With the phase-out of the fossil economy life became much harder for smart spenders. Now energy prices no longer change annually, but hourly. Solar power is in abundance at lunchtime – in the evening the low sun hardly supplies any energy, while returning commuters are causing the demand for electricity to rise rapidly. The effect can be seen so clearly on consumption graphs that scientists have given it a name: the “duck curve”. When the duck raises its head, it is getting expensive for everyone to buy electricity.
Eyeing the clock when drawing energy will thus be important for electric car drivers and homeowners. In future, those who want to use the available renewable energy in a cheap and at the same time environmentally friendly way will no longer be able to rely on permanently installed thermostats and manually operated buttons.
A multifaceted problem
Bratislav Svetozarevic, a researcher at Empa’s Urban Energy Systems lab, has recognized this problem. What is needed is an automatic control system that hoards energy when it’s abundant and makes it available for expensive times of the day. The battery of one’s car, for instance, which is attached to the charging station in the garage, could serve as a storage device. But Svetozarevic has to deal with a complex problem: Every house is different, and so are its inhabitants. Depending on both weather and season, the power generated by the solar cells changes, as does the need for heating or cooling. An optimized energy control must therefore learn the daily rhythm of a house and its inhabitants – and should also be able to react flexibly during operation, for example when a sudden weather change upsets all calculations.
Step one: the theory
The solution to such problems is AI. The Empa researcher designed an AI control system based on the principle of reinforcement learning. When the system acts “correctly”, it receives a “reward”. Gradually the control system perfects its behavior in this way.
Initially, the control system was only simulated on the computer. The specifications: A certain room in a building had to be electrically heated to the desired temperature and maintain this temperature. At the same time, the system had to supply power to an electric car, which had to be at least 60 percent charged by 7 AM in the morning and be ready to go. In the evening at 5 PM, the electric car returns to the charging station with some residual charge and can supply electricity back to the house during the night. The control system was fed with weather data as well as room temperature data from last year and had to cope with two electricity tariffs: expensive electricity during the day between 8 AM and 8 PM, and cheap electricity during the night.
The result was amazing: The self-learning control system saved around 16 percent energy compared to a fixed-programmed solution and, in the theoretical test, also maintained the desired room temperature much more accurately.
Step two: test in a real building
Now the controller had to pass the reality check. Svetozarevic used the NEST research building on the Empa campus for this purpose. In the DFAB House unit, the AI algorithm controlled the temperature of a student bedroom for a week. At the same time, the 100 kWh-sized storage battery was used to simulate the battery of an electric car. This time, the result was even clearer: During a chilly week in February 2020, the AI control system saved 27 percent of heating energy compared to the neighboring student bedroom, whose heating was operated with a fixed-program (rule-based) control system.
“The nice thing about our self-learning AI control system is that it can be used not only at NEST, but also in any other building”, says Bratislav Svetozarevic. “It doesn’t need an engineer to program the control system, nor someone to analyze the house beforehand and calculate a tailor-made solution.”
Even complex building services can be managed
In a next step, Svetozarevic and his colleagues now want to determine how the system can be extended from one room to larger buildings. “In our first experiment we wanted to map a typical household of the future,” says the Empa researcher. For the sake of simplicity, the team has limited itself to heating and vehicle charging. But the work is laying the foundation for much more: “Our AI control system can still cope when a photovoltaic system supplies electricity, a heat pump and a local hot water storage tank have to be operated – and the comfort requirements of the residents are constantly changing.”
However, a new generation of electric cars is required to be able to use the AI system for optimal energy supply in the future. Today’s standard models in Europe and in the US with the “CCS” quick-charging connection can only be filled up, but not supply electricity. Japanese cars with “Chademo” plugs, on the other hand, are designed for so-called bi-directional charging. The Korean company Hyundai announced in December that it would equip its new electric car platform E-GMP for bidirectional charging, too. This could help homeowners save energy in the long term and at the same time stabilize the electricity grid.
Further information on the topic is available at: www.empa.ch/web/energy-hub
Contact:
Bratislav Svetozarevic
Urban Energy Systems
Phone +41 58 765 6535
[email protected]
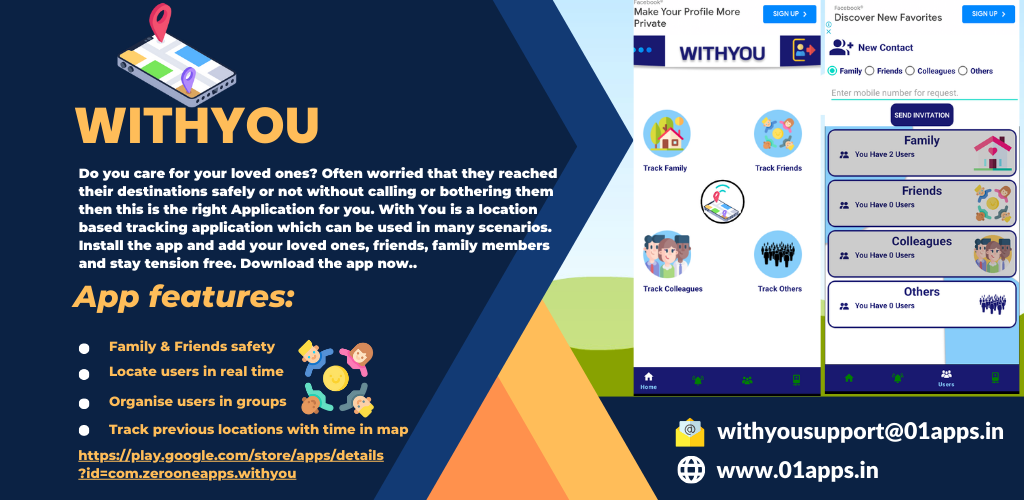