Led by a team of researchers from Hong Kong Polytechnic University, the study (DOI: 10.1186/s43020-024-00136-7) was published in the journal Satellite Navigation on 03 June 2024. The study introduces a novel strategy that uses common geodetic GNSS receivers to identify ionospheric amplitude scintillation events with remarkable precision, potentially transforming GNSS monitoring.
The research focuses on utilizing the vast network of geodetic GNSS receivers to detect ionospheric scintillation events, which are typically identified by specialized ISMRs. The proposed method employs a pre-trained machine learning decision tree algorithm that processes the carrier-to-noise ratio (C/N0) and elevation angle data collected at 1-Hz intervals. By mitigating multipath effects through detailed analysis of multipath patterns, the study effectively reduces noise and false alarms, ensuring the accuracy of the scintillation detection. The methodology involves computing an alternative scintillation index (S4c) based on C/N0 measurements from geodetic GNSS receivers. This index shows a high correlation with the traditional S4 index used by ISMRs, despite the higher susceptibility of geodetic receivers to noise and multipath interference. The machine learning algorithm enhances detection accuracy by leveraging the periodic nature of multipath effects, which differ from the irregularities of scintillation. Experimental results demonstrate that the decision tree algorithm achieves a remarkable 99.9% detection accuracy, surpassing traditional hard and semi-hard threshold methods.
Dr. Yiping Jiang, the lead researcher, stated, “Our study showcases the potential of integrating machine learning with widely available GNSS receivers to revolutionize ionospheric scintillation detection. This method not only provides a cost-effective alternative to specialized equipment but also enhances the accuracy and reliability of space weather monitoring.”
The implications of this research are far-reaching, offering a scalable solution for GNSS users worldwide. By improving the detection of scintillation events, it contributes to the development of more accurate navigation algorithms and techniques. This advancement is crucial for various applications, including aviation, maritime, and land transportation, where GNSS reliability is paramount.
###
References
DOI
Original Source URL
https://doi.org/10.1186/s43020-024-00136-7
Funding information
The work described in this paper was supported by grants from the Research Grants Council of the Hong Kong Special Administrative Region, China (Project No. 25202520; 15214523) and the National Natural Science Foundation of China (Grant No. 42004029).
About Satellite Navigation
Satellite Navigation (E-ISSN: 2662-1363; ISSN: 2662-9291) is the official journal of Aerospace Information Research Institute, Chinese Academy of Sciences. The journal aims to report innovative ideas, new results or progress on the theoretical techniques and applications of satellite navigation. The journal welcomes original articles, reviews and commentaries.
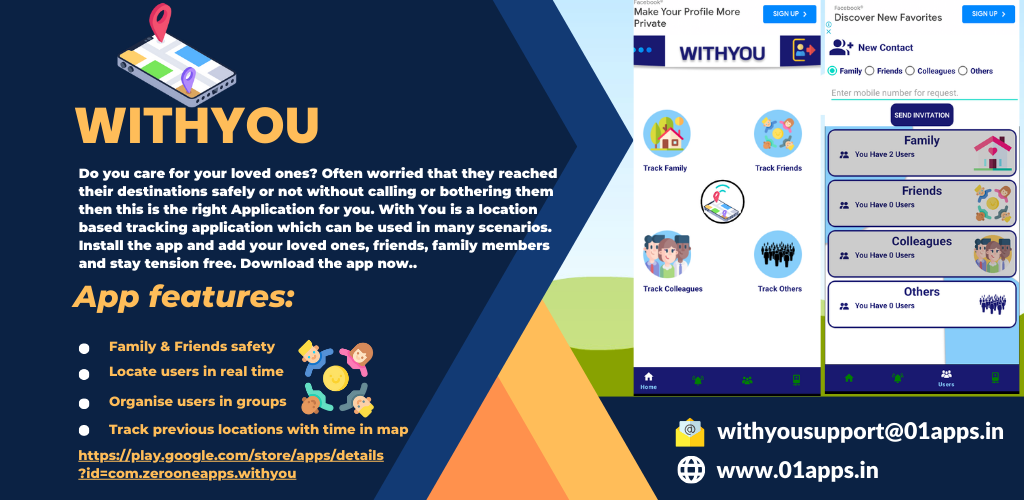