rheumatologists and rheumatology health professionals with a mission to empower rheumatology professionals to excel in their specialty. In doing so, the ACR offers education, research, advocacy and practice management support to help its members continue their innovative work and provide quality patient care. Rheumatologists are experts in the diagnosis, management and treatment of more than 100 different types of arthritis and rheumatic diseases.
ABSTRACT: Development and Validation of an Artificial Intelligence Approach for the Detection of Radiographic Sacroiliitis
Background/Purpose:
Conventional radiography of the sacroiliac joints is still recommended as the first imaging method if axial spondyloarthritis (axSpA) is suspected. Furthermore, radiographic sacroiliitis is included – together with sacroiliitis on magnetic resonance imaging – in the Assessment of Spondyloarthritis International Society (ASAS) classification criteria for axSpA. Depending on the presence or absence of definite radiographic sacroiliitis, axSpA can be classified either as radiographic axSpA (r-axSpA, synonymous to ankylosing spondylitis) or non-radiographic axSpA (nr-axSpA). Although conventional radiography of sacroiliac joints still plays an important role in clinical practice and for clinical trials, the reliability of radiographic sacroiliitis assessment has been reported in a number of studies as mostly poor, even if performed by expert readers. Furthermore, it was shown that untrained local readers perform worse than expert readers specialized in SpA. One possible solution to achieve a comparable high accuracy as an expert on detection of radiographic sacroiliitis, even in non-specialised clinics, could be development of an artificial intelligence-based model for analysis of radiographs.
The aim of this study was to develop and validate an artificial neural network for the detection of definite radiographic sacroiliitis as a manifestation of axial spondyloarthritis.
Methods:
Conventional radiographs of sacroiliac joints from two independent cohorts of patients with axSpA were used. The first cohort (PROOF) consisted of 1669 radiographs and was used for training and validation of a neural network. The second cohort consisted of 100 randomly selected radiographs from GESPIC, which were used as an independent test dataset. In both cohorts all radiographs underwent central reading; the final decision on the presence or absence of definite radiographic sacroiliitis according to the radiographic criterion of the modified New York criteria was used as a reference. For performance evaluation of the neural network, areas under the receiver operating characteristic curves (AUROC) were calculated. Sensitivity and specificity for the prediction cut-offs were calculated. Cohen’s Kappa and the absolute agreement were used to assess the agreement between the neural network and the human readers.
Results:
The neural network achieved an excellent performance in recognition of definite radiographic sacroiliitis with AUROC of 0.97 and 0.96 for the validation and test datasets, respectively (Figure 1). Sensitivity and specificity for the cut-off weighting both measurements equally were 0.90 and 0.93 for the validation and 0.87 and 0.97 for the test set. The Cohen’s kappa between the neural network and the reference judgements were 0.80 for both validation and test sets, and the absolute agreement on the classification yielded 91% and 90%, respectively (Table). Examples of the activation maps of the neural network are presented in Figure 2.
Conclusions: Artificial neural networks enable the accurate detection of definite radiographic sacroiliitis relevant for the diagnosis and classification of axSpA.
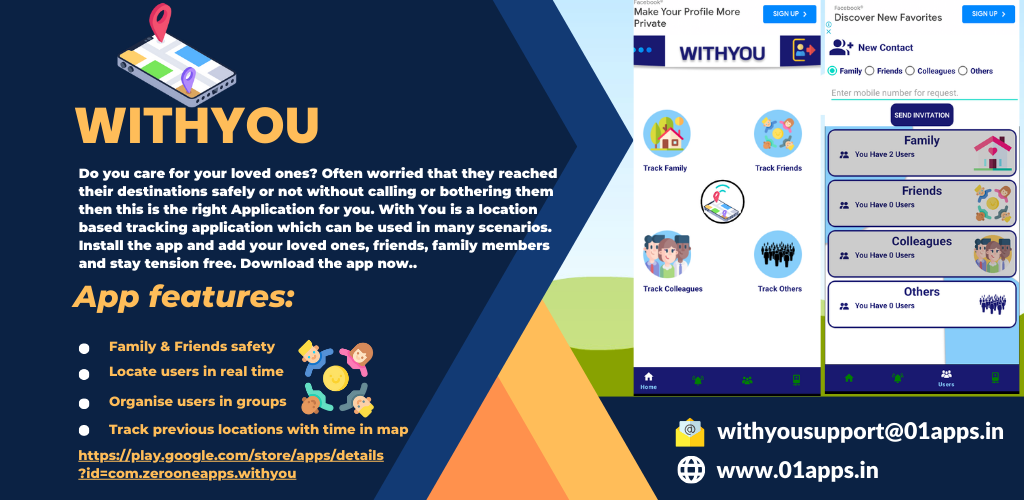