In a major leap forward for environmental science, researchers from Case Western Reserve University and Cornell University have introduced an innovative method for analyzing water alkalinity. Published (DOI: 10.1016/j.eehl.2024.10.002) in the journal Eco-Environment & Health on 14 November 2024, their study reveals a new approach that combines low-cost commercial reagents with machine learning to accurately determine alkalinity levels in water samples—without the need for complex lab equipment.
The researchers’ method uses affordable reagents that change color in response to shifts in alkalinity. These color changes are then captured via smartphone cameras, with images processed by sophisticated machine learning models. The AI algorithms correlate the intensity of the color shift with alkalinity levels, achieving an impressive degree of accuracy—R² values of 0.868 for freshwater and 0.978 for saltwater samples. The technique’s precision is further underscored by its low root-mean-square-error values. With no specialized equipment required, this breakthrough method could revolutionize water quality testing, particularly in regions with limited resources or in situations where traditional equipment is impractical.
Dr. Huichun Zhang, the study’s lead author and a prominent figure in environmental engineering, shared his excitement about the technology’s potential. “This AI-powered approach marks a significant milestone in water quality monitoring. It challenges the trend of ever-more complex and costly analysis techniques, offering a foundation for similar advancements in other water quality parameters,” Zhang said.
The implications of this research are far-reaching. The technique offers an affordable, scalable solution for gathering water quality data, enabling citizen scientists, researchers, and even regulatory agencies to monitor water quality more efficiently. It promises to break down financial barriers, democratizing access to critical environmental data, especially in underserved communities. Moreover, widespread adoption of this technology could contribute to more robust predictive models, enhancing water management practices, agricultural decision-making, and efforts to combat pollution.
###
References
DOI
Original Source URL
https://doi.org/10.1016/j.eehl.2024.10.002
Funding information
This work was funded by the Ohio Department of Higher Education – Harmful Algal Bloom Research Initiative.
About Eco-Environment & Health (EEH)
Eco-Environment & Health (EEH) is an international and multidisciplinary peer-reviewed journal designed for publications on the frontiers of the ecology, environment and health as well as their related disciplines. EEH focuses on the concept of “One Health” to promote green and sustainable development, dealing with the interactions among ecology, environment and health, and the underlying mechanisms and interventions. Our mission is to be one of the most important flagship journals in the field of environmental health.
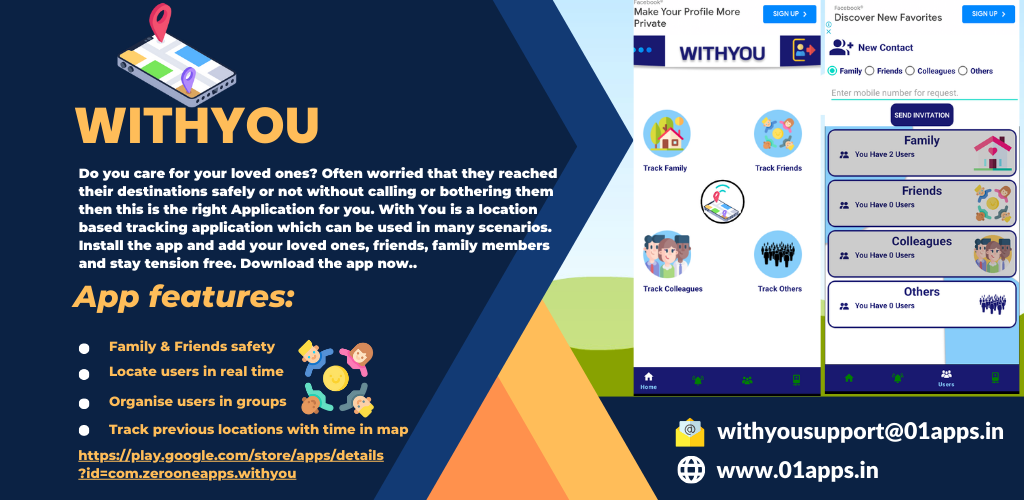